Vecrtorized evluation of function defined by matrix over grid
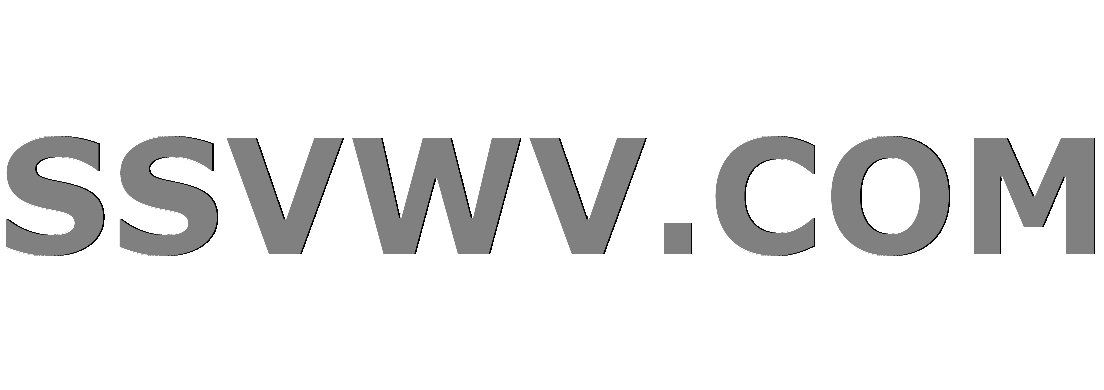
Multi tool use
I'm looking to plot the value of a function defined by a matrix over a grid of values.
Let S
be an invertable 2x2 matrix and let x
be a 2-dimensional vector. How can vectorize the evaluation of x@S@x
over a two dimensional grid?
Here is how I currently do it. It works, but takes a beat to perform the computation since the grid is so fine.
#Initialize Matrix
S = np.zeros(shape = (2,2))
while np.linalg.matrix_rank(S)<S.shape[1]:
S = np.random.randint(-5,5+1, size = (2,2))
X,Y = [j.ravel() for j in np.meshgrid(np.linspace(-2,2,1001),np.linspace(-2,2,1001))]
Z = np.zeros_like(X)
for i,v in enumerate(zip(X,Y)):
v = np.array(v)
Z[i] = v@S@v
n = int(np.sqrt(X.size))
Z = Z.reshape(n,n)
X = X.reshape(n,n)
Y = Y.reshape(n,n)
plt.contour(X,Y,Z)
python numpy
add a comment |
I'm looking to plot the value of a function defined by a matrix over a grid of values.
Let S
be an invertable 2x2 matrix and let x
be a 2-dimensional vector. How can vectorize the evaluation of x@S@x
over a two dimensional grid?
Here is how I currently do it. It works, but takes a beat to perform the computation since the grid is so fine.
#Initialize Matrix
S = np.zeros(shape = (2,2))
while np.linalg.matrix_rank(S)<S.shape[1]:
S = np.random.randint(-5,5+1, size = (2,2))
X,Y = [j.ravel() for j in np.meshgrid(np.linspace(-2,2,1001),np.linspace(-2,2,1001))]
Z = np.zeros_like(X)
for i,v in enumerate(zip(X,Y)):
v = np.array(v)
Z[i] = v@S@v
n = int(np.sqrt(X.size))
Z = Z.reshape(n,n)
X = X.reshape(n,n)
Y = Y.reshape(n,n)
plt.contour(X,Y,Z)
python numpy
add a comment |
I'm looking to plot the value of a function defined by a matrix over a grid of values.
Let S
be an invertable 2x2 matrix and let x
be a 2-dimensional vector. How can vectorize the evaluation of x@S@x
over a two dimensional grid?
Here is how I currently do it. It works, but takes a beat to perform the computation since the grid is so fine.
#Initialize Matrix
S = np.zeros(shape = (2,2))
while np.linalg.matrix_rank(S)<S.shape[1]:
S = np.random.randint(-5,5+1, size = (2,2))
X,Y = [j.ravel() for j in np.meshgrid(np.linspace(-2,2,1001),np.linspace(-2,2,1001))]
Z = np.zeros_like(X)
for i,v in enumerate(zip(X,Y)):
v = np.array(v)
Z[i] = v@S@v
n = int(np.sqrt(X.size))
Z = Z.reshape(n,n)
X = X.reshape(n,n)
Y = Y.reshape(n,n)
plt.contour(X,Y,Z)
python numpy
I'm looking to plot the value of a function defined by a matrix over a grid of values.
Let S
be an invertable 2x2 matrix and let x
be a 2-dimensional vector. How can vectorize the evaluation of x@S@x
over a two dimensional grid?
Here is how I currently do it. It works, but takes a beat to perform the computation since the grid is so fine.
#Initialize Matrix
S = np.zeros(shape = (2,2))
while np.linalg.matrix_rank(S)<S.shape[1]:
S = np.random.randint(-5,5+1, size = (2,2))
X,Y = [j.ravel() for j in np.meshgrid(np.linspace(-2,2,1001),np.linspace(-2,2,1001))]
Z = np.zeros_like(X)
for i,v in enumerate(zip(X,Y)):
v = np.array(v)
Z[i] = v@S@v
n = int(np.sqrt(X.size))
Z = Z.reshape(n,n)
X = X.reshape(n,n)
Y = Y.reshape(n,n)
plt.contour(X,Y,Z)
python numpy
python numpy
asked Dec 27 '18 at 20:08
Demetri Pananos
1,9911231
1,9911231
add a comment |
add a comment |
1 Answer
1
active
oldest
votes
Simplest would be with stacking those X,Y
into a 2-column 2D array and then using np.einsum
to replace the loopy matrix-multiplications -
p = np.column_stack((X,Y)) # or np.stack((X,Y)).T
Zout = np.einsum('ij,jk,ik->i',p,S,p,optimize=True)
add a comment |
Your Answer
StackExchange.ifUsing("editor", function () {
StackExchange.using("externalEditor", function () {
StackExchange.using("snippets", function () {
StackExchange.snippets.init();
});
});
}, "code-snippets");
StackExchange.ready(function() {
var channelOptions = {
tags: "".split(" "),
id: "1"
};
initTagRenderer("".split(" "), "".split(" "), channelOptions);
StackExchange.using("externalEditor", function() {
// Have to fire editor after snippets, if snippets enabled
if (StackExchange.settings.snippets.snippetsEnabled) {
StackExchange.using("snippets", function() {
createEditor();
});
}
else {
createEditor();
}
});
function createEditor() {
StackExchange.prepareEditor({
heartbeatType: 'answer',
autoActivateHeartbeat: false,
convertImagesToLinks: true,
noModals: true,
showLowRepImageUploadWarning: true,
reputationToPostImages: 10,
bindNavPrevention: true,
postfix: "",
imageUploader: {
brandingHtml: "Powered by u003ca class="icon-imgur-white" href="https://imgur.com/"u003eu003c/au003e",
contentPolicyHtml: "User contributions licensed under u003ca href="https://creativecommons.org/licenses/by-sa/3.0/"u003ecc by-sa 3.0 with attribution requiredu003c/au003e u003ca href="https://stackoverflow.com/legal/content-policy"u003e(content policy)u003c/au003e",
allowUrls: true
},
onDemand: true,
discardSelector: ".discard-answer"
,immediatelyShowMarkdownHelp:true
});
}
});
Sign up or log in
StackExchange.ready(function () {
StackExchange.helpers.onClickDraftSave('#login-link');
});
Sign up using Google
Sign up using Facebook
Sign up using Email and Password
Post as a guest
Required, but never shown
StackExchange.ready(
function () {
StackExchange.openid.initPostLogin('.new-post-login', 'https%3a%2f%2fstackoverflow.com%2fquestions%2f53950335%2fvecrtorized-evluation-of-function-defined-by-matrix-over-grid%23new-answer', 'question_page');
}
);
Post as a guest
Required, but never shown
1 Answer
1
active
oldest
votes
1 Answer
1
active
oldest
votes
active
oldest
votes
active
oldest
votes
Simplest would be with stacking those X,Y
into a 2-column 2D array and then using np.einsum
to replace the loopy matrix-multiplications -
p = np.column_stack((X,Y)) # or np.stack((X,Y)).T
Zout = np.einsum('ij,jk,ik->i',p,S,p,optimize=True)
add a comment |
Simplest would be with stacking those X,Y
into a 2-column 2D array and then using np.einsum
to replace the loopy matrix-multiplications -
p = np.column_stack((X,Y)) # or np.stack((X,Y)).T
Zout = np.einsum('ij,jk,ik->i',p,S,p,optimize=True)
add a comment |
Simplest would be with stacking those X,Y
into a 2-column 2D array and then using np.einsum
to replace the loopy matrix-multiplications -
p = np.column_stack((X,Y)) # or np.stack((X,Y)).T
Zout = np.einsum('ij,jk,ik->i',p,S,p,optimize=True)
Simplest would be with stacking those X,Y
into a 2-column 2D array and then using np.einsum
to replace the loopy matrix-multiplications -
p = np.column_stack((X,Y)) # or np.stack((X,Y)).T
Zout = np.einsum('ij,jk,ik->i',p,S,p,optimize=True)
edited Dec 27 '18 at 20:42
answered Dec 27 '18 at 20:34


Divakar
154k1483172
154k1483172
add a comment |
add a comment |
Thanks for contributing an answer to Stack Overflow!
- Please be sure to answer the question. Provide details and share your research!
But avoid …
- Asking for help, clarification, or responding to other answers.
- Making statements based on opinion; back them up with references or personal experience.
To learn more, see our tips on writing great answers.
Some of your past answers have not been well-received, and you're in danger of being blocked from answering.
Please pay close attention to the following guidance:
- Please be sure to answer the question. Provide details and share your research!
But avoid …
- Asking for help, clarification, or responding to other answers.
- Making statements based on opinion; back them up with references or personal experience.
To learn more, see our tips on writing great answers.
Sign up or log in
StackExchange.ready(function () {
StackExchange.helpers.onClickDraftSave('#login-link');
});
Sign up using Google
Sign up using Facebook
Sign up using Email and Password
Post as a guest
Required, but never shown
StackExchange.ready(
function () {
StackExchange.openid.initPostLogin('.new-post-login', 'https%3a%2f%2fstackoverflow.com%2fquestions%2f53950335%2fvecrtorized-evluation-of-function-defined-by-matrix-over-grid%23new-answer', 'question_page');
}
);
Post as a guest
Required, but never shown
Sign up or log in
StackExchange.ready(function () {
StackExchange.helpers.onClickDraftSave('#login-link');
});
Sign up using Google
Sign up using Facebook
Sign up using Email and Password
Post as a guest
Required, but never shown
Sign up or log in
StackExchange.ready(function () {
StackExchange.helpers.onClickDraftSave('#login-link');
});
Sign up using Google
Sign up using Facebook
Sign up using Email and Password
Post as a guest
Required, but never shown
Sign up or log in
StackExchange.ready(function () {
StackExchange.helpers.onClickDraftSave('#login-link');
});
Sign up using Google
Sign up using Facebook
Sign up using Email and Password
Sign up using Google
Sign up using Facebook
Sign up using Email and Password
Post as a guest
Required, but never shown
Required, but never shown
Required, but never shown
Required, but never shown
Required, but never shown
Required, but never shown
Required, but never shown
Required, but never shown
Required, but never shown
K 7B,zlx934zK8,ss l5uRkI8pcmof osOsvLc,fbh