Having trouble with Bayesian Inference model - JAGS with R
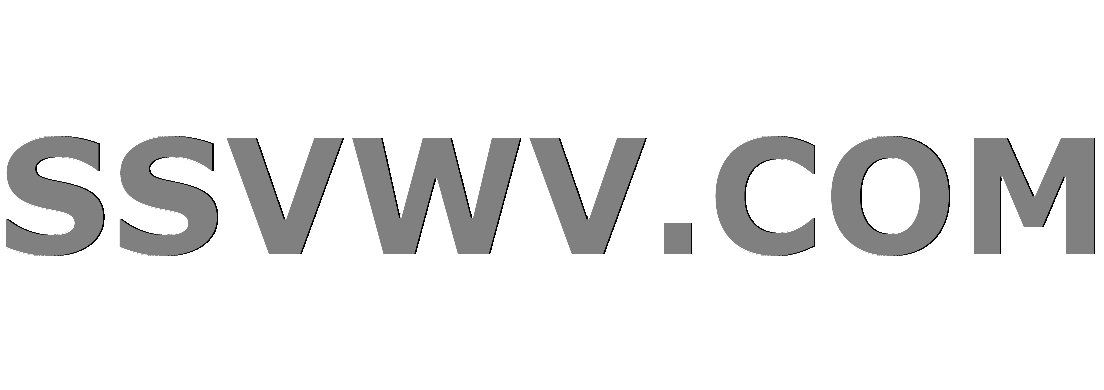
Multi tool use
I've been trying to reproduce the results of the following paper using R and JAGS with no success. I can get the model to run, but the results shown are consistently different.
Link for the paper: https://www.pmi.org/learning/library/bayesian-approach-earned-value-management-2260
The purpose of the paper is to use data gathered from project management reports to estimate project completion date or budget at completion, for instance. Project performance are mostly reported with the use of Earned Value measurement that consist basically on a ratio between actual work completed and the amount of work that was planned to be completed up to a milestone date (in order words, 'Work Done/Planned Work'). So, if I have spent on the third month of the project $300.000 to produce a amount of work that I previously planned to spend $270.000, my Cost Perfomance Index (CPI) is 300.000/270.000 = 1.111. Similarly, if by the 3rd month I had completed a amount of work that correspond with the what was planned to be completed by the 2nd month, my Schedule Performance Index (SPI) is 2/3 = 0.667.
The general problem behind the paper is how to use the performance measurement to update the prior belief about final project performance.
My code is shown bellow. I had to perform a transformation on the data (adding 1 before taking the log(), because some of them would be negative and JAGS return a error (that's why the parameters on my model is different to what's shown on paper's Table 4).
The model used on the paper was lognormal as likelihood and prior for mu and sigma on Normal and Inverse Gamma, respectively. Since BUGS syntax uses tau = 1/(variance) as parameter for Normal and Lognormal, I used the Gamma distribution on tau (that made sense to me).
model_pmi <- function() {
for (i in 1:9) {
cpi_log[i] ~ dlnorm(mu_cpi, tau_cpi)
spi_log[i] ~ dlnorm(mu_spi, tau_spi)
}
tau_cpi ~ dgamma(75, 1)
mu_cpi ~ dnorm(0.734765, 558.126)
cpi_pred ~ dlnorm(mu_cpi, tau_cpi)
tau_spi ~ dgamma(75, 1.5)
mu_spi ~ dnorm(0.67784, 8265.285)
spi_pred ~ dlnorm(mu_spi, tau_spi)
}
model.file <- file.path(tempdir(), "model_pmi.txt")
write.model(model_pmi, model.file)
cpis <- c(0.486, 1.167, 0.856, 0.770, 1.552, 1.534, 1.268, 2.369, 2.921)
spis <- c(0.456, 1.350, 0.949, 0.922, 0.693, 0.109, 0.506, 0.588, 0.525)
cpi_log <- log(1+cpis)
spi_log <- log(1+spis)
data <- list("cpi_log", "spi_log")
params <- c("tau_cpi","mu_cpi","tau_spi", "mu_spi", "cpi_pred", "spi_pred")
inits <- function() { list(tau_cpi = 1, tau_spi = 1, mu_cpi = 1, mu_spi = 1, cpi_pred = 1, spi_pred = 1) }
out_test <- jags(data, inits, params, model.file, n.iter=10000)
out_test
The 95% CI (2.5%;97.5%) found on the paper is (1.05;2.35) for CPI and (0.55;1.525). The model presented the results shown below. For CPI, the results are fairly close, but when I saw the results for SPI, I figured it should be just chance.
Inference for Bugs model at
"C:UsersfelipAppDataLocalTempRtmpSWZ70g/model_pmi.txt", fit using jags,
3 chains, each with 10000 iterations (first 5000 discarded), n.thin = 5
n.sims = 3000 iterations saved
mu.vect sd.vect 2.5% 25% 50% 75% 97.5% Rhat n.eff
cpi_pred 1.691 0.399 1.043 1.406 1.639 1.918 2.610 1.001 2200
mu_cpi 0.500 0.043 0.416 0.471 0.500 0.529 0.585 1.001 3000
mu_spi 0.668 0.011 0.647 0.660 0.668 0.675 0.690 1.001 3000
spi_pred 2.122 0.893 0.892 1.499 1.942 2.567 4.340 1.001 3000
tau_cpi 20.023 2.654 15.202 18.209 19.911 21.726 25.496 1.001 3000
tau_spi 6.132 0.675 4.889 5.657 6.107 6.568 7.541 1.001 3000
deviance 230.411 19.207 194.463 217.506 230.091 243.074 269.147 1.001 3000
For each parameter, n.eff is a crude measure of effective sample size,
and Rhat is the potential scale reduction factor (at convergence, Rhat=1).
DIC info (using the rule, pD = var(deviance)/2)
pD = 184.5 and DIC = 414.9
DIC is an estimate of expected predictive error (lower deviance is better).
Been working on this for days, can't find what's missing or what's wrong.
r bayesian r2jags
add a comment |
I've been trying to reproduce the results of the following paper using R and JAGS with no success. I can get the model to run, but the results shown are consistently different.
Link for the paper: https://www.pmi.org/learning/library/bayesian-approach-earned-value-management-2260
The purpose of the paper is to use data gathered from project management reports to estimate project completion date or budget at completion, for instance. Project performance are mostly reported with the use of Earned Value measurement that consist basically on a ratio between actual work completed and the amount of work that was planned to be completed up to a milestone date (in order words, 'Work Done/Planned Work'). So, if I have spent on the third month of the project $300.000 to produce a amount of work that I previously planned to spend $270.000, my Cost Perfomance Index (CPI) is 300.000/270.000 = 1.111. Similarly, if by the 3rd month I had completed a amount of work that correspond with the what was planned to be completed by the 2nd month, my Schedule Performance Index (SPI) is 2/3 = 0.667.
The general problem behind the paper is how to use the performance measurement to update the prior belief about final project performance.
My code is shown bellow. I had to perform a transformation on the data (adding 1 before taking the log(), because some of them would be negative and JAGS return a error (that's why the parameters on my model is different to what's shown on paper's Table 4).
The model used on the paper was lognormal as likelihood and prior for mu and sigma on Normal and Inverse Gamma, respectively. Since BUGS syntax uses tau = 1/(variance) as parameter for Normal and Lognormal, I used the Gamma distribution on tau (that made sense to me).
model_pmi <- function() {
for (i in 1:9) {
cpi_log[i] ~ dlnorm(mu_cpi, tau_cpi)
spi_log[i] ~ dlnorm(mu_spi, tau_spi)
}
tau_cpi ~ dgamma(75, 1)
mu_cpi ~ dnorm(0.734765, 558.126)
cpi_pred ~ dlnorm(mu_cpi, tau_cpi)
tau_spi ~ dgamma(75, 1.5)
mu_spi ~ dnorm(0.67784, 8265.285)
spi_pred ~ dlnorm(mu_spi, tau_spi)
}
model.file <- file.path(tempdir(), "model_pmi.txt")
write.model(model_pmi, model.file)
cpis <- c(0.486, 1.167, 0.856, 0.770, 1.552, 1.534, 1.268, 2.369, 2.921)
spis <- c(0.456, 1.350, 0.949, 0.922, 0.693, 0.109, 0.506, 0.588, 0.525)
cpi_log <- log(1+cpis)
spi_log <- log(1+spis)
data <- list("cpi_log", "spi_log")
params <- c("tau_cpi","mu_cpi","tau_spi", "mu_spi", "cpi_pred", "spi_pred")
inits <- function() { list(tau_cpi = 1, tau_spi = 1, mu_cpi = 1, mu_spi = 1, cpi_pred = 1, spi_pred = 1) }
out_test <- jags(data, inits, params, model.file, n.iter=10000)
out_test
The 95% CI (2.5%;97.5%) found on the paper is (1.05;2.35) for CPI and (0.55;1.525). The model presented the results shown below. For CPI, the results are fairly close, but when I saw the results for SPI, I figured it should be just chance.
Inference for Bugs model at
"C:UsersfelipAppDataLocalTempRtmpSWZ70g/model_pmi.txt", fit using jags,
3 chains, each with 10000 iterations (first 5000 discarded), n.thin = 5
n.sims = 3000 iterations saved
mu.vect sd.vect 2.5% 25% 50% 75% 97.5% Rhat n.eff
cpi_pred 1.691 0.399 1.043 1.406 1.639 1.918 2.610 1.001 2200
mu_cpi 0.500 0.043 0.416 0.471 0.500 0.529 0.585 1.001 3000
mu_spi 0.668 0.011 0.647 0.660 0.668 0.675 0.690 1.001 3000
spi_pred 2.122 0.893 0.892 1.499 1.942 2.567 4.340 1.001 3000
tau_cpi 20.023 2.654 15.202 18.209 19.911 21.726 25.496 1.001 3000
tau_spi 6.132 0.675 4.889 5.657 6.107 6.568 7.541 1.001 3000
deviance 230.411 19.207 194.463 217.506 230.091 243.074 269.147 1.001 3000
For each parameter, n.eff is a crude measure of effective sample size,
and Rhat is the potential scale reduction factor (at convergence, Rhat=1).
DIC info (using the rule, pD = var(deviance)/2)
pD = 184.5 and DIC = 414.9
DIC is an estimate of expected predictive error (lower deviance is better).
Been working on this for days, can't find what's missing or what's wrong.
r bayesian r2jags
add a comment |
I've been trying to reproduce the results of the following paper using R and JAGS with no success. I can get the model to run, but the results shown are consistently different.
Link for the paper: https://www.pmi.org/learning/library/bayesian-approach-earned-value-management-2260
The purpose of the paper is to use data gathered from project management reports to estimate project completion date or budget at completion, for instance. Project performance are mostly reported with the use of Earned Value measurement that consist basically on a ratio between actual work completed and the amount of work that was planned to be completed up to a milestone date (in order words, 'Work Done/Planned Work'). So, if I have spent on the third month of the project $300.000 to produce a amount of work that I previously planned to spend $270.000, my Cost Perfomance Index (CPI) is 300.000/270.000 = 1.111. Similarly, if by the 3rd month I had completed a amount of work that correspond with the what was planned to be completed by the 2nd month, my Schedule Performance Index (SPI) is 2/3 = 0.667.
The general problem behind the paper is how to use the performance measurement to update the prior belief about final project performance.
My code is shown bellow. I had to perform a transformation on the data (adding 1 before taking the log(), because some of them would be negative and JAGS return a error (that's why the parameters on my model is different to what's shown on paper's Table 4).
The model used on the paper was lognormal as likelihood and prior for mu and sigma on Normal and Inverse Gamma, respectively. Since BUGS syntax uses tau = 1/(variance) as parameter for Normal and Lognormal, I used the Gamma distribution on tau (that made sense to me).
model_pmi <- function() {
for (i in 1:9) {
cpi_log[i] ~ dlnorm(mu_cpi, tau_cpi)
spi_log[i] ~ dlnorm(mu_spi, tau_spi)
}
tau_cpi ~ dgamma(75, 1)
mu_cpi ~ dnorm(0.734765, 558.126)
cpi_pred ~ dlnorm(mu_cpi, tau_cpi)
tau_spi ~ dgamma(75, 1.5)
mu_spi ~ dnorm(0.67784, 8265.285)
spi_pred ~ dlnorm(mu_spi, tau_spi)
}
model.file <- file.path(tempdir(), "model_pmi.txt")
write.model(model_pmi, model.file)
cpis <- c(0.486, 1.167, 0.856, 0.770, 1.552, 1.534, 1.268, 2.369, 2.921)
spis <- c(0.456, 1.350, 0.949, 0.922, 0.693, 0.109, 0.506, 0.588, 0.525)
cpi_log <- log(1+cpis)
spi_log <- log(1+spis)
data <- list("cpi_log", "spi_log")
params <- c("tau_cpi","mu_cpi","tau_spi", "mu_spi", "cpi_pred", "spi_pred")
inits <- function() { list(tau_cpi = 1, tau_spi = 1, mu_cpi = 1, mu_spi = 1, cpi_pred = 1, spi_pred = 1) }
out_test <- jags(data, inits, params, model.file, n.iter=10000)
out_test
The 95% CI (2.5%;97.5%) found on the paper is (1.05;2.35) for CPI and (0.55;1.525). The model presented the results shown below. For CPI, the results are fairly close, but when I saw the results for SPI, I figured it should be just chance.
Inference for Bugs model at
"C:UsersfelipAppDataLocalTempRtmpSWZ70g/model_pmi.txt", fit using jags,
3 chains, each with 10000 iterations (first 5000 discarded), n.thin = 5
n.sims = 3000 iterations saved
mu.vect sd.vect 2.5% 25% 50% 75% 97.5% Rhat n.eff
cpi_pred 1.691 0.399 1.043 1.406 1.639 1.918 2.610 1.001 2200
mu_cpi 0.500 0.043 0.416 0.471 0.500 0.529 0.585 1.001 3000
mu_spi 0.668 0.011 0.647 0.660 0.668 0.675 0.690 1.001 3000
spi_pred 2.122 0.893 0.892 1.499 1.942 2.567 4.340 1.001 3000
tau_cpi 20.023 2.654 15.202 18.209 19.911 21.726 25.496 1.001 3000
tau_spi 6.132 0.675 4.889 5.657 6.107 6.568 7.541 1.001 3000
deviance 230.411 19.207 194.463 217.506 230.091 243.074 269.147 1.001 3000
For each parameter, n.eff is a crude measure of effective sample size,
and Rhat is the potential scale reduction factor (at convergence, Rhat=1).
DIC info (using the rule, pD = var(deviance)/2)
pD = 184.5 and DIC = 414.9
DIC is an estimate of expected predictive error (lower deviance is better).
Been working on this for days, can't find what's missing or what's wrong.
r bayesian r2jags
I've been trying to reproduce the results of the following paper using R and JAGS with no success. I can get the model to run, but the results shown are consistently different.
Link for the paper: https://www.pmi.org/learning/library/bayesian-approach-earned-value-management-2260
The purpose of the paper is to use data gathered from project management reports to estimate project completion date or budget at completion, for instance. Project performance are mostly reported with the use of Earned Value measurement that consist basically on a ratio between actual work completed and the amount of work that was planned to be completed up to a milestone date (in order words, 'Work Done/Planned Work'). So, if I have spent on the third month of the project $300.000 to produce a amount of work that I previously planned to spend $270.000, my Cost Perfomance Index (CPI) is 300.000/270.000 = 1.111. Similarly, if by the 3rd month I had completed a amount of work that correspond with the what was planned to be completed by the 2nd month, my Schedule Performance Index (SPI) is 2/3 = 0.667.
The general problem behind the paper is how to use the performance measurement to update the prior belief about final project performance.
My code is shown bellow. I had to perform a transformation on the data (adding 1 before taking the log(), because some of them would be negative and JAGS return a error (that's why the parameters on my model is different to what's shown on paper's Table 4).
The model used on the paper was lognormal as likelihood and prior for mu and sigma on Normal and Inverse Gamma, respectively. Since BUGS syntax uses tau = 1/(variance) as parameter for Normal and Lognormal, I used the Gamma distribution on tau (that made sense to me).
model_pmi <- function() {
for (i in 1:9) {
cpi_log[i] ~ dlnorm(mu_cpi, tau_cpi)
spi_log[i] ~ dlnorm(mu_spi, tau_spi)
}
tau_cpi ~ dgamma(75, 1)
mu_cpi ~ dnorm(0.734765, 558.126)
cpi_pred ~ dlnorm(mu_cpi, tau_cpi)
tau_spi ~ dgamma(75, 1.5)
mu_spi ~ dnorm(0.67784, 8265.285)
spi_pred ~ dlnorm(mu_spi, tau_spi)
}
model.file <- file.path(tempdir(), "model_pmi.txt")
write.model(model_pmi, model.file)
cpis <- c(0.486, 1.167, 0.856, 0.770, 1.552, 1.534, 1.268, 2.369, 2.921)
spis <- c(0.456, 1.350, 0.949, 0.922, 0.693, 0.109, 0.506, 0.588, 0.525)
cpi_log <- log(1+cpis)
spi_log <- log(1+spis)
data <- list("cpi_log", "spi_log")
params <- c("tau_cpi","mu_cpi","tau_spi", "mu_spi", "cpi_pred", "spi_pred")
inits <- function() { list(tau_cpi = 1, tau_spi = 1, mu_cpi = 1, mu_spi = 1, cpi_pred = 1, spi_pred = 1) }
out_test <- jags(data, inits, params, model.file, n.iter=10000)
out_test
The 95% CI (2.5%;97.5%) found on the paper is (1.05;2.35) for CPI and (0.55;1.525). The model presented the results shown below. For CPI, the results are fairly close, but when I saw the results for SPI, I figured it should be just chance.
Inference for Bugs model at
"C:UsersfelipAppDataLocalTempRtmpSWZ70g/model_pmi.txt", fit using jags,
3 chains, each with 10000 iterations (first 5000 discarded), n.thin = 5
n.sims = 3000 iterations saved
mu.vect sd.vect 2.5% 25% 50% 75% 97.5% Rhat n.eff
cpi_pred 1.691 0.399 1.043 1.406 1.639 1.918 2.610 1.001 2200
mu_cpi 0.500 0.043 0.416 0.471 0.500 0.529 0.585 1.001 3000
mu_spi 0.668 0.011 0.647 0.660 0.668 0.675 0.690 1.001 3000
spi_pred 2.122 0.893 0.892 1.499 1.942 2.567 4.340 1.001 3000
tau_cpi 20.023 2.654 15.202 18.209 19.911 21.726 25.496 1.001 3000
tau_spi 6.132 0.675 4.889 5.657 6.107 6.568 7.541 1.001 3000
deviance 230.411 19.207 194.463 217.506 230.091 243.074 269.147 1.001 3000
For each parameter, n.eff is a crude measure of effective sample size,
and Rhat is the potential scale reduction factor (at convergence, Rhat=1).
DIC info (using the rule, pD = var(deviance)/2)
pD = 184.5 and DIC = 414.9
DIC is an estimate of expected predictive error (lower deviance is better).
Been working on this for days, can't find what's missing or what's wrong.
r bayesian r2jags
r bayesian r2jags
asked Dec 30 '18 at 14:35
Felipe MoreiraFelipe Moreira
11
11
add a comment |
add a comment |
1 Answer
1
active
oldest
votes
When using y ~ dlnorm(mu,tau)
, the y
value is the original-scale value, not the log-scale value. But mu
and tau
are on the log scale (which is confusing).
Also, putting priors directly on mu
and tau
can produce bad autocorrelation in the chains. Reparameterizing helps. For details, see this blog post (that I wrote): http://doingbayesiandataanalysis.blogspot.com/2016/04/bayesian-estimation-of-log-normal.html
Finally, the mean, mode, and SD on the original scale are somewhat complex transformations of mu and tau on the log scale. Again, see the blog post linked above.
First of all, I'm reading your book, great work. Second, I've modified the code so that the the params are obtained with logY and used data as Y only and got good results for the prediction of SPI, but bad results for CPI. That is strange as hell.
– Felipe Moreira
Dec 30 '18 at 22:14
Have you contacted the authors of the analysis you're trying to reproduce? That may be your best bet.
– John K. Kruschke
Dec 31 '18 at 11:54
Sounds like a plan. So, that means the modelling makes sense?
– Felipe Moreira
Jan 2 at 10:39
add a comment |
Your Answer
StackExchange.ifUsing("editor", function () {
StackExchange.using("externalEditor", function () {
StackExchange.using("snippets", function () {
StackExchange.snippets.init();
});
});
}, "code-snippets");
StackExchange.ready(function() {
var channelOptions = {
tags: "".split(" "),
id: "1"
};
initTagRenderer("".split(" "), "".split(" "), channelOptions);
StackExchange.using("externalEditor", function() {
// Have to fire editor after snippets, if snippets enabled
if (StackExchange.settings.snippets.snippetsEnabled) {
StackExchange.using("snippets", function() {
createEditor();
});
}
else {
createEditor();
}
});
function createEditor() {
StackExchange.prepareEditor({
heartbeatType: 'answer',
autoActivateHeartbeat: false,
convertImagesToLinks: true,
noModals: true,
showLowRepImageUploadWarning: true,
reputationToPostImages: 10,
bindNavPrevention: true,
postfix: "",
imageUploader: {
brandingHtml: "Powered by u003ca class="icon-imgur-white" href="https://imgur.com/"u003eu003c/au003e",
contentPolicyHtml: "User contributions licensed under u003ca href="https://creativecommons.org/licenses/by-sa/3.0/"u003ecc by-sa 3.0 with attribution requiredu003c/au003e u003ca href="https://stackoverflow.com/legal/content-policy"u003e(content policy)u003c/au003e",
allowUrls: true
},
onDemand: true,
discardSelector: ".discard-answer"
,immediatelyShowMarkdownHelp:true
});
}
});
Sign up or log in
StackExchange.ready(function () {
StackExchange.helpers.onClickDraftSave('#login-link');
});
Sign up using Google
Sign up using Facebook
Sign up using Email and Password
Post as a guest
Required, but never shown
StackExchange.ready(
function () {
StackExchange.openid.initPostLogin('.new-post-login', 'https%3a%2f%2fstackoverflow.com%2fquestions%2f53978502%2fhaving-trouble-with-bayesian-inference-model-jags-with-r%23new-answer', 'question_page');
}
);
Post as a guest
Required, but never shown
1 Answer
1
active
oldest
votes
1 Answer
1
active
oldest
votes
active
oldest
votes
active
oldest
votes
When using y ~ dlnorm(mu,tau)
, the y
value is the original-scale value, not the log-scale value. But mu
and tau
are on the log scale (which is confusing).
Also, putting priors directly on mu
and tau
can produce bad autocorrelation in the chains. Reparameterizing helps. For details, see this blog post (that I wrote): http://doingbayesiandataanalysis.blogspot.com/2016/04/bayesian-estimation-of-log-normal.html
Finally, the mean, mode, and SD on the original scale are somewhat complex transformations of mu and tau on the log scale. Again, see the blog post linked above.
First of all, I'm reading your book, great work. Second, I've modified the code so that the the params are obtained with logY and used data as Y only and got good results for the prediction of SPI, but bad results for CPI. That is strange as hell.
– Felipe Moreira
Dec 30 '18 at 22:14
Have you contacted the authors of the analysis you're trying to reproduce? That may be your best bet.
– John K. Kruschke
Dec 31 '18 at 11:54
Sounds like a plan. So, that means the modelling makes sense?
– Felipe Moreira
Jan 2 at 10:39
add a comment |
When using y ~ dlnorm(mu,tau)
, the y
value is the original-scale value, not the log-scale value. But mu
and tau
are on the log scale (which is confusing).
Also, putting priors directly on mu
and tau
can produce bad autocorrelation in the chains. Reparameterizing helps. For details, see this blog post (that I wrote): http://doingbayesiandataanalysis.blogspot.com/2016/04/bayesian-estimation-of-log-normal.html
Finally, the mean, mode, and SD on the original scale are somewhat complex transformations of mu and tau on the log scale. Again, see the blog post linked above.
First of all, I'm reading your book, great work. Second, I've modified the code so that the the params are obtained with logY and used data as Y only and got good results for the prediction of SPI, but bad results for CPI. That is strange as hell.
– Felipe Moreira
Dec 30 '18 at 22:14
Have you contacted the authors of the analysis you're trying to reproduce? That may be your best bet.
– John K. Kruschke
Dec 31 '18 at 11:54
Sounds like a plan. So, that means the modelling makes sense?
– Felipe Moreira
Jan 2 at 10:39
add a comment |
When using y ~ dlnorm(mu,tau)
, the y
value is the original-scale value, not the log-scale value. But mu
and tau
are on the log scale (which is confusing).
Also, putting priors directly on mu
and tau
can produce bad autocorrelation in the chains. Reparameterizing helps. For details, see this blog post (that I wrote): http://doingbayesiandataanalysis.blogspot.com/2016/04/bayesian-estimation-of-log-normal.html
Finally, the mean, mode, and SD on the original scale are somewhat complex transformations of mu and tau on the log scale. Again, see the blog post linked above.
When using y ~ dlnorm(mu,tau)
, the y
value is the original-scale value, not the log-scale value. But mu
and tau
are on the log scale (which is confusing).
Also, putting priors directly on mu
and tau
can produce bad autocorrelation in the chains. Reparameterizing helps. For details, see this blog post (that I wrote): http://doingbayesiandataanalysis.blogspot.com/2016/04/bayesian-estimation-of-log-normal.html
Finally, the mean, mode, and SD on the original scale are somewhat complex transformations of mu and tau on the log scale. Again, see the blog post linked above.
answered Dec 30 '18 at 19:12


John K. KruschkeJohn K. Kruschke
225110
225110
First of all, I'm reading your book, great work. Second, I've modified the code so that the the params are obtained with logY and used data as Y only and got good results for the prediction of SPI, but bad results for CPI. That is strange as hell.
– Felipe Moreira
Dec 30 '18 at 22:14
Have you contacted the authors of the analysis you're trying to reproduce? That may be your best bet.
– John K. Kruschke
Dec 31 '18 at 11:54
Sounds like a plan. So, that means the modelling makes sense?
– Felipe Moreira
Jan 2 at 10:39
add a comment |
First of all, I'm reading your book, great work. Second, I've modified the code so that the the params are obtained with logY and used data as Y only and got good results for the prediction of SPI, but bad results for CPI. That is strange as hell.
– Felipe Moreira
Dec 30 '18 at 22:14
Have you contacted the authors of the analysis you're trying to reproduce? That may be your best bet.
– John K. Kruschke
Dec 31 '18 at 11:54
Sounds like a plan. So, that means the modelling makes sense?
– Felipe Moreira
Jan 2 at 10:39
First of all, I'm reading your book, great work. Second, I've modified the code so that the the params are obtained with logY and used data as Y only and got good results for the prediction of SPI, but bad results for CPI. That is strange as hell.
– Felipe Moreira
Dec 30 '18 at 22:14
First of all, I'm reading your book, great work. Second, I've modified the code so that the the params are obtained with logY and used data as Y only and got good results for the prediction of SPI, but bad results for CPI. That is strange as hell.
– Felipe Moreira
Dec 30 '18 at 22:14
Have you contacted the authors of the analysis you're trying to reproduce? That may be your best bet.
– John K. Kruschke
Dec 31 '18 at 11:54
Have you contacted the authors of the analysis you're trying to reproduce? That may be your best bet.
– John K. Kruschke
Dec 31 '18 at 11:54
Sounds like a plan. So, that means the modelling makes sense?
– Felipe Moreira
Jan 2 at 10:39
Sounds like a plan. So, that means the modelling makes sense?
– Felipe Moreira
Jan 2 at 10:39
add a comment |
Thanks for contributing an answer to Stack Overflow!
- Please be sure to answer the question. Provide details and share your research!
But avoid …
- Asking for help, clarification, or responding to other answers.
- Making statements based on opinion; back them up with references or personal experience.
To learn more, see our tips on writing great answers.
Sign up or log in
StackExchange.ready(function () {
StackExchange.helpers.onClickDraftSave('#login-link');
});
Sign up using Google
Sign up using Facebook
Sign up using Email and Password
Post as a guest
Required, but never shown
StackExchange.ready(
function () {
StackExchange.openid.initPostLogin('.new-post-login', 'https%3a%2f%2fstackoverflow.com%2fquestions%2f53978502%2fhaving-trouble-with-bayesian-inference-model-jags-with-r%23new-answer', 'question_page');
}
);
Post as a guest
Required, but never shown
Sign up or log in
StackExchange.ready(function () {
StackExchange.helpers.onClickDraftSave('#login-link');
});
Sign up using Google
Sign up using Facebook
Sign up using Email and Password
Post as a guest
Required, but never shown
Sign up or log in
StackExchange.ready(function () {
StackExchange.helpers.onClickDraftSave('#login-link');
});
Sign up using Google
Sign up using Facebook
Sign up using Email and Password
Post as a guest
Required, but never shown
Sign up or log in
StackExchange.ready(function () {
StackExchange.helpers.onClickDraftSave('#login-link');
});
Sign up using Google
Sign up using Facebook
Sign up using Email and Password
Sign up using Google
Sign up using Facebook
Sign up using Email and Password
Post as a guest
Required, but never shown
Required, but never shown
Required, but never shown
Required, but never shown
Required, but never shown
Required, but never shown
Required, but never shown
Required, but never shown
Required, but never shown
lnQ9l1,30m,uKwZ Q32GpBpkHkZgojwnRKLoymkrxZX