How to do weighted sum as tensor operation in tensorflow?
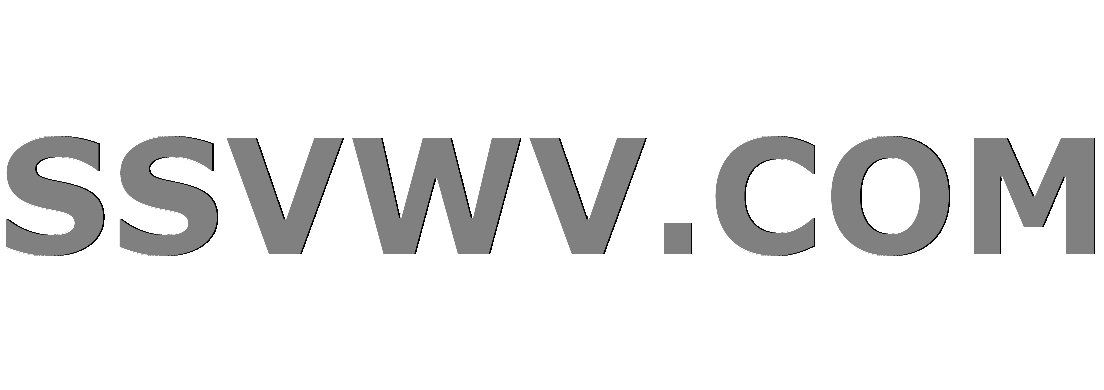
Multi tool use
I am trying to do a weighted sum of matrices in tensorflow.
Unfortunately, my dimensions are not small and I have a problem with memory. Another option is that I doing something completely wrong
I have two tensors U with shape (B,F,M) and A with shape (C,B). I would like to do weighted sum and stacking.
Weighted sum
For each index c from C, I have vector of weights a from A, with shape (B,).
I want to use it for the weighted sum of U to get matrix U_t with shape (F, M). This is pretty same with this, where I found small help.
Concatenation
Unfortunately, I want to do this for each vector a in A to get C matrices U_tc in list. U_tc have mentioned shape (F,M). After that I concatenate all matrices in list to get super matrix with shape (C*F,M)
My values are C=2500, M=500, F=80, B=300
In the beginning, I tried the very naive approach with many loop and element selection which generate very much operation.
Now with help from this, I have following:
U = tf.Variable(tf.truncated_normal([B, F, M],stddev=1.0 ,dtype=tf.float32) #just for example
A = tf.Variable(tf.truncated_normal([C, B],stddev=1.0) ,dtype=tf.float32) #just for example
U_t =
for ccc in xrange(C):
a = A[ccc,:]
a_broadcasted = tf.tile(tf.reshape(a,[B,1,1]), tf.stack([1,F,M]))
T_p.append(tf.reduce_sum(tf.multiply(U,a_broadcasted), axis=0))
U_tcs = tf.concat(U_t,axis=0)
Unfortunately, this is failing at memory error. I am not sure if I did something wrong, or it is because computation has a too much mathematic operation? Because I think... variables aren't too large for memory, right? At least, I had larger variables before and it was ok. (I have 16 GB GPU memory)
Am I doing that weighted sum correctly?
Any idea how to do it more effective?
I will appreciate any help. Thanks.
python-2.7 tensorflow matrix matrix-multiplication weighted
add a comment |
I am trying to do a weighted sum of matrices in tensorflow.
Unfortunately, my dimensions are not small and I have a problem with memory. Another option is that I doing something completely wrong
I have two tensors U with shape (B,F,M) and A with shape (C,B). I would like to do weighted sum and stacking.
Weighted sum
For each index c from C, I have vector of weights a from A, with shape (B,).
I want to use it for the weighted sum of U to get matrix U_t with shape (F, M). This is pretty same with this, where I found small help.
Concatenation
Unfortunately, I want to do this for each vector a in A to get C matrices U_tc in list. U_tc have mentioned shape (F,M). After that I concatenate all matrices in list to get super matrix with shape (C*F,M)
My values are C=2500, M=500, F=80, B=300
In the beginning, I tried the very naive approach with many loop and element selection which generate very much operation.
Now with help from this, I have following:
U = tf.Variable(tf.truncated_normal([B, F, M],stddev=1.0 ,dtype=tf.float32) #just for example
A = tf.Variable(tf.truncated_normal([C, B],stddev=1.0) ,dtype=tf.float32) #just for example
U_t =
for ccc in xrange(C):
a = A[ccc,:]
a_broadcasted = tf.tile(tf.reshape(a,[B,1,1]), tf.stack([1,F,M]))
T_p.append(tf.reduce_sum(tf.multiply(U,a_broadcasted), axis=0))
U_tcs = tf.concat(U_t,axis=0)
Unfortunately, this is failing at memory error. I am not sure if I did something wrong, or it is because computation has a too much mathematic operation? Because I think... variables aren't too large for memory, right? At least, I had larger variables before and it was ok. (I have 16 GB GPU memory)
Am I doing that weighted sum correctly?
Any idea how to do it more effective?
I will appreciate any help. Thanks.
python-2.7 tensorflow matrix matrix-multiplication weighted
Question is difficult to understanding with matrix dimensions alone. Probably asking using a picture or explaining the application use-case (like trying to something with image, etc) might help
– solver149
Dec 31 '18 at 21:03
add a comment |
I am trying to do a weighted sum of matrices in tensorflow.
Unfortunately, my dimensions are not small and I have a problem with memory. Another option is that I doing something completely wrong
I have two tensors U with shape (B,F,M) and A with shape (C,B). I would like to do weighted sum and stacking.
Weighted sum
For each index c from C, I have vector of weights a from A, with shape (B,).
I want to use it for the weighted sum of U to get matrix U_t with shape (F, M). This is pretty same with this, where I found small help.
Concatenation
Unfortunately, I want to do this for each vector a in A to get C matrices U_tc in list. U_tc have mentioned shape (F,M). After that I concatenate all matrices in list to get super matrix with shape (C*F,M)
My values are C=2500, M=500, F=80, B=300
In the beginning, I tried the very naive approach with many loop and element selection which generate very much operation.
Now with help from this, I have following:
U = tf.Variable(tf.truncated_normal([B, F, M],stddev=1.0 ,dtype=tf.float32) #just for example
A = tf.Variable(tf.truncated_normal([C, B],stddev=1.0) ,dtype=tf.float32) #just for example
U_t =
for ccc in xrange(C):
a = A[ccc,:]
a_broadcasted = tf.tile(tf.reshape(a,[B,1,1]), tf.stack([1,F,M]))
T_p.append(tf.reduce_sum(tf.multiply(U,a_broadcasted), axis=0))
U_tcs = tf.concat(U_t,axis=0)
Unfortunately, this is failing at memory error. I am not sure if I did something wrong, or it is because computation has a too much mathematic operation? Because I think... variables aren't too large for memory, right? At least, I had larger variables before and it was ok. (I have 16 GB GPU memory)
Am I doing that weighted sum correctly?
Any idea how to do it more effective?
I will appreciate any help. Thanks.
python-2.7 tensorflow matrix matrix-multiplication weighted
I am trying to do a weighted sum of matrices in tensorflow.
Unfortunately, my dimensions are not small and I have a problem with memory. Another option is that I doing something completely wrong
I have two tensors U with shape (B,F,M) and A with shape (C,B). I would like to do weighted sum and stacking.
Weighted sum
For each index c from C, I have vector of weights a from A, with shape (B,).
I want to use it for the weighted sum of U to get matrix U_t with shape (F, M). This is pretty same with this, where I found small help.
Concatenation
Unfortunately, I want to do this for each vector a in A to get C matrices U_tc in list. U_tc have mentioned shape (F,M). After that I concatenate all matrices in list to get super matrix with shape (C*F,M)
My values are C=2500, M=500, F=80, B=300
In the beginning, I tried the very naive approach with many loop and element selection which generate very much operation.
Now with help from this, I have following:
U = tf.Variable(tf.truncated_normal([B, F, M],stddev=1.0 ,dtype=tf.float32) #just for example
A = tf.Variable(tf.truncated_normal([C, B],stddev=1.0) ,dtype=tf.float32) #just for example
U_t =
for ccc in xrange(C):
a = A[ccc,:]
a_broadcasted = tf.tile(tf.reshape(a,[B,1,1]), tf.stack([1,F,M]))
T_p.append(tf.reduce_sum(tf.multiply(U,a_broadcasted), axis=0))
U_tcs = tf.concat(U_t,axis=0)
Unfortunately, this is failing at memory error. I am not sure if I did something wrong, or it is because computation has a too much mathematic operation? Because I think... variables aren't too large for memory, right? At least, I had larger variables before and it was ok. (I have 16 GB GPU memory)
Am I doing that weighted sum correctly?
Any idea how to do it more effective?
I will appreciate any help. Thanks.
python-2.7 tensorflow matrix matrix-multiplication weighted
python-2.7 tensorflow matrix matrix-multiplication weighted
edited Dec 31 '18 at 20:27
OndraN
asked Dec 31 '18 at 19:03
OndraNOndraN
74
74
Question is difficult to understanding with matrix dimensions alone. Probably asking using a picture or explaining the application use-case (like trying to something with image, etc) might help
– solver149
Dec 31 '18 at 21:03
add a comment |
Question is difficult to understanding with matrix dimensions alone. Probably asking using a picture or explaining the application use-case (like trying to something with image, etc) might help
– solver149
Dec 31 '18 at 21:03
Question is difficult to understanding with matrix dimensions alone. Probably asking using a picture or explaining the application use-case (like trying to something with image, etc) might help
– solver149
Dec 31 '18 at 21:03
Question is difficult to understanding with matrix dimensions alone. Probably asking using a picture or explaining the application use-case (like trying to something with image, etc) might help
– solver149
Dec 31 '18 at 21:03
add a comment |
1 Answer
1
active
oldest
votes
1. Weighted sum and Concatenation
You can use vector operations directly without loops when memory is not limited.
import tensorflow as tf
C,M,F,B=2500,500,80,300
U = tf.Variable(tf.truncated_normal([B, F, M],stddev=1.0 ,dtype=tf.float32)) #just for example
A = tf.Variable(tf.truncated_normal([C, B],stddev=1.0) ,dtype=tf.float32) #just for example
# shape=(C,B,1,1)
A_new = tf.expand_dims(tf.expand_dims(A,-1),-1)
# shape=(B,F,M)
U_t = tf.reduce_sum(tf.multiply(A_new , U),axis=1)
# shape=(C*F,M)
U_tcs = tf.reshape(U_t,(C*F,M))
2. Memory error
In fact, I also had memory errors when I ran the above code.
ResourceExhaustedError (see above for traceback): OOM when allocating tensor with shape[2500,300,80,500]...
With a little modification of the above code, it works properly on my 8GB GPU memory.
import tensorflow as tf
C,M,F,B=2500,500,80,300
U = tf.Variable(tf.truncated_normal([B, F, M],stddev=1.0 ,dtype=tf.float32)) #just for example
A = tf.Variable(tf.truncated_normal([C, B],stddev=1.0) ,dtype=tf.float32) #just for example
# shape=(C,B,1,1)
A_new = tf.expand_dims(tf.expand_dims(A,-1),-1)
U_t =
for ccc in range(C):
a = A_new[ccc,:]
a_broadcasted = tf.reduce_sum(tf.multiply(a, U),axis=0)
U_t.append(a_broadcasted)
U_tcs = tf.concat(U_t,axis=0)
thanks ;) it really helped.
– OndraN
Jan 1 at 16:30
add a comment |
Your Answer
StackExchange.ifUsing("editor", function () {
StackExchange.using("externalEditor", function () {
StackExchange.using("snippets", function () {
StackExchange.snippets.init();
});
});
}, "code-snippets");
StackExchange.ready(function() {
var channelOptions = {
tags: "".split(" "),
id: "1"
};
initTagRenderer("".split(" "), "".split(" "), channelOptions);
StackExchange.using("externalEditor", function() {
// Have to fire editor after snippets, if snippets enabled
if (StackExchange.settings.snippets.snippetsEnabled) {
StackExchange.using("snippets", function() {
createEditor();
});
}
else {
createEditor();
}
});
function createEditor() {
StackExchange.prepareEditor({
heartbeatType: 'answer',
autoActivateHeartbeat: false,
convertImagesToLinks: true,
noModals: true,
showLowRepImageUploadWarning: true,
reputationToPostImages: 10,
bindNavPrevention: true,
postfix: "",
imageUploader: {
brandingHtml: "Powered by u003ca class="icon-imgur-white" href="https://imgur.com/"u003eu003c/au003e",
contentPolicyHtml: "User contributions licensed under u003ca href="https://creativecommons.org/licenses/by-sa/3.0/"u003ecc by-sa 3.0 with attribution requiredu003c/au003e u003ca href="https://stackoverflow.com/legal/content-policy"u003e(content policy)u003c/au003e",
allowUrls: true
},
onDemand: true,
discardSelector: ".discard-answer"
,immediatelyShowMarkdownHelp:true
});
}
});
Sign up or log in
StackExchange.ready(function () {
StackExchange.helpers.onClickDraftSave('#login-link');
});
Sign up using Google
Sign up using Facebook
Sign up using Email and Password
Post as a guest
Required, but never shown
StackExchange.ready(
function () {
StackExchange.openid.initPostLogin('.new-post-login', 'https%3a%2f%2fstackoverflow.com%2fquestions%2f53990649%2fhow-to-do-weighted-sum-as-tensor-operation-in-tensorflow%23new-answer', 'question_page');
}
);
Post as a guest
Required, but never shown
1 Answer
1
active
oldest
votes
1 Answer
1
active
oldest
votes
active
oldest
votes
active
oldest
votes
1. Weighted sum and Concatenation
You can use vector operations directly without loops when memory is not limited.
import tensorflow as tf
C,M,F,B=2500,500,80,300
U = tf.Variable(tf.truncated_normal([B, F, M],stddev=1.0 ,dtype=tf.float32)) #just for example
A = tf.Variable(tf.truncated_normal([C, B],stddev=1.0) ,dtype=tf.float32) #just for example
# shape=(C,B,1,1)
A_new = tf.expand_dims(tf.expand_dims(A,-1),-1)
# shape=(B,F,M)
U_t = tf.reduce_sum(tf.multiply(A_new , U),axis=1)
# shape=(C*F,M)
U_tcs = tf.reshape(U_t,(C*F,M))
2. Memory error
In fact, I also had memory errors when I ran the above code.
ResourceExhaustedError (see above for traceback): OOM when allocating tensor with shape[2500,300,80,500]...
With a little modification of the above code, it works properly on my 8GB GPU memory.
import tensorflow as tf
C,M,F,B=2500,500,80,300
U = tf.Variable(tf.truncated_normal([B, F, M],stddev=1.0 ,dtype=tf.float32)) #just for example
A = tf.Variable(tf.truncated_normal([C, B],stddev=1.0) ,dtype=tf.float32) #just for example
# shape=(C,B,1,1)
A_new = tf.expand_dims(tf.expand_dims(A,-1),-1)
U_t =
for ccc in range(C):
a = A_new[ccc,:]
a_broadcasted = tf.reduce_sum(tf.multiply(a, U),axis=0)
U_t.append(a_broadcasted)
U_tcs = tf.concat(U_t,axis=0)
thanks ;) it really helped.
– OndraN
Jan 1 at 16:30
add a comment |
1. Weighted sum and Concatenation
You can use vector operations directly without loops when memory is not limited.
import tensorflow as tf
C,M,F,B=2500,500,80,300
U = tf.Variable(tf.truncated_normal([B, F, M],stddev=1.0 ,dtype=tf.float32)) #just for example
A = tf.Variable(tf.truncated_normal([C, B],stddev=1.0) ,dtype=tf.float32) #just for example
# shape=(C,B,1,1)
A_new = tf.expand_dims(tf.expand_dims(A,-1),-1)
# shape=(B,F,M)
U_t = tf.reduce_sum(tf.multiply(A_new , U),axis=1)
# shape=(C*F,M)
U_tcs = tf.reshape(U_t,(C*F,M))
2. Memory error
In fact, I also had memory errors when I ran the above code.
ResourceExhaustedError (see above for traceback): OOM when allocating tensor with shape[2500,300,80,500]...
With a little modification of the above code, it works properly on my 8GB GPU memory.
import tensorflow as tf
C,M,F,B=2500,500,80,300
U = tf.Variable(tf.truncated_normal([B, F, M],stddev=1.0 ,dtype=tf.float32)) #just for example
A = tf.Variable(tf.truncated_normal([C, B],stddev=1.0) ,dtype=tf.float32) #just for example
# shape=(C,B,1,1)
A_new = tf.expand_dims(tf.expand_dims(A,-1),-1)
U_t =
for ccc in range(C):
a = A_new[ccc,:]
a_broadcasted = tf.reduce_sum(tf.multiply(a, U),axis=0)
U_t.append(a_broadcasted)
U_tcs = tf.concat(U_t,axis=0)
thanks ;) it really helped.
– OndraN
Jan 1 at 16:30
add a comment |
1. Weighted sum and Concatenation
You can use vector operations directly without loops when memory is not limited.
import tensorflow as tf
C,M,F,B=2500,500,80,300
U = tf.Variable(tf.truncated_normal([B, F, M],stddev=1.0 ,dtype=tf.float32)) #just for example
A = tf.Variable(tf.truncated_normal([C, B],stddev=1.0) ,dtype=tf.float32) #just for example
# shape=(C,B,1,1)
A_new = tf.expand_dims(tf.expand_dims(A,-1),-1)
# shape=(B,F,M)
U_t = tf.reduce_sum(tf.multiply(A_new , U),axis=1)
# shape=(C*F,M)
U_tcs = tf.reshape(U_t,(C*F,M))
2. Memory error
In fact, I also had memory errors when I ran the above code.
ResourceExhaustedError (see above for traceback): OOM when allocating tensor with shape[2500,300,80,500]...
With a little modification of the above code, it works properly on my 8GB GPU memory.
import tensorflow as tf
C,M,F,B=2500,500,80,300
U = tf.Variable(tf.truncated_normal([B, F, M],stddev=1.0 ,dtype=tf.float32)) #just for example
A = tf.Variable(tf.truncated_normal([C, B],stddev=1.0) ,dtype=tf.float32) #just for example
# shape=(C,B,1,1)
A_new = tf.expand_dims(tf.expand_dims(A,-1),-1)
U_t =
for ccc in range(C):
a = A_new[ccc,:]
a_broadcasted = tf.reduce_sum(tf.multiply(a, U),axis=0)
U_t.append(a_broadcasted)
U_tcs = tf.concat(U_t,axis=0)
1. Weighted sum and Concatenation
You can use vector operations directly without loops when memory is not limited.
import tensorflow as tf
C,M,F,B=2500,500,80,300
U = tf.Variable(tf.truncated_normal([B, F, M],stddev=1.0 ,dtype=tf.float32)) #just for example
A = tf.Variable(tf.truncated_normal([C, B],stddev=1.0) ,dtype=tf.float32) #just for example
# shape=(C,B,1,1)
A_new = tf.expand_dims(tf.expand_dims(A,-1),-1)
# shape=(B,F,M)
U_t = tf.reduce_sum(tf.multiply(A_new , U),axis=1)
# shape=(C*F,M)
U_tcs = tf.reshape(U_t,(C*F,M))
2. Memory error
In fact, I also had memory errors when I ran the above code.
ResourceExhaustedError (see above for traceback): OOM when allocating tensor with shape[2500,300,80,500]...
With a little modification of the above code, it works properly on my 8GB GPU memory.
import tensorflow as tf
C,M,F,B=2500,500,80,300
U = tf.Variable(tf.truncated_normal([B, F, M],stddev=1.0 ,dtype=tf.float32)) #just for example
A = tf.Variable(tf.truncated_normal([C, B],stddev=1.0) ,dtype=tf.float32) #just for example
# shape=(C,B,1,1)
A_new = tf.expand_dims(tf.expand_dims(A,-1),-1)
U_t =
for ccc in range(C):
a = A_new[ccc,:]
a_broadcasted = tf.reduce_sum(tf.multiply(a, U),axis=0)
U_t.append(a_broadcasted)
U_tcs = tf.concat(U_t,axis=0)
answered Jan 1 at 8:46


giser_yuganggiser_yugang
1,6631419
1,6631419
thanks ;) it really helped.
– OndraN
Jan 1 at 16:30
add a comment |
thanks ;) it really helped.
– OndraN
Jan 1 at 16:30
thanks ;) it really helped.
– OndraN
Jan 1 at 16:30
thanks ;) it really helped.
– OndraN
Jan 1 at 16:30
add a comment |
Thanks for contributing an answer to Stack Overflow!
- Please be sure to answer the question. Provide details and share your research!
But avoid …
- Asking for help, clarification, or responding to other answers.
- Making statements based on opinion; back them up with references or personal experience.
To learn more, see our tips on writing great answers.
Sign up or log in
StackExchange.ready(function () {
StackExchange.helpers.onClickDraftSave('#login-link');
});
Sign up using Google
Sign up using Facebook
Sign up using Email and Password
Post as a guest
Required, but never shown
StackExchange.ready(
function () {
StackExchange.openid.initPostLogin('.new-post-login', 'https%3a%2f%2fstackoverflow.com%2fquestions%2f53990649%2fhow-to-do-weighted-sum-as-tensor-operation-in-tensorflow%23new-answer', 'question_page');
}
);
Post as a guest
Required, but never shown
Sign up or log in
StackExchange.ready(function () {
StackExchange.helpers.onClickDraftSave('#login-link');
});
Sign up using Google
Sign up using Facebook
Sign up using Email and Password
Post as a guest
Required, but never shown
Sign up or log in
StackExchange.ready(function () {
StackExchange.helpers.onClickDraftSave('#login-link');
});
Sign up using Google
Sign up using Facebook
Sign up using Email and Password
Post as a guest
Required, but never shown
Sign up or log in
StackExchange.ready(function () {
StackExchange.helpers.onClickDraftSave('#login-link');
});
Sign up using Google
Sign up using Facebook
Sign up using Email and Password
Sign up using Google
Sign up using Facebook
Sign up using Email and Password
Post as a guest
Required, but never shown
Required, but never shown
Required, but never shown
Required, but never shown
Required, but never shown
Required, but never shown
Required, but never shown
Required, but never shown
Required, but never shown
vpFZmoV,X
Question is difficult to understanding with matrix dimensions alone. Probably asking using a picture or explaining the application use-case (like trying to something with image, etc) might help
– solver149
Dec 31 '18 at 21:03