Transform from one decision tree (J48) classification to ensemble in python
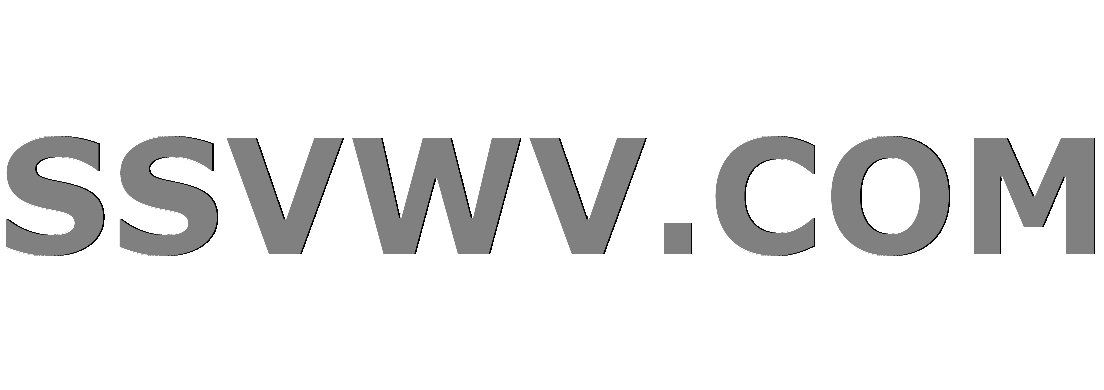
Multi tool use
I would like to implement the classification of the algorithm based on the paper. I have a single J48
(C4.5) decision tree (code mentioned down). I would like to run it for several (I_max
) times over the dataset and calculate the C* = class membership probabilities for all the ensemble. As described here and in page 8 in the paper.
import numpy as np
import pandas as pd
from sklearn.cross_validation import train_test_split
from sklearn.tree import DecisionTreeClassifier
from sklearn.metrics import accuracy_score
from sklearn import tree
url="https://archive.ics.uci.edu/ml/machine-learning-databases/abalone/abalone.data"
c=pd.read_csv(url, header=None)
X = c.values[:,1:8]
Y = c.values[:,0]
X_train, X_test, y_train, y_test = train_test_split( X, Y, test_size = 0.3, random_state = 100)
clf_entropy = DecisionTreeClassifier(criterion = "entropy", random_state = 100,
max_depth=3, min_samples_leaf=5)
clf_entropy.fit(X_train, y_train)
probs = clf_entropy.predict_proba(X_test)
probs
python scikit-learn decision-tree j48 c4.5
add a comment |
I would like to implement the classification of the algorithm based on the paper. I have a single J48
(C4.5) decision tree (code mentioned down). I would like to run it for several (I_max
) times over the dataset and calculate the C* = class membership probabilities for all the ensemble. As described here and in page 8 in the paper.
import numpy as np
import pandas as pd
from sklearn.cross_validation import train_test_split
from sklearn.tree import DecisionTreeClassifier
from sklearn.metrics import accuracy_score
from sklearn import tree
url="https://archive.ics.uci.edu/ml/machine-learning-databases/abalone/abalone.data"
c=pd.read_csv(url, header=None)
X = c.values[:,1:8]
Y = c.values[:,0]
X_train, X_test, y_train, y_test = train_test_split( X, Y, test_size = 0.3, random_state = 100)
clf_entropy = DecisionTreeClassifier(criterion = "entropy", random_state = 100,
max_depth=3, min_samples_leaf=5)
clf_entropy.fit(X_train, y_train)
probs = clf_entropy.predict_proba(X_test)
probs
python scikit-learn decision-tree j48 c4.5
add a comment |
I would like to implement the classification of the algorithm based on the paper. I have a single J48
(C4.5) decision tree (code mentioned down). I would like to run it for several (I_max
) times over the dataset and calculate the C* = class membership probabilities for all the ensemble. As described here and in page 8 in the paper.
import numpy as np
import pandas as pd
from sklearn.cross_validation import train_test_split
from sklearn.tree import DecisionTreeClassifier
from sklearn.metrics import accuracy_score
from sklearn import tree
url="https://archive.ics.uci.edu/ml/machine-learning-databases/abalone/abalone.data"
c=pd.read_csv(url, header=None)
X = c.values[:,1:8]
Y = c.values[:,0]
X_train, X_test, y_train, y_test = train_test_split( X, Y, test_size = 0.3, random_state = 100)
clf_entropy = DecisionTreeClassifier(criterion = "entropy", random_state = 100,
max_depth=3, min_samples_leaf=5)
clf_entropy.fit(X_train, y_train)
probs = clf_entropy.predict_proba(X_test)
probs
python scikit-learn decision-tree j48 c4.5
I would like to implement the classification of the algorithm based on the paper. I have a single J48
(C4.5) decision tree (code mentioned down). I would like to run it for several (I_max
) times over the dataset and calculate the C* = class membership probabilities for all the ensemble. As described here and in page 8 in the paper.
import numpy as np
import pandas as pd
from sklearn.cross_validation import train_test_split
from sklearn.tree import DecisionTreeClassifier
from sklearn.metrics import accuracy_score
from sklearn import tree
url="https://archive.ics.uci.edu/ml/machine-learning-databases/abalone/abalone.data"
c=pd.read_csv(url, header=None)
X = c.values[:,1:8]
Y = c.values[:,0]
X_train, X_test, y_train, y_test = train_test_split( X, Y, test_size = 0.3, random_state = 100)
clf_entropy = DecisionTreeClassifier(criterion = "entropy", random_state = 100,
max_depth=3, min_samples_leaf=5)
clf_entropy.fit(X_train, y_train)
probs = clf_entropy.predict_proba(X_test)
probs
python scikit-learn decision-tree j48 c4.5
python scikit-learn decision-tree j48 c4.5
asked Dec 31 '18 at 12:13
AviAvi
1,0261632
1,0261632
add a comment |
add a comment |
1 Answer
1
active
oldest
votes
Here is my implementation of Decorate based on the proposed algorithm in the mentioned paper. Feel free to improve the solution.
class EnsembleClasifier():
def __init__(self,base_classifier,labels):
self.classifier = [base_classifier]
self.labels = labels
def add_classifier(self,classifier):
self.classifier.append(classifier)
def remove_last_classifier(self):
self.classifier.pop(-1)
def predict_proba(self,X):
return np.array([clf.predict_proba(X) for clf in self.classifier]).sum(axis=0)/len(self.classifier)
def predict(self,X):
return labels[np.argmax(self.predict_proba(X),axis=1)]
def error(self,X,y):
return 1 - accuracy_score(y,ensembleClasifier.predict(X))
class Artificial_data():
def __init__(self,X,y,dtypes):
self.dtypes = {}
self._generator = {}
self.labels = y.unique()
for c,dtype in zip(X.columns,dtypes):
self.dtypes[c] = dtype
if dtype == 'numeric':
self._generator[c] = {'mean':X[c].mean(),'std':X[c].std()}
else:
unique_values = X[c].value_counts() / X.shape[0]
self._generator[c] = {'values':unique_values.index,'prob':unique_values.values}
def sample_generator(self,ensembleClasifier,nb_samples=1):
syn_X = pd.DataFrame()
for c in self.dtypes.keys():
if self.dtypes[c] == 'numeric':
syn_X[c] = np.random.normal(self._generator[c]['mean'],self._generator[c]['std'],nb_samples)
else:
syn_X[c] = np.random.choice(self._generator[c]['values'],p=self._generator[c]['prob'],
size=nb_samples,replace=True)
p_hat = ensembleClasifier.predict_proba(syn_X)
p_hat[p_hat==0] = 1e-5
inverse_p = 1/p_hat
new_p = inverse_p / inverse_p.sum(axis=1)[:, np.newaxis]
syn_y = [np.random.choice(self.labels,p=new_p[i]) for i in range(nb_samples)]
return syn_X,syn_y
import numpy as np
import pandas as pd
from sklearn.model_selection import train_test_split
from sklearn.tree import DecisionTreeClassifier
from sklearn.metrics import accuracy_score
from sklearn import datasets
iris = datasets.load_iris()
X, y = iris.data, iris.target
X_train_base, X_test, y_train_base, y_test = train_test_split( pd.DataFrame(X), pd.Series(y),
test_size = 0.3, random_state = 100)
# dtypes=['numeric' for _ in range(7)] + ['nominal'] #use this for abalone dataset
dtypes=['numeric' for _ in range(4)]
np.random.seed(1)
artifical_data = Artificial_data(X_train_base,y_train_base,dtypes)
c_size = 15
i_max = 300
R_size = len(X_train_base)
i = 1
trails =1
labels = np.unique(y_train_base)
clf_entropy = DecisionTreeClassifier(random_state = 1, max_depth=2)
clf_entropy.fit(X_train_base, y_train_base)
ensembleClasifier = EnsembleClasifier(clf_entropy,labels)
error_bst = ensembleClasifier.error(X_train_base,y_train_base)
while (i<c_size and trails<i_max):
X_syn,y_syn =artifical_data.sample_generator(ensembleClasifier,R_size)
X_train=pd.concat([X_train_base,X_syn],axis=0)
y_train=np.append(y_train_base,y_syn,axis=0)
C_prime=DecisionTreeClassifier( random_state = 1, max_depth=2)
C_prime.fit(X_train, y_train)
ensembleClasifier.add_classifier(C_prime)
error_i = ensembleClasifier.error(X_train_base,y_train_base)
if error_i <= error_bst:
print('improvement')
error_bst = error_i
print(error_i)
i += 1
else:
ensembleClasifier.remove_last_classifier()
trails +=1
Thanks a lot @AI_Learning, Is there a way to implement your code for the following dataset, as well? (archive.ics.uci.edu/ml/machine-learning-databases/abalone/…)
– Avi
Jan 2 at 5:55
1
Just try changing dtypes, # dtypes=['numeric' for _ in range(7)] + ['nominal'] #use this for abalone dataset
– AI_Learning
Jan 2 at 6:44
add a comment |
Your Answer
StackExchange.ifUsing("editor", function () {
StackExchange.using("externalEditor", function () {
StackExchange.using("snippets", function () {
StackExchange.snippets.init();
});
});
}, "code-snippets");
StackExchange.ready(function() {
var channelOptions = {
tags: "".split(" "),
id: "1"
};
initTagRenderer("".split(" "), "".split(" "), channelOptions);
StackExchange.using("externalEditor", function() {
// Have to fire editor after snippets, if snippets enabled
if (StackExchange.settings.snippets.snippetsEnabled) {
StackExchange.using("snippets", function() {
createEditor();
});
}
else {
createEditor();
}
});
function createEditor() {
StackExchange.prepareEditor({
heartbeatType: 'answer',
autoActivateHeartbeat: false,
convertImagesToLinks: true,
noModals: true,
showLowRepImageUploadWarning: true,
reputationToPostImages: 10,
bindNavPrevention: true,
postfix: "",
imageUploader: {
brandingHtml: "Powered by u003ca class="icon-imgur-white" href="https://imgur.com/"u003eu003c/au003e",
contentPolicyHtml: "User contributions licensed under u003ca href="https://creativecommons.org/licenses/by-sa/3.0/"u003ecc by-sa 3.0 with attribution requiredu003c/au003e u003ca href="https://stackoverflow.com/legal/content-policy"u003e(content policy)u003c/au003e",
allowUrls: true
},
onDemand: true,
discardSelector: ".discard-answer"
,immediatelyShowMarkdownHelp:true
});
}
});
Sign up or log in
StackExchange.ready(function () {
StackExchange.helpers.onClickDraftSave('#login-link');
});
Sign up using Google
Sign up using Facebook
Sign up using Email and Password
Post as a guest
Required, but never shown
StackExchange.ready(
function () {
StackExchange.openid.initPostLogin('.new-post-login', 'https%3a%2f%2fstackoverflow.com%2fquestions%2f53987391%2ftransform-from-one-decision-tree-j48-classification-to-ensemble-in-python%23new-answer', 'question_page');
}
);
Post as a guest
Required, but never shown
1 Answer
1
active
oldest
votes
1 Answer
1
active
oldest
votes
active
oldest
votes
active
oldest
votes
Here is my implementation of Decorate based on the proposed algorithm in the mentioned paper. Feel free to improve the solution.
class EnsembleClasifier():
def __init__(self,base_classifier,labels):
self.classifier = [base_classifier]
self.labels = labels
def add_classifier(self,classifier):
self.classifier.append(classifier)
def remove_last_classifier(self):
self.classifier.pop(-1)
def predict_proba(self,X):
return np.array([clf.predict_proba(X) for clf in self.classifier]).sum(axis=0)/len(self.classifier)
def predict(self,X):
return labels[np.argmax(self.predict_proba(X),axis=1)]
def error(self,X,y):
return 1 - accuracy_score(y,ensembleClasifier.predict(X))
class Artificial_data():
def __init__(self,X,y,dtypes):
self.dtypes = {}
self._generator = {}
self.labels = y.unique()
for c,dtype in zip(X.columns,dtypes):
self.dtypes[c] = dtype
if dtype == 'numeric':
self._generator[c] = {'mean':X[c].mean(),'std':X[c].std()}
else:
unique_values = X[c].value_counts() / X.shape[0]
self._generator[c] = {'values':unique_values.index,'prob':unique_values.values}
def sample_generator(self,ensembleClasifier,nb_samples=1):
syn_X = pd.DataFrame()
for c in self.dtypes.keys():
if self.dtypes[c] == 'numeric':
syn_X[c] = np.random.normal(self._generator[c]['mean'],self._generator[c]['std'],nb_samples)
else:
syn_X[c] = np.random.choice(self._generator[c]['values'],p=self._generator[c]['prob'],
size=nb_samples,replace=True)
p_hat = ensembleClasifier.predict_proba(syn_X)
p_hat[p_hat==0] = 1e-5
inverse_p = 1/p_hat
new_p = inverse_p / inverse_p.sum(axis=1)[:, np.newaxis]
syn_y = [np.random.choice(self.labels,p=new_p[i]) for i in range(nb_samples)]
return syn_X,syn_y
import numpy as np
import pandas as pd
from sklearn.model_selection import train_test_split
from sklearn.tree import DecisionTreeClassifier
from sklearn.metrics import accuracy_score
from sklearn import datasets
iris = datasets.load_iris()
X, y = iris.data, iris.target
X_train_base, X_test, y_train_base, y_test = train_test_split( pd.DataFrame(X), pd.Series(y),
test_size = 0.3, random_state = 100)
# dtypes=['numeric' for _ in range(7)] + ['nominal'] #use this for abalone dataset
dtypes=['numeric' for _ in range(4)]
np.random.seed(1)
artifical_data = Artificial_data(X_train_base,y_train_base,dtypes)
c_size = 15
i_max = 300
R_size = len(X_train_base)
i = 1
trails =1
labels = np.unique(y_train_base)
clf_entropy = DecisionTreeClassifier(random_state = 1, max_depth=2)
clf_entropy.fit(X_train_base, y_train_base)
ensembleClasifier = EnsembleClasifier(clf_entropy,labels)
error_bst = ensembleClasifier.error(X_train_base,y_train_base)
while (i<c_size and trails<i_max):
X_syn,y_syn =artifical_data.sample_generator(ensembleClasifier,R_size)
X_train=pd.concat([X_train_base,X_syn],axis=0)
y_train=np.append(y_train_base,y_syn,axis=0)
C_prime=DecisionTreeClassifier( random_state = 1, max_depth=2)
C_prime.fit(X_train, y_train)
ensembleClasifier.add_classifier(C_prime)
error_i = ensembleClasifier.error(X_train_base,y_train_base)
if error_i <= error_bst:
print('improvement')
error_bst = error_i
print(error_i)
i += 1
else:
ensembleClasifier.remove_last_classifier()
trails +=1
Thanks a lot @AI_Learning, Is there a way to implement your code for the following dataset, as well? (archive.ics.uci.edu/ml/machine-learning-databases/abalone/…)
– Avi
Jan 2 at 5:55
1
Just try changing dtypes, # dtypes=['numeric' for _ in range(7)] + ['nominal'] #use this for abalone dataset
– AI_Learning
Jan 2 at 6:44
add a comment |
Here is my implementation of Decorate based on the proposed algorithm in the mentioned paper. Feel free to improve the solution.
class EnsembleClasifier():
def __init__(self,base_classifier,labels):
self.classifier = [base_classifier]
self.labels = labels
def add_classifier(self,classifier):
self.classifier.append(classifier)
def remove_last_classifier(self):
self.classifier.pop(-1)
def predict_proba(self,X):
return np.array([clf.predict_proba(X) for clf in self.classifier]).sum(axis=0)/len(self.classifier)
def predict(self,X):
return labels[np.argmax(self.predict_proba(X),axis=1)]
def error(self,X,y):
return 1 - accuracy_score(y,ensembleClasifier.predict(X))
class Artificial_data():
def __init__(self,X,y,dtypes):
self.dtypes = {}
self._generator = {}
self.labels = y.unique()
for c,dtype in zip(X.columns,dtypes):
self.dtypes[c] = dtype
if dtype == 'numeric':
self._generator[c] = {'mean':X[c].mean(),'std':X[c].std()}
else:
unique_values = X[c].value_counts() / X.shape[0]
self._generator[c] = {'values':unique_values.index,'prob':unique_values.values}
def sample_generator(self,ensembleClasifier,nb_samples=1):
syn_X = pd.DataFrame()
for c in self.dtypes.keys():
if self.dtypes[c] == 'numeric':
syn_X[c] = np.random.normal(self._generator[c]['mean'],self._generator[c]['std'],nb_samples)
else:
syn_X[c] = np.random.choice(self._generator[c]['values'],p=self._generator[c]['prob'],
size=nb_samples,replace=True)
p_hat = ensembleClasifier.predict_proba(syn_X)
p_hat[p_hat==0] = 1e-5
inverse_p = 1/p_hat
new_p = inverse_p / inverse_p.sum(axis=1)[:, np.newaxis]
syn_y = [np.random.choice(self.labels,p=new_p[i]) for i in range(nb_samples)]
return syn_X,syn_y
import numpy as np
import pandas as pd
from sklearn.model_selection import train_test_split
from sklearn.tree import DecisionTreeClassifier
from sklearn.metrics import accuracy_score
from sklearn import datasets
iris = datasets.load_iris()
X, y = iris.data, iris.target
X_train_base, X_test, y_train_base, y_test = train_test_split( pd.DataFrame(X), pd.Series(y),
test_size = 0.3, random_state = 100)
# dtypes=['numeric' for _ in range(7)] + ['nominal'] #use this for abalone dataset
dtypes=['numeric' for _ in range(4)]
np.random.seed(1)
artifical_data = Artificial_data(X_train_base,y_train_base,dtypes)
c_size = 15
i_max = 300
R_size = len(X_train_base)
i = 1
trails =1
labels = np.unique(y_train_base)
clf_entropy = DecisionTreeClassifier(random_state = 1, max_depth=2)
clf_entropy.fit(X_train_base, y_train_base)
ensembleClasifier = EnsembleClasifier(clf_entropy,labels)
error_bst = ensembleClasifier.error(X_train_base,y_train_base)
while (i<c_size and trails<i_max):
X_syn,y_syn =artifical_data.sample_generator(ensembleClasifier,R_size)
X_train=pd.concat([X_train_base,X_syn],axis=0)
y_train=np.append(y_train_base,y_syn,axis=0)
C_prime=DecisionTreeClassifier( random_state = 1, max_depth=2)
C_prime.fit(X_train, y_train)
ensembleClasifier.add_classifier(C_prime)
error_i = ensembleClasifier.error(X_train_base,y_train_base)
if error_i <= error_bst:
print('improvement')
error_bst = error_i
print(error_i)
i += 1
else:
ensembleClasifier.remove_last_classifier()
trails +=1
Thanks a lot @AI_Learning, Is there a way to implement your code for the following dataset, as well? (archive.ics.uci.edu/ml/machine-learning-databases/abalone/…)
– Avi
Jan 2 at 5:55
1
Just try changing dtypes, # dtypes=['numeric' for _ in range(7)] + ['nominal'] #use this for abalone dataset
– AI_Learning
Jan 2 at 6:44
add a comment |
Here is my implementation of Decorate based on the proposed algorithm in the mentioned paper. Feel free to improve the solution.
class EnsembleClasifier():
def __init__(self,base_classifier,labels):
self.classifier = [base_classifier]
self.labels = labels
def add_classifier(self,classifier):
self.classifier.append(classifier)
def remove_last_classifier(self):
self.classifier.pop(-1)
def predict_proba(self,X):
return np.array([clf.predict_proba(X) for clf in self.classifier]).sum(axis=0)/len(self.classifier)
def predict(self,X):
return labels[np.argmax(self.predict_proba(X),axis=1)]
def error(self,X,y):
return 1 - accuracy_score(y,ensembleClasifier.predict(X))
class Artificial_data():
def __init__(self,X,y,dtypes):
self.dtypes = {}
self._generator = {}
self.labels = y.unique()
for c,dtype in zip(X.columns,dtypes):
self.dtypes[c] = dtype
if dtype == 'numeric':
self._generator[c] = {'mean':X[c].mean(),'std':X[c].std()}
else:
unique_values = X[c].value_counts() / X.shape[0]
self._generator[c] = {'values':unique_values.index,'prob':unique_values.values}
def sample_generator(self,ensembleClasifier,nb_samples=1):
syn_X = pd.DataFrame()
for c in self.dtypes.keys():
if self.dtypes[c] == 'numeric':
syn_X[c] = np.random.normal(self._generator[c]['mean'],self._generator[c]['std'],nb_samples)
else:
syn_X[c] = np.random.choice(self._generator[c]['values'],p=self._generator[c]['prob'],
size=nb_samples,replace=True)
p_hat = ensembleClasifier.predict_proba(syn_X)
p_hat[p_hat==0] = 1e-5
inverse_p = 1/p_hat
new_p = inverse_p / inverse_p.sum(axis=1)[:, np.newaxis]
syn_y = [np.random.choice(self.labels,p=new_p[i]) for i in range(nb_samples)]
return syn_X,syn_y
import numpy as np
import pandas as pd
from sklearn.model_selection import train_test_split
from sklearn.tree import DecisionTreeClassifier
from sklearn.metrics import accuracy_score
from sklearn import datasets
iris = datasets.load_iris()
X, y = iris.data, iris.target
X_train_base, X_test, y_train_base, y_test = train_test_split( pd.DataFrame(X), pd.Series(y),
test_size = 0.3, random_state = 100)
# dtypes=['numeric' for _ in range(7)] + ['nominal'] #use this for abalone dataset
dtypes=['numeric' for _ in range(4)]
np.random.seed(1)
artifical_data = Artificial_data(X_train_base,y_train_base,dtypes)
c_size = 15
i_max = 300
R_size = len(X_train_base)
i = 1
trails =1
labels = np.unique(y_train_base)
clf_entropy = DecisionTreeClassifier(random_state = 1, max_depth=2)
clf_entropy.fit(X_train_base, y_train_base)
ensembleClasifier = EnsembleClasifier(clf_entropy,labels)
error_bst = ensembleClasifier.error(X_train_base,y_train_base)
while (i<c_size and trails<i_max):
X_syn,y_syn =artifical_data.sample_generator(ensembleClasifier,R_size)
X_train=pd.concat([X_train_base,X_syn],axis=0)
y_train=np.append(y_train_base,y_syn,axis=0)
C_prime=DecisionTreeClassifier( random_state = 1, max_depth=2)
C_prime.fit(X_train, y_train)
ensembleClasifier.add_classifier(C_prime)
error_i = ensembleClasifier.error(X_train_base,y_train_base)
if error_i <= error_bst:
print('improvement')
error_bst = error_i
print(error_i)
i += 1
else:
ensembleClasifier.remove_last_classifier()
trails +=1
Here is my implementation of Decorate based on the proposed algorithm in the mentioned paper. Feel free to improve the solution.
class EnsembleClasifier():
def __init__(self,base_classifier,labels):
self.classifier = [base_classifier]
self.labels = labels
def add_classifier(self,classifier):
self.classifier.append(classifier)
def remove_last_classifier(self):
self.classifier.pop(-1)
def predict_proba(self,X):
return np.array([clf.predict_proba(X) for clf in self.classifier]).sum(axis=0)/len(self.classifier)
def predict(self,X):
return labels[np.argmax(self.predict_proba(X),axis=1)]
def error(self,X,y):
return 1 - accuracy_score(y,ensembleClasifier.predict(X))
class Artificial_data():
def __init__(self,X,y,dtypes):
self.dtypes = {}
self._generator = {}
self.labels = y.unique()
for c,dtype in zip(X.columns,dtypes):
self.dtypes[c] = dtype
if dtype == 'numeric':
self._generator[c] = {'mean':X[c].mean(),'std':X[c].std()}
else:
unique_values = X[c].value_counts() / X.shape[0]
self._generator[c] = {'values':unique_values.index,'prob':unique_values.values}
def sample_generator(self,ensembleClasifier,nb_samples=1):
syn_X = pd.DataFrame()
for c in self.dtypes.keys():
if self.dtypes[c] == 'numeric':
syn_X[c] = np.random.normal(self._generator[c]['mean'],self._generator[c]['std'],nb_samples)
else:
syn_X[c] = np.random.choice(self._generator[c]['values'],p=self._generator[c]['prob'],
size=nb_samples,replace=True)
p_hat = ensembleClasifier.predict_proba(syn_X)
p_hat[p_hat==0] = 1e-5
inverse_p = 1/p_hat
new_p = inverse_p / inverse_p.sum(axis=1)[:, np.newaxis]
syn_y = [np.random.choice(self.labels,p=new_p[i]) for i in range(nb_samples)]
return syn_X,syn_y
import numpy as np
import pandas as pd
from sklearn.model_selection import train_test_split
from sklearn.tree import DecisionTreeClassifier
from sklearn.metrics import accuracy_score
from sklearn import datasets
iris = datasets.load_iris()
X, y = iris.data, iris.target
X_train_base, X_test, y_train_base, y_test = train_test_split( pd.DataFrame(X), pd.Series(y),
test_size = 0.3, random_state = 100)
# dtypes=['numeric' for _ in range(7)] + ['nominal'] #use this for abalone dataset
dtypes=['numeric' for _ in range(4)]
np.random.seed(1)
artifical_data = Artificial_data(X_train_base,y_train_base,dtypes)
c_size = 15
i_max = 300
R_size = len(X_train_base)
i = 1
trails =1
labels = np.unique(y_train_base)
clf_entropy = DecisionTreeClassifier(random_state = 1, max_depth=2)
clf_entropy.fit(X_train_base, y_train_base)
ensembleClasifier = EnsembleClasifier(clf_entropy,labels)
error_bst = ensembleClasifier.error(X_train_base,y_train_base)
while (i<c_size and trails<i_max):
X_syn,y_syn =artifical_data.sample_generator(ensembleClasifier,R_size)
X_train=pd.concat([X_train_base,X_syn],axis=0)
y_train=np.append(y_train_base,y_syn,axis=0)
C_prime=DecisionTreeClassifier( random_state = 1, max_depth=2)
C_prime.fit(X_train, y_train)
ensembleClasifier.add_classifier(C_prime)
error_i = ensembleClasifier.error(X_train_base,y_train_base)
if error_i <= error_bst:
print('improvement')
error_bst = error_i
print(error_i)
i += 1
else:
ensembleClasifier.remove_last_classifier()
trails +=1
edited Jan 1 at 3:57
answered Jan 1 at 2:47


AI_LearningAI_Learning
3,3462933
3,3462933
Thanks a lot @AI_Learning, Is there a way to implement your code for the following dataset, as well? (archive.ics.uci.edu/ml/machine-learning-databases/abalone/…)
– Avi
Jan 2 at 5:55
1
Just try changing dtypes, # dtypes=['numeric' for _ in range(7)] + ['nominal'] #use this for abalone dataset
– AI_Learning
Jan 2 at 6:44
add a comment |
Thanks a lot @AI_Learning, Is there a way to implement your code for the following dataset, as well? (archive.ics.uci.edu/ml/machine-learning-databases/abalone/…)
– Avi
Jan 2 at 5:55
1
Just try changing dtypes, # dtypes=['numeric' for _ in range(7)] + ['nominal'] #use this for abalone dataset
– AI_Learning
Jan 2 at 6:44
Thanks a lot @AI_Learning, Is there a way to implement your code for the following dataset, as well? (archive.ics.uci.edu/ml/machine-learning-databases/abalone/…)
– Avi
Jan 2 at 5:55
Thanks a lot @AI_Learning, Is there a way to implement your code for the following dataset, as well? (archive.ics.uci.edu/ml/machine-learning-databases/abalone/…)
– Avi
Jan 2 at 5:55
1
1
Just try changing dtypes, # dtypes=['numeric' for _ in range(7)] + ['nominal'] #use this for abalone dataset
– AI_Learning
Jan 2 at 6:44
Just try changing dtypes, # dtypes=['numeric' for _ in range(7)] + ['nominal'] #use this for abalone dataset
– AI_Learning
Jan 2 at 6:44
add a comment |
Thanks for contributing an answer to Stack Overflow!
- Please be sure to answer the question. Provide details and share your research!
But avoid …
- Asking for help, clarification, or responding to other answers.
- Making statements based on opinion; back them up with references or personal experience.
To learn more, see our tips on writing great answers.
Sign up or log in
StackExchange.ready(function () {
StackExchange.helpers.onClickDraftSave('#login-link');
});
Sign up using Google
Sign up using Facebook
Sign up using Email and Password
Post as a guest
Required, but never shown
StackExchange.ready(
function () {
StackExchange.openid.initPostLogin('.new-post-login', 'https%3a%2f%2fstackoverflow.com%2fquestions%2f53987391%2ftransform-from-one-decision-tree-j48-classification-to-ensemble-in-python%23new-answer', 'question_page');
}
);
Post as a guest
Required, but never shown
Sign up or log in
StackExchange.ready(function () {
StackExchange.helpers.onClickDraftSave('#login-link');
});
Sign up using Google
Sign up using Facebook
Sign up using Email and Password
Post as a guest
Required, but never shown
Sign up or log in
StackExchange.ready(function () {
StackExchange.helpers.onClickDraftSave('#login-link');
});
Sign up using Google
Sign up using Facebook
Sign up using Email and Password
Post as a guest
Required, but never shown
Sign up or log in
StackExchange.ready(function () {
StackExchange.helpers.onClickDraftSave('#login-link');
});
Sign up using Google
Sign up using Facebook
Sign up using Email and Password
Sign up using Google
Sign up using Facebook
Sign up using Email and Password
Post as a guest
Required, but never shown
Required, but never shown
Required, but never shown
Required, but never shown
Required, but never shown
Required, but never shown
Required, but never shown
Required, but never shown
Required, but never shown
0Xt0 N4n0X4HiUiPrOcW,KI1SUOETi,RSGnYWP6Y3TvGoCs5n6KL2E6sTNuqEsRJuVVlNjwSptvkrB,UX