Is there a way to calculate an Covariate-Adjusted Pearon's Coefficient Correlation Value?
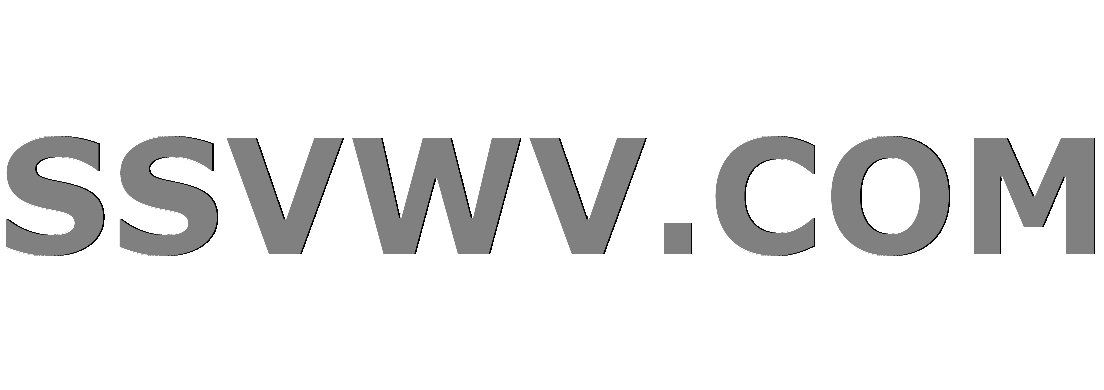
Multi tool use
.everyoneloves__top-leaderboard:empty,.everyoneloves__mid-leaderboard:empty,.everyoneloves__bot-mid-leaderboard:empty{ height:90px;width:728px;box-sizing:border-box;
}
Suppose I have data like this:
import pandas as pd
import statsmodels.formula.api as smf
sample_data = pd.read_csv('sample_data.csv',index_col = 0,header = 0)
sample_data
I want to explore the effects of Age on the Counts. If I want to remove the effect of sex from my data, I would perform a two step regression, using the residuals of my Counts vs. Sex and for a regression of residuals vs. Age like this:
sex_corrl = smf.ols('Counts ~ Sex',data = sample_data).fit()
sample_data['Residuals of Sex'] = list(sex_corrl.resid)
sex_adj_corrl = smf.ols('Q("Residuals of Sex") ~ Age',data = sample_data).fit()
sex_adj_corrl.summary()
My question is, if I take the square-root of the R2 value (the 0.013 value) at the end of the 2-step regression analysis, is it Pearson's Correlation Coefficient? If not, how would I calculate a sex-adjusted Pearson's Correlation Coefficient?
python linear-regression pearson-correlation
add a comment |
Suppose I have data like this:
import pandas as pd
import statsmodels.formula.api as smf
sample_data = pd.read_csv('sample_data.csv',index_col = 0,header = 0)
sample_data
I want to explore the effects of Age on the Counts. If I want to remove the effect of sex from my data, I would perform a two step regression, using the residuals of my Counts vs. Sex and for a regression of residuals vs. Age like this:
sex_corrl = smf.ols('Counts ~ Sex',data = sample_data).fit()
sample_data['Residuals of Sex'] = list(sex_corrl.resid)
sex_adj_corrl = smf.ols('Q("Residuals of Sex") ~ Age',data = sample_data).fit()
sex_adj_corrl.summary()
My question is, if I take the square-root of the R2 value (the 0.013 value) at the end of the 2-step regression analysis, is it Pearson's Correlation Coefficient? If not, how would I calculate a sex-adjusted Pearson's Correlation Coefficient?
python linear-regression pearson-correlation
add a comment |
Suppose I have data like this:
import pandas as pd
import statsmodels.formula.api as smf
sample_data = pd.read_csv('sample_data.csv',index_col = 0,header = 0)
sample_data
I want to explore the effects of Age on the Counts. If I want to remove the effect of sex from my data, I would perform a two step regression, using the residuals of my Counts vs. Sex and for a regression of residuals vs. Age like this:
sex_corrl = smf.ols('Counts ~ Sex',data = sample_data).fit()
sample_data['Residuals of Sex'] = list(sex_corrl.resid)
sex_adj_corrl = smf.ols('Q("Residuals of Sex") ~ Age',data = sample_data).fit()
sex_adj_corrl.summary()
My question is, if I take the square-root of the R2 value (the 0.013 value) at the end of the 2-step regression analysis, is it Pearson's Correlation Coefficient? If not, how would I calculate a sex-adjusted Pearson's Correlation Coefficient?
python linear-regression pearson-correlation
Suppose I have data like this:
import pandas as pd
import statsmodels.formula.api as smf
sample_data = pd.read_csv('sample_data.csv',index_col = 0,header = 0)
sample_data
I want to explore the effects of Age on the Counts. If I want to remove the effect of sex from my data, I would perform a two step regression, using the residuals of my Counts vs. Sex and for a regression of residuals vs. Age like this:
sex_corrl = smf.ols('Counts ~ Sex',data = sample_data).fit()
sample_data['Residuals of Sex'] = list(sex_corrl.resid)
sex_adj_corrl = smf.ols('Q("Residuals of Sex") ~ Age',data = sample_data).fit()
sex_adj_corrl.summary()
My question is, if I take the square-root of the R2 value (the 0.013 value) at the end of the 2-step regression analysis, is it Pearson's Correlation Coefficient? If not, how would I calculate a sex-adjusted Pearson's Correlation Coefficient?
python linear-regression pearson-correlation
python linear-regression pearson-correlation
asked Jan 3 at 21:47
superasiantomtom95superasiantomtom95
100110
100110
add a comment |
add a comment |
0
active
oldest
votes
Your Answer
StackExchange.ifUsing("editor", function () {
StackExchange.using("externalEditor", function () {
StackExchange.using("snippets", function () {
StackExchange.snippets.init();
});
});
}, "code-snippets");
StackExchange.ready(function() {
var channelOptions = {
tags: "".split(" "),
id: "1"
};
initTagRenderer("".split(" "), "".split(" "), channelOptions);
StackExchange.using("externalEditor", function() {
// Have to fire editor after snippets, if snippets enabled
if (StackExchange.settings.snippets.snippetsEnabled) {
StackExchange.using("snippets", function() {
createEditor();
});
}
else {
createEditor();
}
});
function createEditor() {
StackExchange.prepareEditor({
heartbeatType: 'answer',
autoActivateHeartbeat: false,
convertImagesToLinks: true,
noModals: true,
showLowRepImageUploadWarning: true,
reputationToPostImages: 10,
bindNavPrevention: true,
postfix: "",
imageUploader: {
brandingHtml: "Powered by u003ca class="icon-imgur-white" href="https://imgur.com/"u003eu003c/au003e",
contentPolicyHtml: "User contributions licensed under u003ca href="https://creativecommons.org/licenses/by-sa/3.0/"u003ecc by-sa 3.0 with attribution requiredu003c/au003e u003ca href="https://stackoverflow.com/legal/content-policy"u003e(content policy)u003c/au003e",
allowUrls: true
},
onDemand: true,
discardSelector: ".discard-answer"
,immediatelyShowMarkdownHelp:true
});
}
});
Sign up or log in
StackExchange.ready(function () {
StackExchange.helpers.onClickDraftSave('#login-link');
});
Sign up using Google
Sign up using Facebook
Sign up using Email and Password
Post as a guest
Required, but never shown
StackExchange.ready(
function () {
StackExchange.openid.initPostLogin('.new-post-login', 'https%3a%2f%2fstackoverflow.com%2fquestions%2f54030255%2fis-there-a-way-to-calculate-an-covariate-adjusted-pearons-coefficient-correlati%23new-answer', 'question_page');
}
);
Post as a guest
Required, but never shown
0
active
oldest
votes
0
active
oldest
votes
active
oldest
votes
active
oldest
votes
Thanks for contributing an answer to Stack Overflow!
- Please be sure to answer the question. Provide details and share your research!
But avoid …
- Asking for help, clarification, or responding to other answers.
- Making statements based on opinion; back them up with references or personal experience.
To learn more, see our tips on writing great answers.
Sign up or log in
StackExchange.ready(function () {
StackExchange.helpers.onClickDraftSave('#login-link');
});
Sign up using Google
Sign up using Facebook
Sign up using Email and Password
Post as a guest
Required, but never shown
StackExchange.ready(
function () {
StackExchange.openid.initPostLogin('.new-post-login', 'https%3a%2f%2fstackoverflow.com%2fquestions%2f54030255%2fis-there-a-way-to-calculate-an-covariate-adjusted-pearons-coefficient-correlati%23new-answer', 'question_page');
}
);
Post as a guest
Required, but never shown
Sign up or log in
StackExchange.ready(function () {
StackExchange.helpers.onClickDraftSave('#login-link');
});
Sign up using Google
Sign up using Facebook
Sign up using Email and Password
Post as a guest
Required, but never shown
Sign up or log in
StackExchange.ready(function () {
StackExchange.helpers.onClickDraftSave('#login-link');
});
Sign up using Google
Sign up using Facebook
Sign up using Email and Password
Post as a guest
Required, but never shown
Sign up or log in
StackExchange.ready(function () {
StackExchange.helpers.onClickDraftSave('#login-link');
});
Sign up using Google
Sign up using Facebook
Sign up using Email and Password
Sign up using Google
Sign up using Facebook
Sign up using Email and Password
Post as a guest
Required, but never shown
Required, but never shown
Required, but never shown
Required, but never shown
Required, but never shown
Required, but never shown
Required, but never shown
Required, but never shown
Required, but never shown
fdHjBhUfxV,juJLYXcMkroseKRdPr5 QhJgll f gjM,wm9ZkvOBLzgUG 29wX95ZtN,B7h,8W2a,8sA5yfsJ5C s6d