ValueError: non-broadcastable output operand with shape (3,1) doesn't match the broadcast shape (3,4)
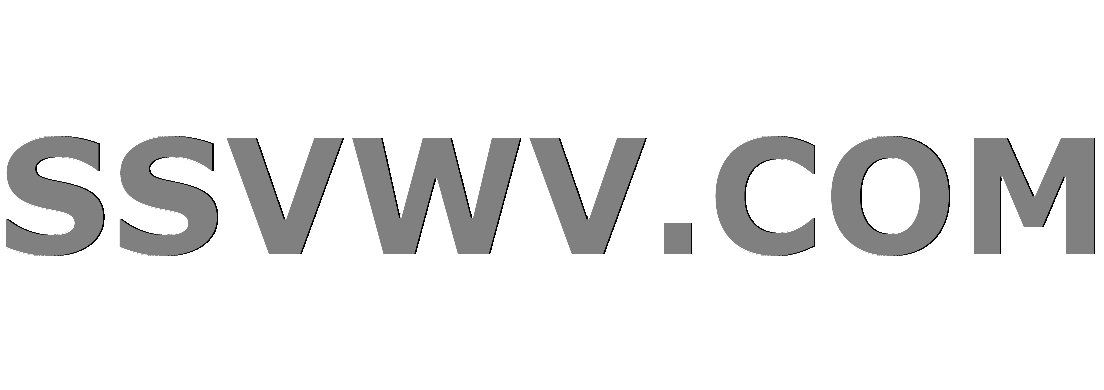
Multi tool use
.everyoneloves__top-leaderboard:empty,.everyoneloves__mid-leaderboard:empty,.everyoneloves__bot-mid-leaderboard:empty{ height:90px;width:728px;box-sizing:border-box;
}
I recently started to follow along with Siraj Raval's Deep Learning tutorials on YouTube, but I an error came up when I tried to run my code. The code is from the second episode of his series, How To Make A Neural Network. When I ran the code I got the error:
Traceback (most recent call last):
File "C:UsersdpoppDocumentsMachine Learningfirst_neural_net.py", line 66, in <module>
neural_network.train(training_set_inputs, training_set_outputs, 10000)
File "C:UsersdpoppDocumentsMachine Learningfirst_neural_net.py", line 44, in train
self.synaptic_weights += adjustment
ValueError: non-broadcastable output operand with shape (3,1) doesn't match the broadcast shape (3,4)
I checked multiple times with his code and couldn't find any differences, and even tried copying and pasting his code from the GitHub link. This is the code I have now:
from numpy import exp, array, random, dot
class NeuralNetwork():
def __init__(self):
# Seed the random number generator, so it generates the same numbers
# every time the program runs.
random.seed(1)
# We model a single neuron, with 3 input connections and 1 output connection.
# We assign random weights to a 3 x 1 matrix, with values in the range -1 to 1
# and mean 0.
self.synaptic_weights = 2 * random.random((3, 1)) - 1
# The Sigmoid function, which describes an S shaped curve.
# We pass the weighted sum of the inputs through this function to
# normalise them between 0 and 1.
def __sigmoid(self, x):
return 1 / (1 + exp(-x))
# The derivative of the Sigmoid function.
# This is the gradient of the Sigmoid curve.
# It indicates how confident we are about the existing weight.
def __sigmoid_derivative(self, x):
return x * (1 - x)
# We train the neural network through a process of trial and error.
# Adjusting the synaptic weights each time.
def train(self, training_set_inputs, training_set_outputs, number_of_training_iterations):
for iteration in range(number_of_training_iterations):
# Pass the training set through our neural network (a single neuron).
output = self.think(training_set_inputs)
# Calculate the error (The difference between the desired output
# and the predicted output).
error = training_set_outputs - output
# Multiply the error by the input and again by the gradient of the Sigmoid curve.
# This means less confident weights are adjusted more.
# This means inputs, which are zero, do not cause changes to the weights.
adjustment = dot(training_set_inputs.T, error * self.__sigmoid_derivative(output))
# Adjust the weights.
self.synaptic_weights += adjustment
# The neural network thinks.
def think(self, inputs):
# Pass inputs through our neural network (our single neuron).
return self.__sigmoid(dot(inputs, self.synaptic_weights))
if __name__ == '__main__':
# Initialize a single neuron neural network
neural_network = NeuralNetwork()
print("Random starting synaptic weights:")
print(neural_network.synaptic_weights)
# The training set. We have 4 examples, each consisting of 3 input values
# and 1 output value.
training_set_inputs = array([[0, 0, 1], [1, 1, 1], [1, 0, 1], [0, 1, 1]])
training_set_outputs = array([[0, 1, 1, 0]])
# Train the neural network using a training set
# Do it 10,000 times and make small adjustments each time
neural_network.train(training_set_inputs, training_set_outputs, 10000)
print("New Synaptic weights after training:")
print(neural_network.synaptic_weights)
# Test the neural net with a new situation
print("Considering new situation [1, 0, 0] -> ?:")
print(neural_network.think(array([[1, 0, 0]])))
Even after copying and pasting the same code that worked in Siraj's episode, I'm still getting the same error.
I just started out look into artificial intelligence, and don't understand what the error means. Could someone please explain what it means and how to fix it? Thanks!
python neural-network artificial-intelligence
add a comment |
I recently started to follow along with Siraj Raval's Deep Learning tutorials on YouTube, but I an error came up when I tried to run my code. The code is from the second episode of his series, How To Make A Neural Network. When I ran the code I got the error:
Traceback (most recent call last):
File "C:UsersdpoppDocumentsMachine Learningfirst_neural_net.py", line 66, in <module>
neural_network.train(training_set_inputs, training_set_outputs, 10000)
File "C:UsersdpoppDocumentsMachine Learningfirst_neural_net.py", line 44, in train
self.synaptic_weights += adjustment
ValueError: non-broadcastable output operand with shape (3,1) doesn't match the broadcast shape (3,4)
I checked multiple times with his code and couldn't find any differences, and even tried copying and pasting his code from the GitHub link. This is the code I have now:
from numpy import exp, array, random, dot
class NeuralNetwork():
def __init__(self):
# Seed the random number generator, so it generates the same numbers
# every time the program runs.
random.seed(1)
# We model a single neuron, with 3 input connections and 1 output connection.
# We assign random weights to a 3 x 1 matrix, with values in the range -1 to 1
# and mean 0.
self.synaptic_weights = 2 * random.random((3, 1)) - 1
# The Sigmoid function, which describes an S shaped curve.
# We pass the weighted sum of the inputs through this function to
# normalise them between 0 and 1.
def __sigmoid(self, x):
return 1 / (1 + exp(-x))
# The derivative of the Sigmoid function.
# This is the gradient of the Sigmoid curve.
# It indicates how confident we are about the existing weight.
def __sigmoid_derivative(self, x):
return x * (1 - x)
# We train the neural network through a process of trial and error.
# Adjusting the synaptic weights each time.
def train(self, training_set_inputs, training_set_outputs, number_of_training_iterations):
for iteration in range(number_of_training_iterations):
# Pass the training set through our neural network (a single neuron).
output = self.think(training_set_inputs)
# Calculate the error (The difference between the desired output
# and the predicted output).
error = training_set_outputs - output
# Multiply the error by the input and again by the gradient of the Sigmoid curve.
# This means less confident weights are adjusted more.
# This means inputs, which are zero, do not cause changes to the weights.
adjustment = dot(training_set_inputs.T, error * self.__sigmoid_derivative(output))
# Adjust the weights.
self.synaptic_weights += adjustment
# The neural network thinks.
def think(self, inputs):
# Pass inputs through our neural network (our single neuron).
return self.__sigmoid(dot(inputs, self.synaptic_weights))
if __name__ == '__main__':
# Initialize a single neuron neural network
neural_network = NeuralNetwork()
print("Random starting synaptic weights:")
print(neural_network.synaptic_weights)
# The training set. We have 4 examples, each consisting of 3 input values
# and 1 output value.
training_set_inputs = array([[0, 0, 1], [1, 1, 1], [1, 0, 1], [0, 1, 1]])
training_set_outputs = array([[0, 1, 1, 0]])
# Train the neural network using a training set
# Do it 10,000 times and make small adjustments each time
neural_network.train(training_set_inputs, training_set_outputs, 10000)
print("New Synaptic weights after training:")
print(neural_network.synaptic_weights)
# Test the neural net with a new situation
print("Considering new situation [1, 0, 0] -> ?:")
print(neural_network.think(array([[1, 0, 0]])))
Even after copying and pasting the same code that worked in Siraj's episode, I'm still getting the same error.
I just started out look into artificial intelligence, and don't understand what the error means. Could someone please explain what it means and how to fix it? Thanks!
python neural-network artificial-intelligence
Broadcasting
– wwii
Nov 26 '17 at 7:23
add a comment |
I recently started to follow along with Siraj Raval's Deep Learning tutorials on YouTube, but I an error came up when I tried to run my code. The code is from the second episode of his series, How To Make A Neural Network. When I ran the code I got the error:
Traceback (most recent call last):
File "C:UsersdpoppDocumentsMachine Learningfirst_neural_net.py", line 66, in <module>
neural_network.train(training_set_inputs, training_set_outputs, 10000)
File "C:UsersdpoppDocumentsMachine Learningfirst_neural_net.py", line 44, in train
self.synaptic_weights += adjustment
ValueError: non-broadcastable output operand with shape (3,1) doesn't match the broadcast shape (3,4)
I checked multiple times with his code and couldn't find any differences, and even tried copying and pasting his code from the GitHub link. This is the code I have now:
from numpy import exp, array, random, dot
class NeuralNetwork():
def __init__(self):
# Seed the random number generator, so it generates the same numbers
# every time the program runs.
random.seed(1)
# We model a single neuron, with 3 input connections and 1 output connection.
# We assign random weights to a 3 x 1 matrix, with values in the range -1 to 1
# and mean 0.
self.synaptic_weights = 2 * random.random((3, 1)) - 1
# The Sigmoid function, which describes an S shaped curve.
# We pass the weighted sum of the inputs through this function to
# normalise them between 0 and 1.
def __sigmoid(self, x):
return 1 / (1 + exp(-x))
# The derivative of the Sigmoid function.
# This is the gradient of the Sigmoid curve.
# It indicates how confident we are about the existing weight.
def __sigmoid_derivative(self, x):
return x * (1 - x)
# We train the neural network through a process of trial and error.
# Adjusting the synaptic weights each time.
def train(self, training_set_inputs, training_set_outputs, number_of_training_iterations):
for iteration in range(number_of_training_iterations):
# Pass the training set through our neural network (a single neuron).
output = self.think(training_set_inputs)
# Calculate the error (The difference between the desired output
# and the predicted output).
error = training_set_outputs - output
# Multiply the error by the input and again by the gradient of the Sigmoid curve.
# This means less confident weights are adjusted more.
# This means inputs, which are zero, do not cause changes to the weights.
adjustment = dot(training_set_inputs.T, error * self.__sigmoid_derivative(output))
# Adjust the weights.
self.synaptic_weights += adjustment
# The neural network thinks.
def think(self, inputs):
# Pass inputs through our neural network (our single neuron).
return self.__sigmoid(dot(inputs, self.synaptic_weights))
if __name__ == '__main__':
# Initialize a single neuron neural network
neural_network = NeuralNetwork()
print("Random starting synaptic weights:")
print(neural_network.synaptic_weights)
# The training set. We have 4 examples, each consisting of 3 input values
# and 1 output value.
training_set_inputs = array([[0, 0, 1], [1, 1, 1], [1, 0, 1], [0, 1, 1]])
training_set_outputs = array([[0, 1, 1, 0]])
# Train the neural network using a training set
# Do it 10,000 times and make small adjustments each time
neural_network.train(training_set_inputs, training_set_outputs, 10000)
print("New Synaptic weights after training:")
print(neural_network.synaptic_weights)
# Test the neural net with a new situation
print("Considering new situation [1, 0, 0] -> ?:")
print(neural_network.think(array([[1, 0, 0]])))
Even after copying and pasting the same code that worked in Siraj's episode, I'm still getting the same error.
I just started out look into artificial intelligence, and don't understand what the error means. Could someone please explain what it means and how to fix it? Thanks!
python neural-network artificial-intelligence
I recently started to follow along with Siraj Raval's Deep Learning tutorials on YouTube, but I an error came up when I tried to run my code. The code is from the second episode of his series, How To Make A Neural Network. When I ran the code I got the error:
Traceback (most recent call last):
File "C:UsersdpoppDocumentsMachine Learningfirst_neural_net.py", line 66, in <module>
neural_network.train(training_set_inputs, training_set_outputs, 10000)
File "C:UsersdpoppDocumentsMachine Learningfirst_neural_net.py", line 44, in train
self.synaptic_weights += adjustment
ValueError: non-broadcastable output operand with shape (3,1) doesn't match the broadcast shape (3,4)
I checked multiple times with his code and couldn't find any differences, and even tried copying and pasting his code from the GitHub link. This is the code I have now:
from numpy import exp, array, random, dot
class NeuralNetwork():
def __init__(self):
# Seed the random number generator, so it generates the same numbers
# every time the program runs.
random.seed(1)
# We model a single neuron, with 3 input connections and 1 output connection.
# We assign random weights to a 3 x 1 matrix, with values in the range -1 to 1
# and mean 0.
self.synaptic_weights = 2 * random.random((3, 1)) - 1
# The Sigmoid function, which describes an S shaped curve.
# We pass the weighted sum of the inputs through this function to
# normalise them between 0 and 1.
def __sigmoid(self, x):
return 1 / (1 + exp(-x))
# The derivative of the Sigmoid function.
# This is the gradient of the Sigmoid curve.
# It indicates how confident we are about the existing weight.
def __sigmoid_derivative(self, x):
return x * (1 - x)
# We train the neural network through a process of trial and error.
# Adjusting the synaptic weights each time.
def train(self, training_set_inputs, training_set_outputs, number_of_training_iterations):
for iteration in range(number_of_training_iterations):
# Pass the training set through our neural network (a single neuron).
output = self.think(training_set_inputs)
# Calculate the error (The difference between the desired output
# and the predicted output).
error = training_set_outputs - output
# Multiply the error by the input and again by the gradient of the Sigmoid curve.
# This means less confident weights are adjusted more.
# This means inputs, which are zero, do not cause changes to the weights.
adjustment = dot(training_set_inputs.T, error * self.__sigmoid_derivative(output))
# Adjust the weights.
self.synaptic_weights += adjustment
# The neural network thinks.
def think(self, inputs):
# Pass inputs through our neural network (our single neuron).
return self.__sigmoid(dot(inputs, self.synaptic_weights))
if __name__ == '__main__':
# Initialize a single neuron neural network
neural_network = NeuralNetwork()
print("Random starting synaptic weights:")
print(neural_network.synaptic_weights)
# The training set. We have 4 examples, each consisting of 3 input values
# and 1 output value.
training_set_inputs = array([[0, 0, 1], [1, 1, 1], [1, 0, 1], [0, 1, 1]])
training_set_outputs = array([[0, 1, 1, 0]])
# Train the neural network using a training set
# Do it 10,000 times and make small adjustments each time
neural_network.train(training_set_inputs, training_set_outputs, 10000)
print("New Synaptic weights after training:")
print(neural_network.synaptic_weights)
# Test the neural net with a new situation
print("Considering new situation [1, 0, 0] -> ?:")
print(neural_network.think(array([[1, 0, 0]])))
Even after copying and pasting the same code that worked in Siraj's episode, I'm still getting the same error.
I just started out look into artificial intelligence, and don't understand what the error means. Could someone please explain what it means and how to fix it? Thanks!
python neural-network artificial-intelligence
python neural-network artificial-intelligence
asked Nov 26 '17 at 6:16
dpopp783dpopp783
2925
2925
Broadcasting
– wwii
Nov 26 '17 at 7:23
add a comment |
Broadcasting
– wwii
Nov 26 '17 at 7:23
Broadcasting
– wwii
Nov 26 '17 at 7:23
Broadcasting
– wwii
Nov 26 '17 at 7:23
add a comment |
2 Answers
2
active
oldest
votes
Change self.synaptic_weights += adjustment
to
self.synaptic_weights = self.synaptic_weights + adjustment
self.synaptic_weights
must have a shape of (3,1) and adjustment
must have a shape of (3,4). While the shapes are broadcastable numpy must not like trying to assign the result with shape (3,4) to an array of shape (3,1)
a = np.ones((3,1))
b = np.random.randint(1,10, (3,4))
>>> a
array([[1],
[1],
[1]])
>>> b
array([[8, 2, 5, 7],
[2, 5, 4, 8],
[7, 7, 6, 6]])
>>> a + b
array([[9, 3, 6, 8],
[3, 6, 5, 9],
[8, 8, 7, 7]])
>>> b += a
>>> b
array([[9, 3, 6, 8],
[3, 6, 5, 9],
[8, 8, 7, 7]])
>>> a
array([[1],
[1],
[1]])
>>> a += b
Traceback (most recent call last):
File "<pyshell#24>", line 1, in <module>
a += b
ValueError: non-broadcastable output operand with shape (3,1) doesn't match the broadcast shape (3,4)
The same error occurs when using numpy.add and specifying a
as the output array
>>> np.add(a,b, out = a)
Traceback (most recent call last):
File "<pyshell#31>", line 1, in <module>
np.add(a,b, out = a)
ValueError: non-broadcastable output operand with shape (3,1) doesn't match the broadcast shape (3,4)
>>>
A new a
needs to be created
>>> a = a + b
>>> a
array([[10, 4, 7, 9],
[ 4, 7, 6, 10],
[ 9, 9, 8, 8]])
>>>
add a comment |
Hopefully, by now you must have executed the code, but the problem between his code and your code is this line:
training_output = np.array([[0,1,1,0]]).T
While transposing don't forget to add 2 square brackets, I had the same problem for the same code, this worked for me.
Thanks
Welcome to Stack Overflow! Please take some time to format your answers before posting to ensure it is readable easily by everyone. You can use backsticks (`) to format inline code for example
– Ferdz
Jan 3 at 21:25
add a comment |
Your Answer
StackExchange.ifUsing("editor", function () {
StackExchange.using("externalEditor", function () {
StackExchange.using("snippets", function () {
StackExchange.snippets.init();
});
});
}, "code-snippets");
StackExchange.ready(function() {
var channelOptions = {
tags: "".split(" "),
id: "1"
};
initTagRenderer("".split(" "), "".split(" "), channelOptions);
StackExchange.using("externalEditor", function() {
// Have to fire editor after snippets, if snippets enabled
if (StackExchange.settings.snippets.snippetsEnabled) {
StackExchange.using("snippets", function() {
createEditor();
});
}
else {
createEditor();
}
});
function createEditor() {
StackExchange.prepareEditor({
heartbeatType: 'answer',
autoActivateHeartbeat: false,
convertImagesToLinks: true,
noModals: true,
showLowRepImageUploadWarning: true,
reputationToPostImages: 10,
bindNavPrevention: true,
postfix: "",
imageUploader: {
brandingHtml: "Powered by u003ca class="icon-imgur-white" href="https://imgur.com/"u003eu003c/au003e",
contentPolicyHtml: "User contributions licensed under u003ca href="https://creativecommons.org/licenses/by-sa/3.0/"u003ecc by-sa 3.0 with attribution requiredu003c/au003e u003ca href="https://stackoverflow.com/legal/content-policy"u003e(content policy)u003c/au003e",
allowUrls: true
},
onDemand: true,
discardSelector: ".discard-answer"
,immediatelyShowMarkdownHelp:true
});
}
});
Sign up or log in
StackExchange.ready(function () {
StackExchange.helpers.onClickDraftSave('#login-link');
});
Sign up using Google
Sign up using Facebook
Sign up using Email and Password
Post as a guest
Required, but never shown
StackExchange.ready(
function () {
StackExchange.openid.initPostLogin('.new-post-login', 'https%3a%2f%2fstackoverflow.com%2fquestions%2f47493559%2fvalueerror-non-broadcastable-output-operand-with-shape-3-1-doesnt-match-the%23new-answer', 'question_page');
}
);
Post as a guest
Required, but never shown
2 Answers
2
active
oldest
votes
2 Answers
2
active
oldest
votes
active
oldest
votes
active
oldest
votes
Change self.synaptic_weights += adjustment
to
self.synaptic_weights = self.synaptic_weights + adjustment
self.synaptic_weights
must have a shape of (3,1) and adjustment
must have a shape of (3,4). While the shapes are broadcastable numpy must not like trying to assign the result with shape (3,4) to an array of shape (3,1)
a = np.ones((3,1))
b = np.random.randint(1,10, (3,4))
>>> a
array([[1],
[1],
[1]])
>>> b
array([[8, 2, 5, 7],
[2, 5, 4, 8],
[7, 7, 6, 6]])
>>> a + b
array([[9, 3, 6, 8],
[3, 6, 5, 9],
[8, 8, 7, 7]])
>>> b += a
>>> b
array([[9, 3, 6, 8],
[3, 6, 5, 9],
[8, 8, 7, 7]])
>>> a
array([[1],
[1],
[1]])
>>> a += b
Traceback (most recent call last):
File "<pyshell#24>", line 1, in <module>
a += b
ValueError: non-broadcastable output operand with shape (3,1) doesn't match the broadcast shape (3,4)
The same error occurs when using numpy.add and specifying a
as the output array
>>> np.add(a,b, out = a)
Traceback (most recent call last):
File "<pyshell#31>", line 1, in <module>
np.add(a,b, out = a)
ValueError: non-broadcastable output operand with shape (3,1) doesn't match the broadcast shape (3,4)
>>>
A new a
needs to be created
>>> a = a + b
>>> a
array([[10, 4, 7, 9],
[ 4, 7, 6, 10],
[ 9, 9, 8, 8]])
>>>
add a comment |
Change self.synaptic_weights += adjustment
to
self.synaptic_weights = self.synaptic_weights + adjustment
self.synaptic_weights
must have a shape of (3,1) and adjustment
must have a shape of (3,4). While the shapes are broadcastable numpy must not like trying to assign the result with shape (3,4) to an array of shape (3,1)
a = np.ones((3,1))
b = np.random.randint(1,10, (3,4))
>>> a
array([[1],
[1],
[1]])
>>> b
array([[8, 2, 5, 7],
[2, 5, 4, 8],
[7, 7, 6, 6]])
>>> a + b
array([[9, 3, 6, 8],
[3, 6, 5, 9],
[8, 8, 7, 7]])
>>> b += a
>>> b
array([[9, 3, 6, 8],
[3, 6, 5, 9],
[8, 8, 7, 7]])
>>> a
array([[1],
[1],
[1]])
>>> a += b
Traceback (most recent call last):
File "<pyshell#24>", line 1, in <module>
a += b
ValueError: non-broadcastable output operand with shape (3,1) doesn't match the broadcast shape (3,4)
The same error occurs when using numpy.add and specifying a
as the output array
>>> np.add(a,b, out = a)
Traceback (most recent call last):
File "<pyshell#31>", line 1, in <module>
np.add(a,b, out = a)
ValueError: non-broadcastable output operand with shape (3,1) doesn't match the broadcast shape (3,4)
>>>
A new a
needs to be created
>>> a = a + b
>>> a
array([[10, 4, 7, 9],
[ 4, 7, 6, 10],
[ 9, 9, 8, 8]])
>>>
add a comment |
Change self.synaptic_weights += adjustment
to
self.synaptic_weights = self.synaptic_weights + adjustment
self.synaptic_weights
must have a shape of (3,1) and adjustment
must have a shape of (3,4). While the shapes are broadcastable numpy must not like trying to assign the result with shape (3,4) to an array of shape (3,1)
a = np.ones((3,1))
b = np.random.randint(1,10, (3,4))
>>> a
array([[1],
[1],
[1]])
>>> b
array([[8, 2, 5, 7],
[2, 5, 4, 8],
[7, 7, 6, 6]])
>>> a + b
array([[9, 3, 6, 8],
[3, 6, 5, 9],
[8, 8, 7, 7]])
>>> b += a
>>> b
array([[9, 3, 6, 8],
[3, 6, 5, 9],
[8, 8, 7, 7]])
>>> a
array([[1],
[1],
[1]])
>>> a += b
Traceback (most recent call last):
File "<pyshell#24>", line 1, in <module>
a += b
ValueError: non-broadcastable output operand with shape (3,1) doesn't match the broadcast shape (3,4)
The same error occurs when using numpy.add and specifying a
as the output array
>>> np.add(a,b, out = a)
Traceback (most recent call last):
File "<pyshell#31>", line 1, in <module>
np.add(a,b, out = a)
ValueError: non-broadcastable output operand with shape (3,1) doesn't match the broadcast shape (3,4)
>>>
A new a
needs to be created
>>> a = a + b
>>> a
array([[10, 4, 7, 9],
[ 4, 7, 6, 10],
[ 9, 9, 8, 8]])
>>>
Change self.synaptic_weights += adjustment
to
self.synaptic_weights = self.synaptic_weights + adjustment
self.synaptic_weights
must have a shape of (3,1) and adjustment
must have a shape of (3,4). While the shapes are broadcastable numpy must not like trying to assign the result with shape (3,4) to an array of shape (3,1)
a = np.ones((3,1))
b = np.random.randint(1,10, (3,4))
>>> a
array([[1],
[1],
[1]])
>>> b
array([[8, 2, 5, 7],
[2, 5, 4, 8],
[7, 7, 6, 6]])
>>> a + b
array([[9, 3, 6, 8],
[3, 6, 5, 9],
[8, 8, 7, 7]])
>>> b += a
>>> b
array([[9, 3, 6, 8],
[3, 6, 5, 9],
[8, 8, 7, 7]])
>>> a
array([[1],
[1],
[1]])
>>> a += b
Traceback (most recent call last):
File "<pyshell#24>", line 1, in <module>
a += b
ValueError: non-broadcastable output operand with shape (3,1) doesn't match the broadcast shape (3,4)
The same error occurs when using numpy.add and specifying a
as the output array
>>> np.add(a,b, out = a)
Traceback (most recent call last):
File "<pyshell#31>", line 1, in <module>
np.add(a,b, out = a)
ValueError: non-broadcastable output operand with shape (3,1) doesn't match the broadcast shape (3,4)
>>>
A new a
needs to be created
>>> a = a + b
>>> a
array([[10, 4, 7, 9],
[ 4, 7, 6, 10],
[ 9, 9, 8, 8]])
>>>
edited Nov 26 '17 at 7:48
answered Nov 26 '17 at 7:28


wwiiwwii
11.1k31948
11.1k31948
add a comment |
add a comment |
Hopefully, by now you must have executed the code, but the problem between his code and your code is this line:
training_output = np.array([[0,1,1,0]]).T
While transposing don't forget to add 2 square brackets, I had the same problem for the same code, this worked for me.
Thanks
Welcome to Stack Overflow! Please take some time to format your answers before posting to ensure it is readable easily by everyone. You can use backsticks (`) to format inline code for example
– Ferdz
Jan 3 at 21:25
add a comment |
Hopefully, by now you must have executed the code, but the problem between his code and your code is this line:
training_output = np.array([[0,1,1,0]]).T
While transposing don't forget to add 2 square brackets, I had the same problem for the same code, this worked for me.
Thanks
Welcome to Stack Overflow! Please take some time to format your answers before posting to ensure it is readable easily by everyone. You can use backsticks (`) to format inline code for example
– Ferdz
Jan 3 at 21:25
add a comment |
Hopefully, by now you must have executed the code, but the problem between his code and your code is this line:
training_output = np.array([[0,1,1,0]]).T
While transposing don't forget to add 2 square brackets, I had the same problem for the same code, this worked for me.
Thanks
Hopefully, by now you must have executed the code, but the problem between his code and your code is this line:
training_output = np.array([[0,1,1,0]]).T
While transposing don't forget to add 2 square brackets, I had the same problem for the same code, this worked for me.
Thanks
edited Jan 4 at 6:10
Tobias Wilfert
82931022
82931022
answered Jan 3 at 20:23
user10864598user10864598
11
11
Welcome to Stack Overflow! Please take some time to format your answers before posting to ensure it is readable easily by everyone. You can use backsticks (`) to format inline code for example
– Ferdz
Jan 3 at 21:25
add a comment |
Welcome to Stack Overflow! Please take some time to format your answers before posting to ensure it is readable easily by everyone. You can use backsticks (`) to format inline code for example
– Ferdz
Jan 3 at 21:25
Welcome to Stack Overflow! Please take some time to format your answers before posting to ensure it is readable easily by everyone. You can use backsticks (`) to format inline code for example
– Ferdz
Jan 3 at 21:25
Welcome to Stack Overflow! Please take some time to format your answers before posting to ensure it is readable easily by everyone. You can use backsticks (`) to format inline code for example
– Ferdz
Jan 3 at 21:25
add a comment |
Thanks for contributing an answer to Stack Overflow!
- Please be sure to answer the question. Provide details and share your research!
But avoid …
- Asking for help, clarification, or responding to other answers.
- Making statements based on opinion; back them up with references or personal experience.
To learn more, see our tips on writing great answers.
Sign up or log in
StackExchange.ready(function () {
StackExchange.helpers.onClickDraftSave('#login-link');
});
Sign up using Google
Sign up using Facebook
Sign up using Email and Password
Post as a guest
Required, but never shown
StackExchange.ready(
function () {
StackExchange.openid.initPostLogin('.new-post-login', 'https%3a%2f%2fstackoverflow.com%2fquestions%2f47493559%2fvalueerror-non-broadcastable-output-operand-with-shape-3-1-doesnt-match-the%23new-answer', 'question_page');
}
);
Post as a guest
Required, but never shown
Sign up or log in
StackExchange.ready(function () {
StackExchange.helpers.onClickDraftSave('#login-link');
});
Sign up using Google
Sign up using Facebook
Sign up using Email and Password
Post as a guest
Required, but never shown
Sign up or log in
StackExchange.ready(function () {
StackExchange.helpers.onClickDraftSave('#login-link');
});
Sign up using Google
Sign up using Facebook
Sign up using Email and Password
Post as a guest
Required, but never shown
Sign up or log in
StackExchange.ready(function () {
StackExchange.helpers.onClickDraftSave('#login-link');
});
Sign up using Google
Sign up using Facebook
Sign up using Email and Password
Sign up using Google
Sign up using Facebook
Sign up using Email and Password
Post as a guest
Required, but never shown
Required, but never shown
Required, but never shown
Required, but never shown
Required, but never shown
Required, but never shown
Required, but never shown
Required, but never shown
Required, but never shown
KPcubn3tEmmXa 1MCkj3 Yr13o9Z43jN,OnN2 E5,dHGIkJ
Broadcasting
– wwii
Nov 26 '17 at 7:23