Algorithm for downsampling array of intervals
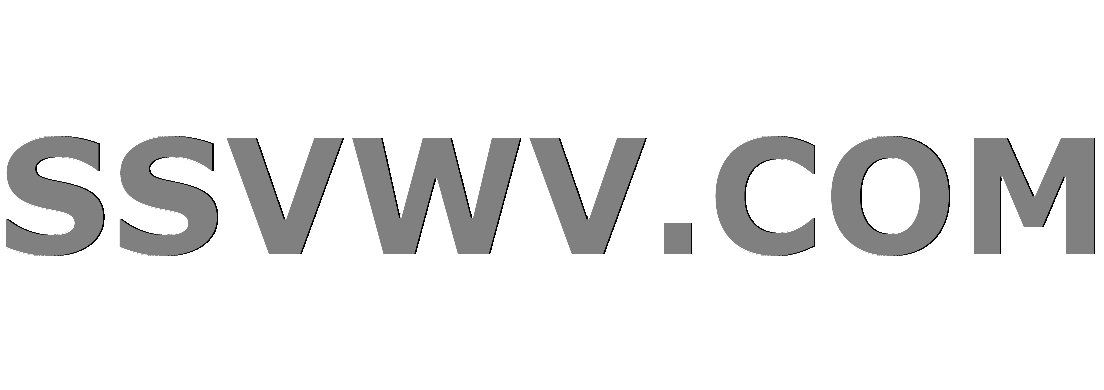
Multi tool use
I have a sorted array of N intervals of different length. I am plotting these intervals with alternating colors blue/green.
I am trying to find a method or algorithm to "downsample" the array of intervals to produce a visually similar plot, but with less elements.
Ideally I could write some function where I can pass the target number of output intervals as an argument. The output length only has to come close to the target.
input = [
[0, 5, "blue"],
[5, 6, "green"],
[6, 10, "blue"],
// ...etc
]
output = downsample(input, 25)
// [[0, 10, "blue"], ... ]
Below is a picture of what I am trying to accomplish. In this example the input has about 250 intervals, and the output about ~25 intervals. The input length can vary a lot.
algorithm intervals downsampling
add a comment |
I have a sorted array of N intervals of different length. I am plotting these intervals with alternating colors blue/green.
I am trying to find a method or algorithm to "downsample" the array of intervals to produce a visually similar plot, but with less elements.
Ideally I could write some function where I can pass the target number of output intervals as an argument. The output length only has to come close to the target.
input = [
[0, 5, "blue"],
[5, 6, "green"],
[6, 10, "blue"],
// ...etc
]
output = downsample(input, 25)
// [[0, 10, "blue"], ... ]
Below is a picture of what I am trying to accomplish. In this example the input has about 250 intervals, and the output about ~25 intervals. The input length can vary a lot.
algorithm intervals downsampling
Given N input intervals, exactly how many output intervals do you want? Or is there another way that the desired output is defined, like minimum interval size?
– Matt Timmermans
Dec 30 '18 at 16:21
@MattTimmermans the exact output number is not important, but I'd like to supply a maximum number of approximate outputs. Something likeoutput_length = min(len(input), 25)
– hampusohlsson
Dec 31 '18 at 9:15
add a comment |
I have a sorted array of N intervals of different length. I am plotting these intervals with alternating colors blue/green.
I am trying to find a method or algorithm to "downsample" the array of intervals to produce a visually similar plot, but with less elements.
Ideally I could write some function where I can pass the target number of output intervals as an argument. The output length only has to come close to the target.
input = [
[0, 5, "blue"],
[5, 6, "green"],
[6, 10, "blue"],
// ...etc
]
output = downsample(input, 25)
// [[0, 10, "blue"], ... ]
Below is a picture of what I am trying to accomplish. In this example the input has about 250 intervals, and the output about ~25 intervals. The input length can vary a lot.
algorithm intervals downsampling
I have a sorted array of N intervals of different length. I am plotting these intervals with alternating colors blue/green.
I am trying to find a method or algorithm to "downsample" the array of intervals to produce a visually similar plot, but with less elements.
Ideally I could write some function where I can pass the target number of output intervals as an argument. The output length only has to come close to the target.
input = [
[0, 5, "blue"],
[5, 6, "green"],
[6, 10, "blue"],
// ...etc
]
output = downsample(input, 25)
// [[0, 10, "blue"], ... ]
Below is a picture of what I am trying to accomplish. In this example the input has about 250 intervals, and the output about ~25 intervals. The input length can vary a lot.
algorithm intervals downsampling
algorithm intervals downsampling
asked Dec 30 '18 at 14:34


hampusohlssonhampusohlsson
4,91342442
4,91342442
Given N input intervals, exactly how many output intervals do you want? Or is there another way that the desired output is defined, like minimum interval size?
– Matt Timmermans
Dec 30 '18 at 16:21
@MattTimmermans the exact output number is not important, but I'd like to supply a maximum number of approximate outputs. Something likeoutput_length = min(len(input), 25)
– hampusohlsson
Dec 31 '18 at 9:15
add a comment |
Given N input intervals, exactly how many output intervals do you want? Or is there another way that the desired output is defined, like minimum interval size?
– Matt Timmermans
Dec 30 '18 at 16:21
@MattTimmermans the exact output number is not important, but I'd like to supply a maximum number of approximate outputs. Something likeoutput_length = min(len(input), 25)
– hampusohlsson
Dec 31 '18 at 9:15
Given N input intervals, exactly how many output intervals do you want? Or is there another way that the desired output is defined, like minimum interval size?
– Matt Timmermans
Dec 30 '18 at 16:21
Given N input intervals, exactly how many output intervals do you want? Or is there another way that the desired output is defined, like minimum interval size?
– Matt Timmermans
Dec 30 '18 at 16:21
@MattTimmermans the exact output number is not important, but I'd like to supply a maximum number of approximate outputs. Something like
output_length = min(len(input), 25)
– hampusohlsson
Dec 31 '18 at 9:15
@MattTimmermans the exact output number is not important, but I'd like to supply a maximum number of approximate outputs. Something like
output_length = min(len(input), 25)
– hampusohlsson
Dec 31 '18 at 9:15
add a comment |
5 Answers
5
active
oldest
votes
Update 1:
Below is my original post which I initially deleted, because there were issues with displaying the equations and also I wasn't very confident if it really makes sense. But later, I figured that the optimisation problem that I described can be actually solved efficiently with DP (Dynamic programming).
So I did a sample C++ implementation. Here are some results:
Here is a live demo that you can play with in your browser (make sure browser support WebGL2, like Chrome or Firefox). It takes a bit to load the page.
Here is the C++ implementation: link
Update 2:
Turns out the proposed solution has the following nice property - we can easily control the importance of the two parts F1 and F2 of the cost function. Simply change the cost function to F(α)=F1 + αF2, where α >= 1.0 is a free parameter. The DP algorithm remains the same.
Here are some result for different α values using the same number of intervals N:
Live demo (WebGL2 required)
As can be seen, higher α means it is more important to cover the original input intervals even if this means covering more of the background in-between.
Original post
Even-though some good algorithms have already been proposed, I would like to propose a slightly unusual approach - interpreting the task as an optimisation problem. Although, I don't know how to efficiently solve the optimisation problem (or even if it can be solved in reasonable time at all), it might be useful to someone purely as a concept.
First, without loss of generality, lets declare the blue color to be background. We will be painting N green intervals on top of it (N is the number provided to the downsample()
function in OP's description). The ith interval is defined by its starting coordinate 0 <= xi < xmax and width wi >= 0 (xmax is the maximum coordinate from the input).
Lets also define the array G(x) to be the number of green cells in the interval [0, x) in the input data. This array can easily be pre-calculated. We will use it to quickly calculate the number of green cells in arbitrary interval [x, y) - namely: G(y) - G(x).
We can now introduce the first part of the cost function for our optimisation problem:
The smaller F1 is, the better our generated intervals cover the input intervals, so we will be searching for xi, wi that minimise it. Ideally we want F1=0 which would mean that the intervals do not cover any of the background (which of course is not possible because N is less than the input intervals).
However, this function is not enough to describe the problem, because obviously we can minimise it by taking empty intervals: F1(x, 0)=0. Instead, we want to cover as much as possible from the input intervals. Lets introduce the second part of the cost function which corresponds to this requirement:
The smaller F2 is, the more input intervals are covered. Ideally we want F2=0 which would mean that we covered all of the input rectangles. However, minimising F2 competes with minimising F1.
Finally, we can state our optimisation problem: find xi, wi that minimize F=F1 + F2
How to solve this problem? Not sure. Maybe use some metaheuristic approach for global optimisation such as Simulated annealing or Differential evolution. These are typically easy to implement, especially for this simple cost function.
Best case would be to exist some kind of DP algorithm for solving it efficiently, but unlikely.
1
This is awesome, thanks for sharing and spending time on this @Georgi Gerganov
– hampusohlsson
Dec 31 '18 at 11:05
1
So I played around with your example - fabulous! Follow up question -- what if you introduce another color/dimension @Gregori Gerganov? Could this approach still work?
– hampusohlsson
Dec 31 '18 at 11:17
1
Sure. Again, declare one of the C >= 2 colors to be background and apply this same algorithm for the rest C-1 colors individually.
– Georgi Gerganov
Dec 31 '18 at 11:24
1
Great answer (+1). By the way, when I set the slider to 4 on your demo, I only see two green rectangles - is that because I'm on a smartphone and it's subtle or because their borders are neighbouring? In the latter case, shouldn't they be considered 2 rather than 4 intervals?
– גלעד ברקן
Jan 1 at 4:05
1
No, I see now the complete line was extending beyond the screen :) I needed to pinch.
– גלעד ברקן
Jan 1 at 14:11
|
show 1 more comment
I would advise you to use Haar wavelet. That is a very simple algorithm which was often used to provide the functionality of progressive loading for big images on websites.
Here you can see how it works with 2D function. That is what you can use. Alas, the document is in Ukrainian, but code in C++, so readable:)
This document provides an example of 3D object:
Pseudocode on how to compress with Haar wavelet you can find in Wavelets for Computer Graphics: A Primer Part 1y.
1
thanks for the suggestion. Will definitely look into this
– hampusohlsson
Dec 31 '18 at 9:17
add a comment |
You could do the following:
- Write out the points that divide the whole strip into intervals as the array
[a[0], a[1], a[2], ..., a[n-1]]
. In your example, the array would be[0, 5, 6, 10, ... ]
. - Calculate double-interval lengths
a[2]-a[0], a[3]-a[1], a[4]-a[2], ..., a[n-1]-a[n-3]
and find the least of them. Let it bea[k+2]-a[k]
. If there are two or more equal lengths having the lowest value, choose one of them randomly. In your example, you should get the array[6, 5, ... ]
and search for the minimum value through it. - Swap the intervals
(a[k], a[k+1])
and(a[k+1], a[k+2])
. Basically, you need to assigna[k+1]=a[k]+a[k+2]-a[k+1]
to keep the lengths, and to remove the pointsa[k]
anda[k+2]
from the array after that because two pairs of intervals of the same color are now merged into two larger intervals. Thus, the numbers of blue and green intervals decreases by one each after this step. - If you're satisfied with the current number of intervals, end the process, otherwise go to the step 1.
You performed the step 2 in order to decrease "color shift" because, at the step 3, the left interval is moved a[k+2]-a[k+1]
to the right and the right interval is moved a[k+1]-a[k]
to the left. The sum of these distances, a[k+2]-a[k]
can be considered a measure of change you're introducing into the whole picture.
Main advantages of this approach:
- It is simple.
- It doesn't give a preference to any of the two colors. You don't need to assign one of the colors to be the background and the other to be the painting color. The picture can be considered both as "green-on-blue" and "blue-on-green". This reflects quite common use case when two colors just describe two opposite states (like the bit
0/1
, "yes/no" answer) of some process extended in time or in space. - It always keeps the balance between colors, i.e. the sum of intervals of each color remains the same during the reduction process. Thus the total brightness of the picture doesn't change. It is important as this total brightness can be considered an "indicator of completeness" at some cases.
add a comment |
Here's another attempt at dynamic programming that's slightly different than Georgi Gerganov's, although the idea to try and formulate a dynamic program may have been inspired by his answer. Neither the implementation nor the concept is guaranteed to be sound but I did include a code sketch with a visual example :)
The search space in this case is not reliant on the total unit width but rather on the number of intervals. It's O(N * n^2)
time and O(N * n)
space, where N
and n
are the target and given number of (green) intervals, respectively, because we assume that any newly chosen green interval must be bound by two green intervals (rather than extend arbitrarily into the background).
The idea also utilises the prefix sum idea used to calculate runs with a majority element. We add 1 when we see the target element (in this case green) and subtract 1 for others (that algorithm is also amenable to multiple elements with parallel prefix sum tracking). (I'm not sure that restricting candidate intervals to sections with a majority of the target colour is always warranted but it may be a useful heuristic depending on the desired outcome. It's also adjustable -- we can easily adjust it to check for a different part than 1/2.)
Where Georgi Gerganov's program seeks to minimise, this dynamic program seeks to maximise two ratios. Let h(i, k)
represent the best sequence of green intervals up to the i
th given interval, utilising k
intervals, where each is allowed to stretch back to the left edge of some previous green interval. We speculate that
h(i, k) = max(r + C*r1 + h(i-l, k-1))
where, in the current candidate interval, r
is the ratio of green to the length of the stretch, and r1
is the ratio of green to the total given green. r1
is multiplied by an adjustable constant to give more weight to the volume of green covered. l
is the length of the stretch.
JavaScript code (for debugging, it includes some extra variables and log lines):
function rnd(n, d=2){
let m = Math.pow(10,d)
return Math.round(m*n) / m;
}
function f(A, N, C){
let ps = [[0,0]];
let psBG = [0];
let totalG = 0;
A.unshift([0,0]);
for (let i=1; i<A.length; i++){
let [l,r,c] = A[i];
if (c == 'g'){
totalG += r - l;
let prevI = ps[ps.length-1][1];
let d = l - A[prevI][1];
let prevS = ps[ps.length-1][0];
ps.push(
[prevS - d, i, 'l'],
[prevS - d + r - l, i, 'r']
);
psBG[i] = psBG[i-1];
} else {
psBG[i] = psBG[i-1] + r - l;
}
}
//console.log(JSON.stringify(A));
//console.log('');
//console.log(JSON.stringify(ps));
//console.log('');
//console.log(JSON.stringify(psBG));
let m = new Array(N + 1);
m[0] = new Array((ps.length >> 1) + 1);
for (let i=0; i<m[0].length; i++)
m[0][i] = [0,0];
// for each in N
for (let i=1; i<=N; i++){
m[i] = new Array((ps.length >> 1) + 1);
for (let ii=0; ii<m[0].length; ii++)
m[i][ii] = [0,0];
// for each interval
for (let j=i; j<m[0].length; j++){
m[i][j] = m[i][j-1];
for (let k=j; k>i-1; k--){
// our anchors are the right
// side of each interval, k's are the left
let jj = 2*j;
let kk = 2*k - 1;
// positive means green
// is a majority
if (ps[jj][0] - ps[kk][0] > 0){
let bg = psBG[ps[jj][1]] - psBG[ps[kk][1]];
let s = A[ps[jj][1]][1] - A[ps[kk][1]][0] - bg;
let r = s / (bg + s);
let r1 = C * s / totalG;
let candidate = r + r1 + m[i-1][j-1][0];
if (candidate > m[i][j][0]){
m[i][j] = [
candidate,
ps[kk][1] + ',' + ps[jj][1],
bg, s, r, r1,k,m[i-1][j-1][0]
];
}
}
}
}
}
/*
for (row of m)
console.log(JSON.stringify(
row.map(l => l.map(x => typeof x != 'number' ? x : rnd(x)))));
*/
let result = new Array(N);
let j = m[0].length - 1;
for (let i=N; i>0; i--){
let [_,idxs,w,x,y,z,k] = m[i][j];
let [l,r] = idxs.split(',');
result[i-1] = [A[l][0], A[r][1], 'g'];
j = k - 1;
}
return result;
}
function show(A, last){
if (last[1] != A[A.length-1])
A.push(last);
let s = '';
let j;
for (let i=A.length-1; i>=0; i--){
let [l, r, c] = A[i];
let cc = c == 'g' ? 'X' : '.';
for (let j=r-1; j>=l; j--)
s = cc + s;
if (i > 0)
for (let j=l-1; j>=A[i-1][1]; j--)
s = '.' + s
}
for (let j=A[0][0]-1; j>=0; j--)
s = '.' + s
console.log(s);
return s;
}
function g(A, N, C){
const ts = f(A, N, C);
//console.log(JSON.stringify(ts));
show(A, A[A.length-1]);
show(ts, A[A.length-1]);
}
var a = [
[0,5,'b'],
[5,9,'g'],
[9,10,'b'],
[10,15,'g'],
[15,40,'b'],
[40,41,'g'],
[41,43,'b'],
[43,44,'g'],
[44,45,'b'],
[45,46,'g'],
[46,55,'b'],
[55,65,'g'],
[65,100,'b']
];
// (input, N, C)
g(a, 2, 2);
console.log('');
g(a, 3, 2);
console.log('');
g(a, 4, 2);
console.log('');
g(a, 4, 5);
1
"not sure that restricting candidate intervals to sections with a majority of the target colour is always warranted but it may be a useful heuristic depending on the desired outcome" - I agree it depends on the desired outcome. The current problem statement "to produce a visually similar plot" leaves room for interpretation and therefore there can be different formulations of the objective function. Also, I liked the idea to reduce the search space to a function of the number of input intervals, instead of the max width.
– Georgi Gerganov
Jan 2 at 13:50
@GeorgiGerganov cool, thanks for reading and commenting!
– גלעד ברקן
Jan 2 at 20:11
add a comment |
I would suggest using K-means it is an algorithm used to group data(a more detailed explanation here: https://en.wikipedia.org/wiki/K-means_clustering and here https://scikit-learn.org/stable/modules/generated/sklearn.cluster.KMeans.html)
this would be a brief explanation of how the function should look like, hope it is helpful.
from sklearn.cluster import KMeans
import numpy as np
def downsample(input, cluster = 25):
# you will need to group your labels in a nmpy array as shown bellow
# for the sake of example I will take just a random array
X = np.array([[1, 2], [1, 4], [1, 0],[4, 2], [4, 4], [4, 0]])
# n_clusters will be the same as desired output
kmeans = KMeans(n_clusters= cluster, random_state=0).fit(X)
# then you can iterate through labels that was assigned to every entr of your input
# in our case the interval
kmeans_list = [None]*cluster
for i in range(0, X.shape[0]):
kmeans_list[kmeans.labels_[i]].append(X[i])
# after that you will basicly have a list of lists and every inner list will contain all points that corespond to a
# specific label
ret = #return list
for label_list in kmeans_list:
left = 10001000 # a big enough number to exced anything that you will get as an input
right = -left # same here
for entry in label_list:
left = min(left, entry[0])
right = max(right, entry[1])
ret.append([left,right])
return ret
1
Using KMeans is definitely an interesting approach, I could totally see this work. Thanks for your answer @Dan Butmalai
– hampusohlsson
Dec 31 '18 at 9:18
add a comment |
Your Answer
StackExchange.ifUsing("editor", function () {
StackExchange.using("externalEditor", function () {
StackExchange.using("snippets", function () {
StackExchange.snippets.init();
});
});
}, "code-snippets");
StackExchange.ready(function() {
var channelOptions = {
tags: "".split(" "),
id: "1"
};
initTagRenderer("".split(" "), "".split(" "), channelOptions);
StackExchange.using("externalEditor", function() {
// Have to fire editor after snippets, if snippets enabled
if (StackExchange.settings.snippets.snippetsEnabled) {
StackExchange.using("snippets", function() {
createEditor();
});
}
else {
createEditor();
}
});
function createEditor() {
StackExchange.prepareEditor({
heartbeatType: 'answer',
autoActivateHeartbeat: false,
convertImagesToLinks: true,
noModals: true,
showLowRepImageUploadWarning: true,
reputationToPostImages: 10,
bindNavPrevention: true,
postfix: "",
imageUploader: {
brandingHtml: "Powered by u003ca class="icon-imgur-white" href="https://imgur.com/"u003eu003c/au003e",
contentPolicyHtml: "User contributions licensed under u003ca href="https://creativecommons.org/licenses/by-sa/3.0/"u003ecc by-sa 3.0 with attribution requiredu003c/au003e u003ca href="https://stackoverflow.com/legal/content-policy"u003e(content policy)u003c/au003e",
allowUrls: true
},
onDemand: true,
discardSelector: ".discard-answer"
,immediatelyShowMarkdownHelp:true
});
}
});
Sign up or log in
StackExchange.ready(function () {
StackExchange.helpers.onClickDraftSave('#login-link');
});
Sign up using Google
Sign up using Facebook
Sign up using Email and Password
Post as a guest
Required, but never shown
StackExchange.ready(
function () {
StackExchange.openid.initPostLogin('.new-post-login', 'https%3a%2f%2fstackoverflow.com%2fquestions%2f53978490%2falgorithm-for-downsampling-array-of-intervals%23new-answer', 'question_page');
}
);
Post as a guest
Required, but never shown
5 Answers
5
active
oldest
votes
5 Answers
5
active
oldest
votes
active
oldest
votes
active
oldest
votes
Update 1:
Below is my original post which I initially deleted, because there were issues with displaying the equations and also I wasn't very confident if it really makes sense. But later, I figured that the optimisation problem that I described can be actually solved efficiently with DP (Dynamic programming).
So I did a sample C++ implementation. Here are some results:
Here is a live demo that you can play with in your browser (make sure browser support WebGL2, like Chrome or Firefox). It takes a bit to load the page.
Here is the C++ implementation: link
Update 2:
Turns out the proposed solution has the following nice property - we can easily control the importance of the two parts F1 and F2 of the cost function. Simply change the cost function to F(α)=F1 + αF2, where α >= 1.0 is a free parameter. The DP algorithm remains the same.
Here are some result for different α values using the same number of intervals N:
Live demo (WebGL2 required)
As can be seen, higher α means it is more important to cover the original input intervals even if this means covering more of the background in-between.
Original post
Even-though some good algorithms have already been proposed, I would like to propose a slightly unusual approach - interpreting the task as an optimisation problem. Although, I don't know how to efficiently solve the optimisation problem (or even if it can be solved in reasonable time at all), it might be useful to someone purely as a concept.
First, without loss of generality, lets declare the blue color to be background. We will be painting N green intervals on top of it (N is the number provided to the downsample()
function in OP's description). The ith interval is defined by its starting coordinate 0 <= xi < xmax and width wi >= 0 (xmax is the maximum coordinate from the input).
Lets also define the array G(x) to be the number of green cells in the interval [0, x) in the input data. This array can easily be pre-calculated. We will use it to quickly calculate the number of green cells in arbitrary interval [x, y) - namely: G(y) - G(x).
We can now introduce the first part of the cost function for our optimisation problem:
The smaller F1 is, the better our generated intervals cover the input intervals, so we will be searching for xi, wi that minimise it. Ideally we want F1=0 which would mean that the intervals do not cover any of the background (which of course is not possible because N is less than the input intervals).
However, this function is not enough to describe the problem, because obviously we can minimise it by taking empty intervals: F1(x, 0)=0. Instead, we want to cover as much as possible from the input intervals. Lets introduce the second part of the cost function which corresponds to this requirement:
The smaller F2 is, the more input intervals are covered. Ideally we want F2=0 which would mean that we covered all of the input rectangles. However, minimising F2 competes with minimising F1.
Finally, we can state our optimisation problem: find xi, wi that minimize F=F1 + F2
How to solve this problem? Not sure. Maybe use some metaheuristic approach for global optimisation such as Simulated annealing or Differential evolution. These are typically easy to implement, especially for this simple cost function.
Best case would be to exist some kind of DP algorithm for solving it efficiently, but unlikely.
1
This is awesome, thanks for sharing and spending time on this @Georgi Gerganov
– hampusohlsson
Dec 31 '18 at 11:05
1
So I played around with your example - fabulous! Follow up question -- what if you introduce another color/dimension @Gregori Gerganov? Could this approach still work?
– hampusohlsson
Dec 31 '18 at 11:17
1
Sure. Again, declare one of the C >= 2 colors to be background and apply this same algorithm for the rest C-1 colors individually.
– Georgi Gerganov
Dec 31 '18 at 11:24
1
Great answer (+1). By the way, when I set the slider to 4 on your demo, I only see two green rectangles - is that because I'm on a smartphone and it's subtle or because their borders are neighbouring? In the latter case, shouldn't they be considered 2 rather than 4 intervals?
– גלעד ברקן
Jan 1 at 4:05
1
No, I see now the complete line was extending beyond the screen :) I needed to pinch.
– גלעד ברקן
Jan 1 at 14:11
|
show 1 more comment
Update 1:
Below is my original post which I initially deleted, because there were issues with displaying the equations and also I wasn't very confident if it really makes sense. But later, I figured that the optimisation problem that I described can be actually solved efficiently with DP (Dynamic programming).
So I did a sample C++ implementation. Here are some results:
Here is a live demo that you can play with in your browser (make sure browser support WebGL2, like Chrome or Firefox). It takes a bit to load the page.
Here is the C++ implementation: link
Update 2:
Turns out the proposed solution has the following nice property - we can easily control the importance of the two parts F1 and F2 of the cost function. Simply change the cost function to F(α)=F1 + αF2, where α >= 1.0 is a free parameter. The DP algorithm remains the same.
Here are some result for different α values using the same number of intervals N:
Live demo (WebGL2 required)
As can be seen, higher α means it is more important to cover the original input intervals even if this means covering more of the background in-between.
Original post
Even-though some good algorithms have already been proposed, I would like to propose a slightly unusual approach - interpreting the task as an optimisation problem. Although, I don't know how to efficiently solve the optimisation problem (or even if it can be solved in reasonable time at all), it might be useful to someone purely as a concept.
First, without loss of generality, lets declare the blue color to be background. We will be painting N green intervals on top of it (N is the number provided to the downsample()
function in OP's description). The ith interval is defined by its starting coordinate 0 <= xi < xmax and width wi >= 0 (xmax is the maximum coordinate from the input).
Lets also define the array G(x) to be the number of green cells in the interval [0, x) in the input data. This array can easily be pre-calculated. We will use it to quickly calculate the number of green cells in arbitrary interval [x, y) - namely: G(y) - G(x).
We can now introduce the first part of the cost function for our optimisation problem:
The smaller F1 is, the better our generated intervals cover the input intervals, so we will be searching for xi, wi that minimise it. Ideally we want F1=0 which would mean that the intervals do not cover any of the background (which of course is not possible because N is less than the input intervals).
However, this function is not enough to describe the problem, because obviously we can minimise it by taking empty intervals: F1(x, 0)=0. Instead, we want to cover as much as possible from the input intervals. Lets introduce the second part of the cost function which corresponds to this requirement:
The smaller F2 is, the more input intervals are covered. Ideally we want F2=0 which would mean that we covered all of the input rectangles. However, minimising F2 competes with minimising F1.
Finally, we can state our optimisation problem: find xi, wi that minimize F=F1 + F2
How to solve this problem? Not sure. Maybe use some metaheuristic approach for global optimisation such as Simulated annealing or Differential evolution. These are typically easy to implement, especially for this simple cost function.
Best case would be to exist some kind of DP algorithm for solving it efficiently, but unlikely.
1
This is awesome, thanks for sharing and spending time on this @Georgi Gerganov
– hampusohlsson
Dec 31 '18 at 11:05
1
So I played around with your example - fabulous! Follow up question -- what if you introduce another color/dimension @Gregori Gerganov? Could this approach still work?
– hampusohlsson
Dec 31 '18 at 11:17
1
Sure. Again, declare one of the C >= 2 colors to be background and apply this same algorithm for the rest C-1 colors individually.
– Georgi Gerganov
Dec 31 '18 at 11:24
1
Great answer (+1). By the way, when I set the slider to 4 on your demo, I only see two green rectangles - is that because I'm on a smartphone and it's subtle or because their borders are neighbouring? In the latter case, shouldn't they be considered 2 rather than 4 intervals?
– גלעד ברקן
Jan 1 at 4:05
1
No, I see now the complete line was extending beyond the screen :) I needed to pinch.
– גלעד ברקן
Jan 1 at 14:11
|
show 1 more comment
Update 1:
Below is my original post which I initially deleted, because there were issues with displaying the equations and also I wasn't very confident if it really makes sense. But later, I figured that the optimisation problem that I described can be actually solved efficiently with DP (Dynamic programming).
So I did a sample C++ implementation. Here are some results:
Here is a live demo that you can play with in your browser (make sure browser support WebGL2, like Chrome or Firefox). It takes a bit to load the page.
Here is the C++ implementation: link
Update 2:
Turns out the proposed solution has the following nice property - we can easily control the importance of the two parts F1 and F2 of the cost function. Simply change the cost function to F(α)=F1 + αF2, where α >= 1.0 is a free parameter. The DP algorithm remains the same.
Here are some result for different α values using the same number of intervals N:
Live demo (WebGL2 required)
As can be seen, higher α means it is more important to cover the original input intervals even if this means covering more of the background in-between.
Original post
Even-though some good algorithms have already been proposed, I would like to propose a slightly unusual approach - interpreting the task as an optimisation problem. Although, I don't know how to efficiently solve the optimisation problem (or even if it can be solved in reasonable time at all), it might be useful to someone purely as a concept.
First, without loss of generality, lets declare the blue color to be background. We will be painting N green intervals on top of it (N is the number provided to the downsample()
function in OP's description). The ith interval is defined by its starting coordinate 0 <= xi < xmax and width wi >= 0 (xmax is the maximum coordinate from the input).
Lets also define the array G(x) to be the number of green cells in the interval [0, x) in the input data. This array can easily be pre-calculated. We will use it to quickly calculate the number of green cells in arbitrary interval [x, y) - namely: G(y) - G(x).
We can now introduce the first part of the cost function for our optimisation problem:
The smaller F1 is, the better our generated intervals cover the input intervals, so we will be searching for xi, wi that minimise it. Ideally we want F1=0 which would mean that the intervals do not cover any of the background (which of course is not possible because N is less than the input intervals).
However, this function is not enough to describe the problem, because obviously we can minimise it by taking empty intervals: F1(x, 0)=0. Instead, we want to cover as much as possible from the input intervals. Lets introduce the second part of the cost function which corresponds to this requirement:
The smaller F2 is, the more input intervals are covered. Ideally we want F2=0 which would mean that we covered all of the input rectangles. However, minimising F2 competes with minimising F1.
Finally, we can state our optimisation problem: find xi, wi that minimize F=F1 + F2
How to solve this problem? Not sure. Maybe use some metaheuristic approach for global optimisation such as Simulated annealing or Differential evolution. These are typically easy to implement, especially for this simple cost function.
Best case would be to exist some kind of DP algorithm for solving it efficiently, but unlikely.
Update 1:
Below is my original post which I initially deleted, because there were issues with displaying the equations and also I wasn't very confident if it really makes sense. But later, I figured that the optimisation problem that I described can be actually solved efficiently with DP (Dynamic programming).
So I did a sample C++ implementation. Here are some results:
Here is a live demo that you can play with in your browser (make sure browser support WebGL2, like Chrome or Firefox). It takes a bit to load the page.
Here is the C++ implementation: link
Update 2:
Turns out the proposed solution has the following nice property - we can easily control the importance of the two parts F1 and F2 of the cost function. Simply change the cost function to F(α)=F1 + αF2, where α >= 1.0 is a free parameter. The DP algorithm remains the same.
Here are some result for different α values using the same number of intervals N:
Live demo (WebGL2 required)
As can be seen, higher α means it is more important to cover the original input intervals even if this means covering more of the background in-between.
Original post
Even-though some good algorithms have already been proposed, I would like to propose a slightly unusual approach - interpreting the task as an optimisation problem. Although, I don't know how to efficiently solve the optimisation problem (or even if it can be solved in reasonable time at all), it might be useful to someone purely as a concept.
First, without loss of generality, lets declare the blue color to be background. We will be painting N green intervals on top of it (N is the number provided to the downsample()
function in OP's description). The ith interval is defined by its starting coordinate 0 <= xi < xmax and width wi >= 0 (xmax is the maximum coordinate from the input).
Lets also define the array G(x) to be the number of green cells in the interval [0, x) in the input data. This array can easily be pre-calculated. We will use it to quickly calculate the number of green cells in arbitrary interval [x, y) - namely: G(y) - G(x).
We can now introduce the first part of the cost function for our optimisation problem:
The smaller F1 is, the better our generated intervals cover the input intervals, so we will be searching for xi, wi that minimise it. Ideally we want F1=0 which would mean that the intervals do not cover any of the background (which of course is not possible because N is less than the input intervals).
However, this function is not enough to describe the problem, because obviously we can minimise it by taking empty intervals: F1(x, 0)=0. Instead, we want to cover as much as possible from the input intervals. Lets introduce the second part of the cost function which corresponds to this requirement:
The smaller F2 is, the more input intervals are covered. Ideally we want F2=0 which would mean that we covered all of the input rectangles. However, minimising F2 competes with minimising F1.
Finally, we can state our optimisation problem: find xi, wi that minimize F=F1 + F2
How to solve this problem? Not sure. Maybe use some metaheuristic approach for global optimisation such as Simulated annealing or Differential evolution. These are typically easy to implement, especially for this simple cost function.
Best case would be to exist some kind of DP algorithm for solving it efficiently, but unlikely.
edited Jan 1 at 12:11
answered Dec 30 '18 at 22:00


Georgi GerganovGeorgi Gerganov
761611
761611
1
This is awesome, thanks for sharing and spending time on this @Georgi Gerganov
– hampusohlsson
Dec 31 '18 at 11:05
1
So I played around with your example - fabulous! Follow up question -- what if you introduce another color/dimension @Gregori Gerganov? Could this approach still work?
– hampusohlsson
Dec 31 '18 at 11:17
1
Sure. Again, declare one of the C >= 2 colors to be background and apply this same algorithm for the rest C-1 colors individually.
– Georgi Gerganov
Dec 31 '18 at 11:24
1
Great answer (+1). By the way, when I set the slider to 4 on your demo, I only see two green rectangles - is that because I'm on a smartphone and it's subtle or because their borders are neighbouring? In the latter case, shouldn't they be considered 2 rather than 4 intervals?
– גלעד ברקן
Jan 1 at 4:05
1
No, I see now the complete line was extending beyond the screen :) I needed to pinch.
– גלעד ברקן
Jan 1 at 14:11
|
show 1 more comment
1
This is awesome, thanks for sharing and spending time on this @Georgi Gerganov
– hampusohlsson
Dec 31 '18 at 11:05
1
So I played around with your example - fabulous! Follow up question -- what if you introduce another color/dimension @Gregori Gerganov? Could this approach still work?
– hampusohlsson
Dec 31 '18 at 11:17
1
Sure. Again, declare one of the C >= 2 colors to be background and apply this same algorithm for the rest C-1 colors individually.
– Georgi Gerganov
Dec 31 '18 at 11:24
1
Great answer (+1). By the way, when I set the slider to 4 on your demo, I only see two green rectangles - is that because I'm on a smartphone and it's subtle or because their borders are neighbouring? In the latter case, shouldn't they be considered 2 rather than 4 intervals?
– גלעד ברקן
Jan 1 at 4:05
1
No, I see now the complete line was extending beyond the screen :) I needed to pinch.
– גלעד ברקן
Jan 1 at 14:11
1
1
This is awesome, thanks for sharing and spending time on this @Georgi Gerganov
– hampusohlsson
Dec 31 '18 at 11:05
This is awesome, thanks for sharing and spending time on this @Georgi Gerganov
– hampusohlsson
Dec 31 '18 at 11:05
1
1
So I played around with your example - fabulous! Follow up question -- what if you introduce another color/dimension @Gregori Gerganov? Could this approach still work?
– hampusohlsson
Dec 31 '18 at 11:17
So I played around with your example - fabulous! Follow up question -- what if you introduce another color/dimension @Gregori Gerganov? Could this approach still work?
– hampusohlsson
Dec 31 '18 at 11:17
1
1
Sure. Again, declare one of the C >= 2 colors to be background and apply this same algorithm for the rest C-1 colors individually.
– Georgi Gerganov
Dec 31 '18 at 11:24
Sure. Again, declare one of the C >= 2 colors to be background and apply this same algorithm for the rest C-1 colors individually.
– Georgi Gerganov
Dec 31 '18 at 11:24
1
1
Great answer (+1). By the way, when I set the slider to 4 on your demo, I only see two green rectangles - is that because I'm on a smartphone and it's subtle or because their borders are neighbouring? In the latter case, shouldn't they be considered 2 rather than 4 intervals?
– גלעד ברקן
Jan 1 at 4:05
Great answer (+1). By the way, when I set the slider to 4 on your demo, I only see two green rectangles - is that because I'm on a smartphone and it's subtle or because their borders are neighbouring? In the latter case, shouldn't they be considered 2 rather than 4 intervals?
– גלעד ברקן
Jan 1 at 4:05
1
1
No, I see now the complete line was extending beyond the screen :) I needed to pinch.
– גלעד ברקן
Jan 1 at 14:11
No, I see now the complete line was extending beyond the screen :) I needed to pinch.
– גלעד ברקן
Jan 1 at 14:11
|
show 1 more comment
I would advise you to use Haar wavelet. That is a very simple algorithm which was often used to provide the functionality of progressive loading for big images on websites.
Here you can see how it works with 2D function. That is what you can use. Alas, the document is in Ukrainian, but code in C++, so readable:)
This document provides an example of 3D object:
Pseudocode on how to compress with Haar wavelet you can find in Wavelets for Computer Graphics: A Primer Part 1y.
1
thanks for the suggestion. Will definitely look into this
– hampusohlsson
Dec 31 '18 at 9:17
add a comment |
I would advise you to use Haar wavelet. That is a very simple algorithm which was often used to provide the functionality of progressive loading for big images on websites.
Here you can see how it works with 2D function. That is what you can use. Alas, the document is in Ukrainian, but code in C++, so readable:)
This document provides an example of 3D object:
Pseudocode on how to compress with Haar wavelet you can find in Wavelets for Computer Graphics: A Primer Part 1y.
1
thanks for the suggestion. Will definitely look into this
– hampusohlsson
Dec 31 '18 at 9:17
add a comment |
I would advise you to use Haar wavelet. That is a very simple algorithm which was often used to provide the functionality of progressive loading for big images on websites.
Here you can see how it works with 2D function. That is what you can use. Alas, the document is in Ukrainian, but code in C++, so readable:)
This document provides an example of 3D object:
Pseudocode on how to compress with Haar wavelet you can find in Wavelets for Computer Graphics: A Primer Part 1y.
I would advise you to use Haar wavelet. That is a very simple algorithm which was often used to provide the functionality of progressive loading for big images on websites.
Here you can see how it works with 2D function. That is what you can use. Alas, the document is in Ukrainian, but code in C++, so readable:)
This document provides an example of 3D object:
Pseudocode on how to compress with Haar wavelet you can find in Wavelets for Computer Graphics: A Primer Part 1y.
edited Dec 30 '18 at 19:39
answered Dec 30 '18 at 19:33
YolaYola
11.1k64672
11.1k64672
1
thanks for the suggestion. Will definitely look into this
– hampusohlsson
Dec 31 '18 at 9:17
add a comment |
1
thanks for the suggestion. Will definitely look into this
– hampusohlsson
Dec 31 '18 at 9:17
1
1
thanks for the suggestion. Will definitely look into this
– hampusohlsson
Dec 31 '18 at 9:17
thanks for the suggestion. Will definitely look into this
– hampusohlsson
Dec 31 '18 at 9:17
add a comment |
You could do the following:
- Write out the points that divide the whole strip into intervals as the array
[a[0], a[1], a[2], ..., a[n-1]]
. In your example, the array would be[0, 5, 6, 10, ... ]
. - Calculate double-interval lengths
a[2]-a[0], a[3]-a[1], a[4]-a[2], ..., a[n-1]-a[n-3]
and find the least of them. Let it bea[k+2]-a[k]
. If there are two or more equal lengths having the lowest value, choose one of them randomly. In your example, you should get the array[6, 5, ... ]
and search for the minimum value through it. - Swap the intervals
(a[k], a[k+1])
and(a[k+1], a[k+2])
. Basically, you need to assigna[k+1]=a[k]+a[k+2]-a[k+1]
to keep the lengths, and to remove the pointsa[k]
anda[k+2]
from the array after that because two pairs of intervals of the same color are now merged into two larger intervals. Thus, the numbers of blue and green intervals decreases by one each after this step. - If you're satisfied with the current number of intervals, end the process, otherwise go to the step 1.
You performed the step 2 in order to decrease "color shift" because, at the step 3, the left interval is moved a[k+2]-a[k+1]
to the right and the right interval is moved a[k+1]-a[k]
to the left. The sum of these distances, a[k+2]-a[k]
can be considered a measure of change you're introducing into the whole picture.
Main advantages of this approach:
- It is simple.
- It doesn't give a preference to any of the two colors. You don't need to assign one of the colors to be the background and the other to be the painting color. The picture can be considered both as "green-on-blue" and "blue-on-green". This reflects quite common use case when two colors just describe two opposite states (like the bit
0/1
, "yes/no" answer) of some process extended in time or in space. - It always keeps the balance between colors, i.e. the sum of intervals of each color remains the same during the reduction process. Thus the total brightness of the picture doesn't change. It is important as this total brightness can be considered an "indicator of completeness" at some cases.
add a comment |
You could do the following:
- Write out the points that divide the whole strip into intervals as the array
[a[0], a[1], a[2], ..., a[n-1]]
. In your example, the array would be[0, 5, 6, 10, ... ]
. - Calculate double-interval lengths
a[2]-a[0], a[3]-a[1], a[4]-a[2], ..., a[n-1]-a[n-3]
and find the least of them. Let it bea[k+2]-a[k]
. If there are two or more equal lengths having the lowest value, choose one of them randomly. In your example, you should get the array[6, 5, ... ]
and search for the minimum value through it. - Swap the intervals
(a[k], a[k+1])
and(a[k+1], a[k+2])
. Basically, you need to assigna[k+1]=a[k]+a[k+2]-a[k+1]
to keep the lengths, and to remove the pointsa[k]
anda[k+2]
from the array after that because two pairs of intervals of the same color are now merged into two larger intervals. Thus, the numbers of blue and green intervals decreases by one each after this step. - If you're satisfied with the current number of intervals, end the process, otherwise go to the step 1.
You performed the step 2 in order to decrease "color shift" because, at the step 3, the left interval is moved a[k+2]-a[k+1]
to the right and the right interval is moved a[k+1]-a[k]
to the left. The sum of these distances, a[k+2]-a[k]
can be considered a measure of change you're introducing into the whole picture.
Main advantages of this approach:
- It is simple.
- It doesn't give a preference to any of the two colors. You don't need to assign one of the colors to be the background and the other to be the painting color. The picture can be considered both as "green-on-blue" and "blue-on-green". This reflects quite common use case when two colors just describe two opposite states (like the bit
0/1
, "yes/no" answer) of some process extended in time or in space. - It always keeps the balance between colors, i.e. the sum of intervals of each color remains the same during the reduction process. Thus the total brightness of the picture doesn't change. It is important as this total brightness can be considered an "indicator of completeness" at some cases.
add a comment |
You could do the following:
- Write out the points that divide the whole strip into intervals as the array
[a[0], a[1], a[2], ..., a[n-1]]
. In your example, the array would be[0, 5, 6, 10, ... ]
. - Calculate double-interval lengths
a[2]-a[0], a[3]-a[1], a[4]-a[2], ..., a[n-1]-a[n-3]
and find the least of them. Let it bea[k+2]-a[k]
. If there are two or more equal lengths having the lowest value, choose one of them randomly. In your example, you should get the array[6, 5, ... ]
and search for the minimum value through it. - Swap the intervals
(a[k], a[k+1])
and(a[k+1], a[k+2])
. Basically, you need to assigna[k+1]=a[k]+a[k+2]-a[k+1]
to keep the lengths, and to remove the pointsa[k]
anda[k+2]
from the array after that because two pairs of intervals of the same color are now merged into two larger intervals. Thus, the numbers of blue and green intervals decreases by one each after this step. - If you're satisfied with the current number of intervals, end the process, otherwise go to the step 1.
You performed the step 2 in order to decrease "color shift" because, at the step 3, the left interval is moved a[k+2]-a[k+1]
to the right and the right interval is moved a[k+1]-a[k]
to the left. The sum of these distances, a[k+2]-a[k]
can be considered a measure of change you're introducing into the whole picture.
Main advantages of this approach:
- It is simple.
- It doesn't give a preference to any of the two colors. You don't need to assign one of the colors to be the background and the other to be the painting color. The picture can be considered both as "green-on-blue" and "blue-on-green". This reflects quite common use case when two colors just describe two opposite states (like the bit
0/1
, "yes/no" answer) of some process extended in time or in space. - It always keeps the balance between colors, i.e. the sum of intervals of each color remains the same during the reduction process. Thus the total brightness of the picture doesn't change. It is important as this total brightness can be considered an "indicator of completeness" at some cases.
You could do the following:
- Write out the points that divide the whole strip into intervals as the array
[a[0], a[1], a[2], ..., a[n-1]]
. In your example, the array would be[0, 5, 6, 10, ... ]
. - Calculate double-interval lengths
a[2]-a[0], a[3]-a[1], a[4]-a[2], ..., a[n-1]-a[n-3]
and find the least of them. Let it bea[k+2]-a[k]
. If there are two or more equal lengths having the lowest value, choose one of them randomly. In your example, you should get the array[6, 5, ... ]
and search for the minimum value through it. - Swap the intervals
(a[k], a[k+1])
and(a[k+1], a[k+2])
. Basically, you need to assigna[k+1]=a[k]+a[k+2]-a[k+1]
to keep the lengths, and to remove the pointsa[k]
anda[k+2]
from the array after that because two pairs of intervals of the same color are now merged into two larger intervals. Thus, the numbers of blue and green intervals decreases by one each after this step. - If you're satisfied with the current number of intervals, end the process, otherwise go to the step 1.
You performed the step 2 in order to decrease "color shift" because, at the step 3, the left interval is moved a[k+2]-a[k+1]
to the right and the right interval is moved a[k+1]-a[k]
to the left. The sum of these distances, a[k+2]-a[k]
can be considered a measure of change you're introducing into the whole picture.
Main advantages of this approach:
- It is simple.
- It doesn't give a preference to any of the two colors. You don't need to assign one of the colors to be the background and the other to be the painting color. The picture can be considered both as "green-on-blue" and "blue-on-green". This reflects quite common use case when two colors just describe two opposite states (like the bit
0/1
, "yes/no" answer) of some process extended in time or in space. - It always keeps the balance between colors, i.e. the sum of intervals of each color remains the same during the reduction process. Thus the total brightness of the picture doesn't change. It is important as this total brightness can be considered an "indicator of completeness" at some cases.
edited Dec 31 '18 at 15:07
answered Dec 30 '18 at 19:17


John McClaneJohn McClane
1,5502419
1,5502419
add a comment |
add a comment |
Here's another attempt at dynamic programming that's slightly different than Georgi Gerganov's, although the idea to try and formulate a dynamic program may have been inspired by his answer. Neither the implementation nor the concept is guaranteed to be sound but I did include a code sketch with a visual example :)
The search space in this case is not reliant on the total unit width but rather on the number of intervals. It's O(N * n^2)
time and O(N * n)
space, where N
and n
are the target and given number of (green) intervals, respectively, because we assume that any newly chosen green interval must be bound by two green intervals (rather than extend arbitrarily into the background).
The idea also utilises the prefix sum idea used to calculate runs with a majority element. We add 1 when we see the target element (in this case green) and subtract 1 for others (that algorithm is also amenable to multiple elements with parallel prefix sum tracking). (I'm not sure that restricting candidate intervals to sections with a majority of the target colour is always warranted but it may be a useful heuristic depending on the desired outcome. It's also adjustable -- we can easily adjust it to check for a different part than 1/2.)
Where Georgi Gerganov's program seeks to minimise, this dynamic program seeks to maximise two ratios. Let h(i, k)
represent the best sequence of green intervals up to the i
th given interval, utilising k
intervals, where each is allowed to stretch back to the left edge of some previous green interval. We speculate that
h(i, k) = max(r + C*r1 + h(i-l, k-1))
where, in the current candidate interval, r
is the ratio of green to the length of the stretch, and r1
is the ratio of green to the total given green. r1
is multiplied by an adjustable constant to give more weight to the volume of green covered. l
is the length of the stretch.
JavaScript code (for debugging, it includes some extra variables and log lines):
function rnd(n, d=2){
let m = Math.pow(10,d)
return Math.round(m*n) / m;
}
function f(A, N, C){
let ps = [[0,0]];
let psBG = [0];
let totalG = 0;
A.unshift([0,0]);
for (let i=1; i<A.length; i++){
let [l,r,c] = A[i];
if (c == 'g'){
totalG += r - l;
let prevI = ps[ps.length-1][1];
let d = l - A[prevI][1];
let prevS = ps[ps.length-1][0];
ps.push(
[prevS - d, i, 'l'],
[prevS - d + r - l, i, 'r']
);
psBG[i] = psBG[i-1];
} else {
psBG[i] = psBG[i-1] + r - l;
}
}
//console.log(JSON.stringify(A));
//console.log('');
//console.log(JSON.stringify(ps));
//console.log('');
//console.log(JSON.stringify(psBG));
let m = new Array(N + 1);
m[0] = new Array((ps.length >> 1) + 1);
for (let i=0; i<m[0].length; i++)
m[0][i] = [0,0];
// for each in N
for (let i=1; i<=N; i++){
m[i] = new Array((ps.length >> 1) + 1);
for (let ii=0; ii<m[0].length; ii++)
m[i][ii] = [0,0];
// for each interval
for (let j=i; j<m[0].length; j++){
m[i][j] = m[i][j-1];
for (let k=j; k>i-1; k--){
// our anchors are the right
// side of each interval, k's are the left
let jj = 2*j;
let kk = 2*k - 1;
// positive means green
// is a majority
if (ps[jj][0] - ps[kk][0] > 0){
let bg = psBG[ps[jj][1]] - psBG[ps[kk][1]];
let s = A[ps[jj][1]][1] - A[ps[kk][1]][0] - bg;
let r = s / (bg + s);
let r1 = C * s / totalG;
let candidate = r + r1 + m[i-1][j-1][0];
if (candidate > m[i][j][0]){
m[i][j] = [
candidate,
ps[kk][1] + ',' + ps[jj][1],
bg, s, r, r1,k,m[i-1][j-1][0]
];
}
}
}
}
}
/*
for (row of m)
console.log(JSON.stringify(
row.map(l => l.map(x => typeof x != 'number' ? x : rnd(x)))));
*/
let result = new Array(N);
let j = m[0].length - 1;
for (let i=N; i>0; i--){
let [_,idxs,w,x,y,z,k] = m[i][j];
let [l,r] = idxs.split(',');
result[i-1] = [A[l][0], A[r][1], 'g'];
j = k - 1;
}
return result;
}
function show(A, last){
if (last[1] != A[A.length-1])
A.push(last);
let s = '';
let j;
for (let i=A.length-1; i>=0; i--){
let [l, r, c] = A[i];
let cc = c == 'g' ? 'X' : '.';
for (let j=r-1; j>=l; j--)
s = cc + s;
if (i > 0)
for (let j=l-1; j>=A[i-1][1]; j--)
s = '.' + s
}
for (let j=A[0][0]-1; j>=0; j--)
s = '.' + s
console.log(s);
return s;
}
function g(A, N, C){
const ts = f(A, N, C);
//console.log(JSON.stringify(ts));
show(A, A[A.length-1]);
show(ts, A[A.length-1]);
}
var a = [
[0,5,'b'],
[5,9,'g'],
[9,10,'b'],
[10,15,'g'],
[15,40,'b'],
[40,41,'g'],
[41,43,'b'],
[43,44,'g'],
[44,45,'b'],
[45,46,'g'],
[46,55,'b'],
[55,65,'g'],
[65,100,'b']
];
// (input, N, C)
g(a, 2, 2);
console.log('');
g(a, 3, 2);
console.log('');
g(a, 4, 2);
console.log('');
g(a, 4, 5);
1
"not sure that restricting candidate intervals to sections with a majority of the target colour is always warranted but it may be a useful heuristic depending on the desired outcome" - I agree it depends on the desired outcome. The current problem statement "to produce a visually similar plot" leaves room for interpretation and therefore there can be different formulations of the objective function. Also, I liked the idea to reduce the search space to a function of the number of input intervals, instead of the max width.
– Georgi Gerganov
Jan 2 at 13:50
@GeorgiGerganov cool, thanks for reading and commenting!
– גלעד ברקן
Jan 2 at 20:11
add a comment |
Here's another attempt at dynamic programming that's slightly different than Georgi Gerganov's, although the idea to try and formulate a dynamic program may have been inspired by his answer. Neither the implementation nor the concept is guaranteed to be sound but I did include a code sketch with a visual example :)
The search space in this case is not reliant on the total unit width but rather on the number of intervals. It's O(N * n^2)
time and O(N * n)
space, where N
and n
are the target and given number of (green) intervals, respectively, because we assume that any newly chosen green interval must be bound by two green intervals (rather than extend arbitrarily into the background).
The idea also utilises the prefix sum idea used to calculate runs with a majority element. We add 1 when we see the target element (in this case green) and subtract 1 for others (that algorithm is also amenable to multiple elements with parallel prefix sum tracking). (I'm not sure that restricting candidate intervals to sections with a majority of the target colour is always warranted but it may be a useful heuristic depending on the desired outcome. It's also adjustable -- we can easily adjust it to check for a different part than 1/2.)
Where Georgi Gerganov's program seeks to minimise, this dynamic program seeks to maximise two ratios. Let h(i, k)
represent the best sequence of green intervals up to the i
th given interval, utilising k
intervals, where each is allowed to stretch back to the left edge of some previous green interval. We speculate that
h(i, k) = max(r + C*r1 + h(i-l, k-1))
where, in the current candidate interval, r
is the ratio of green to the length of the stretch, and r1
is the ratio of green to the total given green. r1
is multiplied by an adjustable constant to give more weight to the volume of green covered. l
is the length of the stretch.
JavaScript code (for debugging, it includes some extra variables and log lines):
function rnd(n, d=2){
let m = Math.pow(10,d)
return Math.round(m*n) / m;
}
function f(A, N, C){
let ps = [[0,0]];
let psBG = [0];
let totalG = 0;
A.unshift([0,0]);
for (let i=1; i<A.length; i++){
let [l,r,c] = A[i];
if (c == 'g'){
totalG += r - l;
let prevI = ps[ps.length-1][1];
let d = l - A[prevI][1];
let prevS = ps[ps.length-1][0];
ps.push(
[prevS - d, i, 'l'],
[prevS - d + r - l, i, 'r']
);
psBG[i] = psBG[i-1];
} else {
psBG[i] = psBG[i-1] + r - l;
}
}
//console.log(JSON.stringify(A));
//console.log('');
//console.log(JSON.stringify(ps));
//console.log('');
//console.log(JSON.stringify(psBG));
let m = new Array(N + 1);
m[0] = new Array((ps.length >> 1) + 1);
for (let i=0; i<m[0].length; i++)
m[0][i] = [0,0];
// for each in N
for (let i=1; i<=N; i++){
m[i] = new Array((ps.length >> 1) + 1);
for (let ii=0; ii<m[0].length; ii++)
m[i][ii] = [0,0];
// for each interval
for (let j=i; j<m[0].length; j++){
m[i][j] = m[i][j-1];
for (let k=j; k>i-1; k--){
// our anchors are the right
// side of each interval, k's are the left
let jj = 2*j;
let kk = 2*k - 1;
// positive means green
// is a majority
if (ps[jj][0] - ps[kk][0] > 0){
let bg = psBG[ps[jj][1]] - psBG[ps[kk][1]];
let s = A[ps[jj][1]][1] - A[ps[kk][1]][0] - bg;
let r = s / (bg + s);
let r1 = C * s / totalG;
let candidate = r + r1 + m[i-1][j-1][0];
if (candidate > m[i][j][0]){
m[i][j] = [
candidate,
ps[kk][1] + ',' + ps[jj][1],
bg, s, r, r1,k,m[i-1][j-1][0]
];
}
}
}
}
}
/*
for (row of m)
console.log(JSON.stringify(
row.map(l => l.map(x => typeof x != 'number' ? x : rnd(x)))));
*/
let result = new Array(N);
let j = m[0].length - 1;
for (let i=N; i>0; i--){
let [_,idxs,w,x,y,z,k] = m[i][j];
let [l,r] = idxs.split(',');
result[i-1] = [A[l][0], A[r][1], 'g'];
j = k - 1;
}
return result;
}
function show(A, last){
if (last[1] != A[A.length-1])
A.push(last);
let s = '';
let j;
for (let i=A.length-1; i>=0; i--){
let [l, r, c] = A[i];
let cc = c == 'g' ? 'X' : '.';
for (let j=r-1; j>=l; j--)
s = cc + s;
if (i > 0)
for (let j=l-1; j>=A[i-1][1]; j--)
s = '.' + s
}
for (let j=A[0][0]-1; j>=0; j--)
s = '.' + s
console.log(s);
return s;
}
function g(A, N, C){
const ts = f(A, N, C);
//console.log(JSON.stringify(ts));
show(A, A[A.length-1]);
show(ts, A[A.length-1]);
}
var a = [
[0,5,'b'],
[5,9,'g'],
[9,10,'b'],
[10,15,'g'],
[15,40,'b'],
[40,41,'g'],
[41,43,'b'],
[43,44,'g'],
[44,45,'b'],
[45,46,'g'],
[46,55,'b'],
[55,65,'g'],
[65,100,'b']
];
// (input, N, C)
g(a, 2, 2);
console.log('');
g(a, 3, 2);
console.log('');
g(a, 4, 2);
console.log('');
g(a, 4, 5);
1
"not sure that restricting candidate intervals to sections with a majority of the target colour is always warranted but it may be a useful heuristic depending on the desired outcome" - I agree it depends on the desired outcome. The current problem statement "to produce a visually similar plot" leaves room for interpretation and therefore there can be different formulations of the objective function. Also, I liked the idea to reduce the search space to a function of the number of input intervals, instead of the max width.
– Georgi Gerganov
Jan 2 at 13:50
@GeorgiGerganov cool, thanks for reading and commenting!
– גלעד ברקן
Jan 2 at 20:11
add a comment |
Here's another attempt at dynamic programming that's slightly different than Georgi Gerganov's, although the idea to try and formulate a dynamic program may have been inspired by his answer. Neither the implementation nor the concept is guaranteed to be sound but I did include a code sketch with a visual example :)
The search space in this case is not reliant on the total unit width but rather on the number of intervals. It's O(N * n^2)
time and O(N * n)
space, where N
and n
are the target and given number of (green) intervals, respectively, because we assume that any newly chosen green interval must be bound by two green intervals (rather than extend arbitrarily into the background).
The idea also utilises the prefix sum idea used to calculate runs with a majority element. We add 1 when we see the target element (in this case green) and subtract 1 for others (that algorithm is also amenable to multiple elements with parallel prefix sum tracking). (I'm not sure that restricting candidate intervals to sections with a majority of the target colour is always warranted but it may be a useful heuristic depending on the desired outcome. It's also adjustable -- we can easily adjust it to check for a different part than 1/2.)
Where Georgi Gerganov's program seeks to minimise, this dynamic program seeks to maximise two ratios. Let h(i, k)
represent the best sequence of green intervals up to the i
th given interval, utilising k
intervals, where each is allowed to stretch back to the left edge of some previous green interval. We speculate that
h(i, k) = max(r + C*r1 + h(i-l, k-1))
where, in the current candidate interval, r
is the ratio of green to the length of the stretch, and r1
is the ratio of green to the total given green. r1
is multiplied by an adjustable constant to give more weight to the volume of green covered. l
is the length of the stretch.
JavaScript code (for debugging, it includes some extra variables and log lines):
function rnd(n, d=2){
let m = Math.pow(10,d)
return Math.round(m*n) / m;
}
function f(A, N, C){
let ps = [[0,0]];
let psBG = [0];
let totalG = 0;
A.unshift([0,0]);
for (let i=1; i<A.length; i++){
let [l,r,c] = A[i];
if (c == 'g'){
totalG += r - l;
let prevI = ps[ps.length-1][1];
let d = l - A[prevI][1];
let prevS = ps[ps.length-1][0];
ps.push(
[prevS - d, i, 'l'],
[prevS - d + r - l, i, 'r']
);
psBG[i] = psBG[i-1];
} else {
psBG[i] = psBG[i-1] + r - l;
}
}
//console.log(JSON.stringify(A));
//console.log('');
//console.log(JSON.stringify(ps));
//console.log('');
//console.log(JSON.stringify(psBG));
let m = new Array(N + 1);
m[0] = new Array((ps.length >> 1) + 1);
for (let i=0; i<m[0].length; i++)
m[0][i] = [0,0];
// for each in N
for (let i=1; i<=N; i++){
m[i] = new Array((ps.length >> 1) + 1);
for (let ii=0; ii<m[0].length; ii++)
m[i][ii] = [0,0];
// for each interval
for (let j=i; j<m[0].length; j++){
m[i][j] = m[i][j-1];
for (let k=j; k>i-1; k--){
// our anchors are the right
// side of each interval, k's are the left
let jj = 2*j;
let kk = 2*k - 1;
// positive means green
// is a majority
if (ps[jj][0] - ps[kk][0] > 0){
let bg = psBG[ps[jj][1]] - psBG[ps[kk][1]];
let s = A[ps[jj][1]][1] - A[ps[kk][1]][0] - bg;
let r = s / (bg + s);
let r1 = C * s / totalG;
let candidate = r + r1 + m[i-1][j-1][0];
if (candidate > m[i][j][0]){
m[i][j] = [
candidate,
ps[kk][1] + ',' + ps[jj][1],
bg, s, r, r1,k,m[i-1][j-1][0]
];
}
}
}
}
}
/*
for (row of m)
console.log(JSON.stringify(
row.map(l => l.map(x => typeof x != 'number' ? x : rnd(x)))));
*/
let result = new Array(N);
let j = m[0].length - 1;
for (let i=N; i>0; i--){
let [_,idxs,w,x,y,z,k] = m[i][j];
let [l,r] = idxs.split(',');
result[i-1] = [A[l][0], A[r][1], 'g'];
j = k - 1;
}
return result;
}
function show(A, last){
if (last[1] != A[A.length-1])
A.push(last);
let s = '';
let j;
for (let i=A.length-1; i>=0; i--){
let [l, r, c] = A[i];
let cc = c == 'g' ? 'X' : '.';
for (let j=r-1; j>=l; j--)
s = cc + s;
if (i > 0)
for (let j=l-1; j>=A[i-1][1]; j--)
s = '.' + s
}
for (let j=A[0][0]-1; j>=0; j--)
s = '.' + s
console.log(s);
return s;
}
function g(A, N, C){
const ts = f(A, N, C);
//console.log(JSON.stringify(ts));
show(A, A[A.length-1]);
show(ts, A[A.length-1]);
}
var a = [
[0,5,'b'],
[5,9,'g'],
[9,10,'b'],
[10,15,'g'],
[15,40,'b'],
[40,41,'g'],
[41,43,'b'],
[43,44,'g'],
[44,45,'b'],
[45,46,'g'],
[46,55,'b'],
[55,65,'g'],
[65,100,'b']
];
// (input, N, C)
g(a, 2, 2);
console.log('');
g(a, 3, 2);
console.log('');
g(a, 4, 2);
console.log('');
g(a, 4, 5);
Here's another attempt at dynamic programming that's slightly different than Georgi Gerganov's, although the idea to try and formulate a dynamic program may have been inspired by his answer. Neither the implementation nor the concept is guaranteed to be sound but I did include a code sketch with a visual example :)
The search space in this case is not reliant on the total unit width but rather on the number of intervals. It's O(N * n^2)
time and O(N * n)
space, where N
and n
are the target and given number of (green) intervals, respectively, because we assume that any newly chosen green interval must be bound by two green intervals (rather than extend arbitrarily into the background).
The idea also utilises the prefix sum idea used to calculate runs with a majority element. We add 1 when we see the target element (in this case green) and subtract 1 for others (that algorithm is also amenable to multiple elements with parallel prefix sum tracking). (I'm not sure that restricting candidate intervals to sections with a majority of the target colour is always warranted but it may be a useful heuristic depending on the desired outcome. It's also adjustable -- we can easily adjust it to check for a different part than 1/2.)
Where Georgi Gerganov's program seeks to minimise, this dynamic program seeks to maximise two ratios. Let h(i, k)
represent the best sequence of green intervals up to the i
th given interval, utilising k
intervals, where each is allowed to stretch back to the left edge of some previous green interval. We speculate that
h(i, k) = max(r + C*r1 + h(i-l, k-1))
where, in the current candidate interval, r
is the ratio of green to the length of the stretch, and r1
is the ratio of green to the total given green. r1
is multiplied by an adjustable constant to give more weight to the volume of green covered. l
is the length of the stretch.
JavaScript code (for debugging, it includes some extra variables and log lines):
function rnd(n, d=2){
let m = Math.pow(10,d)
return Math.round(m*n) / m;
}
function f(A, N, C){
let ps = [[0,0]];
let psBG = [0];
let totalG = 0;
A.unshift([0,0]);
for (let i=1; i<A.length; i++){
let [l,r,c] = A[i];
if (c == 'g'){
totalG += r - l;
let prevI = ps[ps.length-1][1];
let d = l - A[prevI][1];
let prevS = ps[ps.length-1][0];
ps.push(
[prevS - d, i, 'l'],
[prevS - d + r - l, i, 'r']
);
psBG[i] = psBG[i-1];
} else {
psBG[i] = psBG[i-1] + r - l;
}
}
//console.log(JSON.stringify(A));
//console.log('');
//console.log(JSON.stringify(ps));
//console.log('');
//console.log(JSON.stringify(psBG));
let m = new Array(N + 1);
m[0] = new Array((ps.length >> 1) + 1);
for (let i=0; i<m[0].length; i++)
m[0][i] = [0,0];
// for each in N
for (let i=1; i<=N; i++){
m[i] = new Array((ps.length >> 1) + 1);
for (let ii=0; ii<m[0].length; ii++)
m[i][ii] = [0,0];
// for each interval
for (let j=i; j<m[0].length; j++){
m[i][j] = m[i][j-1];
for (let k=j; k>i-1; k--){
// our anchors are the right
// side of each interval, k's are the left
let jj = 2*j;
let kk = 2*k - 1;
// positive means green
// is a majority
if (ps[jj][0] - ps[kk][0] > 0){
let bg = psBG[ps[jj][1]] - psBG[ps[kk][1]];
let s = A[ps[jj][1]][1] - A[ps[kk][1]][0] - bg;
let r = s / (bg + s);
let r1 = C * s / totalG;
let candidate = r + r1 + m[i-1][j-1][0];
if (candidate > m[i][j][0]){
m[i][j] = [
candidate,
ps[kk][1] + ',' + ps[jj][1],
bg, s, r, r1,k,m[i-1][j-1][0]
];
}
}
}
}
}
/*
for (row of m)
console.log(JSON.stringify(
row.map(l => l.map(x => typeof x != 'number' ? x : rnd(x)))));
*/
let result = new Array(N);
let j = m[0].length - 1;
for (let i=N; i>0; i--){
let [_,idxs,w,x,y,z,k] = m[i][j];
let [l,r] = idxs.split(',');
result[i-1] = [A[l][0], A[r][1], 'g'];
j = k - 1;
}
return result;
}
function show(A, last){
if (last[1] != A[A.length-1])
A.push(last);
let s = '';
let j;
for (let i=A.length-1; i>=0; i--){
let [l, r, c] = A[i];
let cc = c == 'g' ? 'X' : '.';
for (let j=r-1; j>=l; j--)
s = cc + s;
if (i > 0)
for (let j=l-1; j>=A[i-1][1]; j--)
s = '.' + s
}
for (let j=A[0][0]-1; j>=0; j--)
s = '.' + s
console.log(s);
return s;
}
function g(A, N, C){
const ts = f(A, N, C);
//console.log(JSON.stringify(ts));
show(A, A[A.length-1]);
show(ts, A[A.length-1]);
}
var a = [
[0,5,'b'],
[5,9,'g'],
[9,10,'b'],
[10,15,'g'],
[15,40,'b'],
[40,41,'g'],
[41,43,'b'],
[43,44,'g'],
[44,45,'b'],
[45,46,'g'],
[46,55,'b'],
[55,65,'g'],
[65,100,'b']
];
// (input, N, C)
g(a, 2, 2);
console.log('');
g(a, 3, 2);
console.log('');
g(a, 4, 2);
console.log('');
g(a, 4, 5);
function rnd(n, d=2){
let m = Math.pow(10,d)
return Math.round(m*n) / m;
}
function f(A, N, C){
let ps = [[0,0]];
let psBG = [0];
let totalG = 0;
A.unshift([0,0]);
for (let i=1; i<A.length; i++){
let [l,r,c] = A[i];
if (c == 'g'){
totalG += r - l;
let prevI = ps[ps.length-1][1];
let d = l - A[prevI][1];
let prevS = ps[ps.length-1][0];
ps.push(
[prevS - d, i, 'l'],
[prevS - d + r - l, i, 'r']
);
psBG[i] = psBG[i-1];
} else {
psBG[i] = psBG[i-1] + r - l;
}
}
//console.log(JSON.stringify(A));
//console.log('');
//console.log(JSON.stringify(ps));
//console.log('');
//console.log(JSON.stringify(psBG));
let m = new Array(N + 1);
m[0] = new Array((ps.length >> 1) + 1);
for (let i=0; i<m[0].length; i++)
m[0][i] = [0,0];
// for each in N
for (let i=1; i<=N; i++){
m[i] = new Array((ps.length >> 1) + 1);
for (let ii=0; ii<m[0].length; ii++)
m[i][ii] = [0,0];
// for each interval
for (let j=i; j<m[0].length; j++){
m[i][j] = m[i][j-1];
for (let k=j; k>i-1; k--){
// our anchors are the right
// side of each interval, k's are the left
let jj = 2*j;
let kk = 2*k - 1;
// positive means green
// is a majority
if (ps[jj][0] - ps[kk][0] > 0){
let bg = psBG[ps[jj][1]] - psBG[ps[kk][1]];
let s = A[ps[jj][1]][1] - A[ps[kk][1]][0] - bg;
let r = s / (bg + s);
let r1 = C * s / totalG;
let candidate = r + r1 + m[i-1][j-1][0];
if (candidate > m[i][j][0]){
m[i][j] = [
candidate,
ps[kk][1] + ',' + ps[jj][1],
bg, s, r, r1,k,m[i-1][j-1][0]
];
}
}
}
}
}
/*
for (row of m)
console.log(JSON.stringify(
row.map(l => l.map(x => typeof x != 'number' ? x : rnd(x)))));
*/
let result = new Array(N);
let j = m[0].length - 1;
for (let i=N; i>0; i--){
let [_,idxs,w,x,y,z,k] = m[i][j];
let [l,r] = idxs.split(',');
result[i-1] = [A[l][0], A[r][1], 'g'];
j = k - 1;
}
return result;
}
function show(A, last){
if (last[1] != A[A.length-1])
A.push(last);
let s = '';
let j;
for (let i=A.length-1; i>=0; i--){
let [l, r, c] = A[i];
let cc = c == 'g' ? 'X' : '.';
for (let j=r-1; j>=l; j--)
s = cc + s;
if (i > 0)
for (let j=l-1; j>=A[i-1][1]; j--)
s = '.' + s
}
for (let j=A[0][0]-1; j>=0; j--)
s = '.' + s
console.log(s);
return s;
}
function g(A, N, C){
const ts = f(A, N, C);
//console.log(JSON.stringify(ts));
show(A, A[A.length-1]);
show(ts, A[A.length-1]);
}
var a = [
[0,5,'b'],
[5,9,'g'],
[9,10,'b'],
[10,15,'g'],
[15,40,'b'],
[40,41,'g'],
[41,43,'b'],
[43,44,'g'],
[44,45,'b'],
[45,46,'g'],
[46,55,'b'],
[55,65,'g'],
[65,100,'b']
];
// (input, N, C)
g(a, 2, 2);
console.log('');
g(a, 3, 2);
console.log('');
g(a, 4, 2);
console.log('');
g(a, 4, 5);
function rnd(n, d=2){
let m = Math.pow(10,d)
return Math.round(m*n) / m;
}
function f(A, N, C){
let ps = [[0,0]];
let psBG = [0];
let totalG = 0;
A.unshift([0,0]);
for (let i=1; i<A.length; i++){
let [l,r,c] = A[i];
if (c == 'g'){
totalG += r - l;
let prevI = ps[ps.length-1][1];
let d = l - A[prevI][1];
let prevS = ps[ps.length-1][0];
ps.push(
[prevS - d, i, 'l'],
[prevS - d + r - l, i, 'r']
);
psBG[i] = psBG[i-1];
} else {
psBG[i] = psBG[i-1] + r - l;
}
}
//console.log(JSON.stringify(A));
//console.log('');
//console.log(JSON.stringify(ps));
//console.log('');
//console.log(JSON.stringify(psBG));
let m = new Array(N + 1);
m[0] = new Array((ps.length >> 1) + 1);
for (let i=0; i<m[0].length; i++)
m[0][i] = [0,0];
// for each in N
for (let i=1; i<=N; i++){
m[i] = new Array((ps.length >> 1) + 1);
for (let ii=0; ii<m[0].length; ii++)
m[i][ii] = [0,0];
// for each interval
for (let j=i; j<m[0].length; j++){
m[i][j] = m[i][j-1];
for (let k=j; k>i-1; k--){
// our anchors are the right
// side of each interval, k's are the left
let jj = 2*j;
let kk = 2*k - 1;
// positive means green
// is a majority
if (ps[jj][0] - ps[kk][0] > 0){
let bg = psBG[ps[jj][1]] - psBG[ps[kk][1]];
let s = A[ps[jj][1]][1] - A[ps[kk][1]][0] - bg;
let r = s / (bg + s);
let r1 = C * s / totalG;
let candidate = r + r1 + m[i-1][j-1][0];
if (candidate > m[i][j][0]){
m[i][j] = [
candidate,
ps[kk][1] + ',' + ps[jj][1],
bg, s, r, r1,k,m[i-1][j-1][0]
];
}
}
}
}
}
/*
for (row of m)
console.log(JSON.stringify(
row.map(l => l.map(x => typeof x != 'number' ? x : rnd(x)))));
*/
let result = new Array(N);
let j = m[0].length - 1;
for (let i=N; i>0; i--){
let [_,idxs,w,x,y,z,k] = m[i][j];
let [l,r] = idxs.split(',');
result[i-1] = [A[l][0], A[r][1], 'g'];
j = k - 1;
}
return result;
}
function show(A, last){
if (last[1] != A[A.length-1])
A.push(last);
let s = '';
let j;
for (let i=A.length-1; i>=0; i--){
let [l, r, c] = A[i];
let cc = c == 'g' ? 'X' : '.';
for (let j=r-1; j>=l; j--)
s = cc + s;
if (i > 0)
for (let j=l-1; j>=A[i-1][1]; j--)
s = '.' + s
}
for (let j=A[0][0]-1; j>=0; j--)
s = '.' + s
console.log(s);
return s;
}
function g(A, N, C){
const ts = f(A, N, C);
//console.log(JSON.stringify(ts));
show(A, A[A.length-1]);
show(ts, A[A.length-1]);
}
var a = [
[0,5,'b'],
[5,9,'g'],
[9,10,'b'],
[10,15,'g'],
[15,40,'b'],
[40,41,'g'],
[41,43,'b'],
[43,44,'g'],
[44,45,'b'],
[45,46,'g'],
[46,55,'b'],
[55,65,'g'],
[65,100,'b']
];
// (input, N, C)
g(a, 2, 2);
console.log('');
g(a, 3, 2);
console.log('');
g(a, 4, 2);
console.log('');
g(a, 4, 5);
edited Jan 2 at 23:51
answered Jan 2 at 4:56
גלעד ברקןגלעד ברקן
12.5k21441
12.5k21441
1
"not sure that restricting candidate intervals to sections with a majority of the target colour is always warranted but it may be a useful heuristic depending on the desired outcome" - I agree it depends on the desired outcome. The current problem statement "to produce a visually similar plot" leaves room for interpretation and therefore there can be different formulations of the objective function. Also, I liked the idea to reduce the search space to a function of the number of input intervals, instead of the max width.
– Georgi Gerganov
Jan 2 at 13:50
@GeorgiGerganov cool, thanks for reading and commenting!
– גלעד ברקן
Jan 2 at 20:11
add a comment |
1
"not sure that restricting candidate intervals to sections with a majority of the target colour is always warranted but it may be a useful heuristic depending on the desired outcome" - I agree it depends on the desired outcome. The current problem statement "to produce a visually similar plot" leaves room for interpretation and therefore there can be different formulations of the objective function. Also, I liked the idea to reduce the search space to a function of the number of input intervals, instead of the max width.
– Georgi Gerganov
Jan 2 at 13:50
@GeorgiGerganov cool, thanks for reading and commenting!
– גלעד ברקן
Jan 2 at 20:11
1
1
"not sure that restricting candidate intervals to sections with a majority of the target colour is always warranted but it may be a useful heuristic depending on the desired outcome" - I agree it depends on the desired outcome. The current problem statement "to produce a visually similar plot" leaves room for interpretation and therefore there can be different formulations of the objective function. Also, I liked the idea to reduce the search space to a function of the number of input intervals, instead of the max width.
– Georgi Gerganov
Jan 2 at 13:50
"not sure that restricting candidate intervals to sections with a majority of the target colour is always warranted but it may be a useful heuristic depending on the desired outcome" - I agree it depends on the desired outcome. The current problem statement "to produce a visually similar plot" leaves room for interpretation and therefore there can be different formulations of the objective function. Also, I liked the idea to reduce the search space to a function of the number of input intervals, instead of the max width.
– Georgi Gerganov
Jan 2 at 13:50
@GeorgiGerganov cool, thanks for reading and commenting!
– גלעד ברקן
Jan 2 at 20:11
@GeorgiGerganov cool, thanks for reading and commenting!
– גלעד ברקן
Jan 2 at 20:11
add a comment |
I would suggest using K-means it is an algorithm used to group data(a more detailed explanation here: https://en.wikipedia.org/wiki/K-means_clustering and here https://scikit-learn.org/stable/modules/generated/sklearn.cluster.KMeans.html)
this would be a brief explanation of how the function should look like, hope it is helpful.
from sklearn.cluster import KMeans
import numpy as np
def downsample(input, cluster = 25):
# you will need to group your labels in a nmpy array as shown bellow
# for the sake of example I will take just a random array
X = np.array([[1, 2], [1, 4], [1, 0],[4, 2], [4, 4], [4, 0]])
# n_clusters will be the same as desired output
kmeans = KMeans(n_clusters= cluster, random_state=0).fit(X)
# then you can iterate through labels that was assigned to every entr of your input
# in our case the interval
kmeans_list = [None]*cluster
for i in range(0, X.shape[0]):
kmeans_list[kmeans.labels_[i]].append(X[i])
# after that you will basicly have a list of lists and every inner list will contain all points that corespond to a
# specific label
ret = #return list
for label_list in kmeans_list:
left = 10001000 # a big enough number to exced anything that you will get as an input
right = -left # same here
for entry in label_list:
left = min(left, entry[0])
right = max(right, entry[1])
ret.append([left,right])
return ret
1
Using KMeans is definitely an interesting approach, I could totally see this work. Thanks for your answer @Dan Butmalai
– hampusohlsson
Dec 31 '18 at 9:18
add a comment |
I would suggest using K-means it is an algorithm used to group data(a more detailed explanation here: https://en.wikipedia.org/wiki/K-means_clustering and here https://scikit-learn.org/stable/modules/generated/sklearn.cluster.KMeans.html)
this would be a brief explanation of how the function should look like, hope it is helpful.
from sklearn.cluster import KMeans
import numpy as np
def downsample(input, cluster = 25):
# you will need to group your labels in a nmpy array as shown bellow
# for the sake of example I will take just a random array
X = np.array([[1, 2], [1, 4], [1, 0],[4, 2], [4, 4], [4, 0]])
# n_clusters will be the same as desired output
kmeans = KMeans(n_clusters= cluster, random_state=0).fit(X)
# then you can iterate through labels that was assigned to every entr of your input
# in our case the interval
kmeans_list = [None]*cluster
for i in range(0, X.shape[0]):
kmeans_list[kmeans.labels_[i]].append(X[i])
# after that you will basicly have a list of lists and every inner list will contain all points that corespond to a
# specific label
ret = #return list
for label_list in kmeans_list:
left = 10001000 # a big enough number to exced anything that you will get as an input
right = -left # same here
for entry in label_list:
left = min(left, entry[0])
right = max(right, entry[1])
ret.append([left,right])
return ret
1
Using KMeans is definitely an interesting approach, I could totally see this work. Thanks for your answer @Dan Butmalai
– hampusohlsson
Dec 31 '18 at 9:18
add a comment |
I would suggest using K-means it is an algorithm used to group data(a more detailed explanation here: https://en.wikipedia.org/wiki/K-means_clustering and here https://scikit-learn.org/stable/modules/generated/sklearn.cluster.KMeans.html)
this would be a brief explanation of how the function should look like, hope it is helpful.
from sklearn.cluster import KMeans
import numpy as np
def downsample(input, cluster = 25):
# you will need to group your labels in a nmpy array as shown bellow
# for the sake of example I will take just a random array
X = np.array([[1, 2], [1, 4], [1, 0],[4, 2], [4, 4], [4, 0]])
# n_clusters will be the same as desired output
kmeans = KMeans(n_clusters= cluster, random_state=0).fit(X)
# then you can iterate through labels that was assigned to every entr of your input
# in our case the interval
kmeans_list = [None]*cluster
for i in range(0, X.shape[0]):
kmeans_list[kmeans.labels_[i]].append(X[i])
# after that you will basicly have a list of lists and every inner list will contain all points that corespond to a
# specific label
ret = #return list
for label_list in kmeans_list:
left = 10001000 # a big enough number to exced anything that you will get as an input
right = -left # same here
for entry in label_list:
left = min(left, entry[0])
right = max(right, entry[1])
ret.append([left,right])
return ret
I would suggest using K-means it is an algorithm used to group data(a more detailed explanation here: https://en.wikipedia.org/wiki/K-means_clustering and here https://scikit-learn.org/stable/modules/generated/sklearn.cluster.KMeans.html)
this would be a brief explanation of how the function should look like, hope it is helpful.
from sklearn.cluster import KMeans
import numpy as np
def downsample(input, cluster = 25):
# you will need to group your labels in a nmpy array as shown bellow
# for the sake of example I will take just a random array
X = np.array([[1, 2], [1, 4], [1, 0],[4, 2], [4, 4], [4, 0]])
# n_clusters will be the same as desired output
kmeans = KMeans(n_clusters= cluster, random_state=0).fit(X)
# then you can iterate through labels that was assigned to every entr of your input
# in our case the interval
kmeans_list = [None]*cluster
for i in range(0, X.shape[0]):
kmeans_list[kmeans.labels_[i]].append(X[i])
# after that you will basicly have a list of lists and every inner list will contain all points that corespond to a
# specific label
ret = #return list
for label_list in kmeans_list:
left = 10001000 # a big enough number to exced anything that you will get as an input
right = -left # same here
for entry in label_list:
left = min(left, entry[0])
right = max(right, entry[1])
ret.append([left,right])
return ret
edited Jan 6 at 16:36
answered Dec 30 '18 at 19:16
Dan ButmalaiDan Butmalai
264
264
1
Using KMeans is definitely an interesting approach, I could totally see this work. Thanks for your answer @Dan Butmalai
– hampusohlsson
Dec 31 '18 at 9:18
add a comment |
1
Using KMeans is definitely an interesting approach, I could totally see this work. Thanks for your answer @Dan Butmalai
– hampusohlsson
Dec 31 '18 at 9:18
1
1
Using KMeans is definitely an interesting approach, I could totally see this work. Thanks for your answer @Dan Butmalai
– hampusohlsson
Dec 31 '18 at 9:18
Using KMeans is definitely an interesting approach, I could totally see this work. Thanks for your answer @Dan Butmalai
– hampusohlsson
Dec 31 '18 at 9:18
add a comment |
Thanks for contributing an answer to Stack Overflow!
- Please be sure to answer the question. Provide details and share your research!
But avoid …
- Asking for help, clarification, or responding to other answers.
- Making statements based on opinion; back them up with references or personal experience.
To learn more, see our tips on writing great answers.
Sign up or log in
StackExchange.ready(function () {
StackExchange.helpers.onClickDraftSave('#login-link');
});
Sign up using Google
Sign up using Facebook
Sign up using Email and Password
Post as a guest
Required, but never shown
StackExchange.ready(
function () {
StackExchange.openid.initPostLogin('.new-post-login', 'https%3a%2f%2fstackoverflow.com%2fquestions%2f53978490%2falgorithm-for-downsampling-array-of-intervals%23new-answer', 'question_page');
}
);
Post as a guest
Required, but never shown
Sign up or log in
StackExchange.ready(function () {
StackExchange.helpers.onClickDraftSave('#login-link');
});
Sign up using Google
Sign up using Facebook
Sign up using Email and Password
Post as a guest
Required, but never shown
Sign up or log in
StackExchange.ready(function () {
StackExchange.helpers.onClickDraftSave('#login-link');
});
Sign up using Google
Sign up using Facebook
Sign up using Email and Password
Post as a guest
Required, but never shown
Sign up or log in
StackExchange.ready(function () {
StackExchange.helpers.onClickDraftSave('#login-link');
});
Sign up using Google
Sign up using Facebook
Sign up using Email and Password
Sign up using Google
Sign up using Facebook
Sign up using Email and Password
Post as a guest
Required, but never shown
Required, but never shown
Required, but never shown
Required, but never shown
Required, but never shown
Required, but never shown
Required, but never shown
Required, but never shown
Required, but never shown
fYQzASQY
Given N input intervals, exactly how many output intervals do you want? Or is there another way that the desired output is defined, like minimum interval size?
– Matt Timmermans
Dec 30 '18 at 16:21
@MattTimmermans the exact output number is not important, but I'd like to supply a maximum number of approximate outputs. Something like
output_length = min(len(input), 25)
– hampusohlsson
Dec 31 '18 at 9:15