Calculate slope, length and angle of a specific part / side / line on a contour?
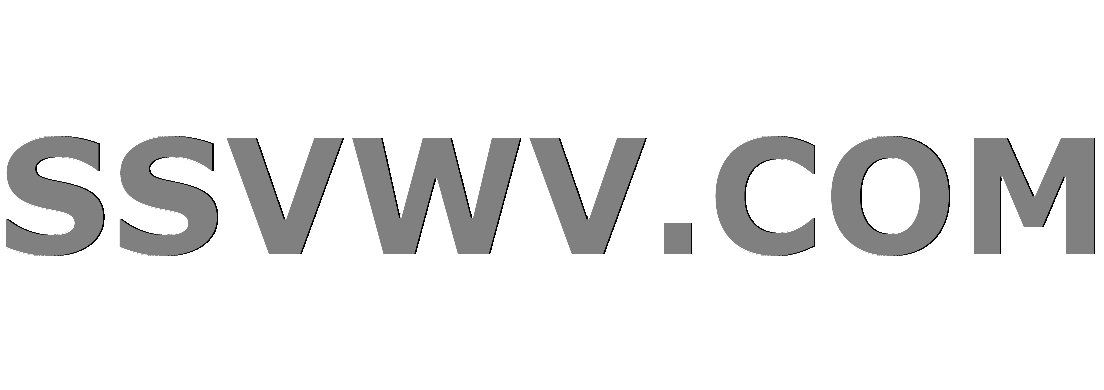
Multi tool use
.everyoneloves__top-leaderboard:empty,.everyoneloves__mid-leaderboard:empty,.everyoneloves__bot-mid-leaderboard:empty{ height:90px;width:728px;box-sizing:border-box;
}
I got two detected contours in an image and need the diameter between the two vertical-edges of the top contour and the diameter between the vertical-edges of the lower contour. I achieved this with this code.
import cv2
import numpy as np
import math, os
import imutils
img = cv2.imread("1.jpg")
gray = cv2.cvtColor(img, cv2.COLOR_RGB2GRAY)
gray = cv2.GaussianBlur(gray, (7, 7), 0)
edges = cv2.Canny(gray, 200, 100)
edges = cv2.dilate(edges, None, iterations=1)
edges = cv2.erode(edges, None, iterations=1)
cnts = cv2.findContours(edges.copy(), cv2.RETR_EXTERNAL, cv2.CHAIN_APPROX_SIMPLE)
cnts = imutils.grab_contours(cnts)
# sorting the contours to find the largest and smallest one
c1 = max(cnts, key=cv2.contourArea)
c2 = min(cnts, key=cv2.contourArea)
# determine the most extreme points along the contours
extLeft1 = tuple(c1[c1[:, :, 0].argmin()][0])
extRight1 = tuple(c1[c1[:, :, 0].argmax()][0])
extLeft2 = tuple(c2[c2[:, :, 0].argmin()][0])
extRight2 = tuple(c2[c2[:, :, 0].argmax()][0])
# show contour
cimg = cv2.drawContours(img, cnts, -1, (0,200,0), 2)
# set y of left point to y of right point
lst1 = list(extLeft1)
lst1[1] = extRight1[1]
extLeft1 = tuple(lst1)
lst2 = list(extLeft2)
lst2[1] = extRight2[1]
extLeft2= tuple(lst2)
# compute the distance between the points (x1, y1) and (x2, y2)
dist1 = math.sqrt( ((extLeft1[0]-extRight1[0])**2)+((extLeft1[1]-extRight1[1])**2) )
dist2 = math.sqrt( ((extLeft2[0]-extRight2[0])**2)+((extLeft2[1]-extRight2[1])**2) )
# draw lines
cv2.line(cimg, extLeft1, extRight1, (255,0,0), 1)
cv2.line(cimg, extLeft2, extRight2, (255,0,0), 1)
# draw the distance text
font = cv2.FONT_HERSHEY_SIMPLEX
fontScale = 0.5
fontColor = (255,0,0)
lineType = 1
cv2.putText(cimg,str(dist1),(155,100),font, fontScale, fontColor, lineType)
cv2.putText(cimg,str(dist2),(155,280),font, fontScale, fontColor, lineType)
# show image
cv2.imshow("Image", img)
cv2.waitKey(0)
Now I would also need the angle of the slope lines on the bottom side of the upper contour.
Any ideas how I can get this? Is it possible using contours?
Or is it necessary to use HoughLinesP and sort the regarding lines somehow?
And continued question: Maybe its also possible to get function which describes parabola slope of that sides ?
Thanks alot for any help!
python opencv image-processing angle
add a comment |
I got two detected contours in an image and need the diameter between the two vertical-edges of the top contour and the diameter between the vertical-edges of the lower contour. I achieved this with this code.
import cv2
import numpy as np
import math, os
import imutils
img = cv2.imread("1.jpg")
gray = cv2.cvtColor(img, cv2.COLOR_RGB2GRAY)
gray = cv2.GaussianBlur(gray, (7, 7), 0)
edges = cv2.Canny(gray, 200, 100)
edges = cv2.dilate(edges, None, iterations=1)
edges = cv2.erode(edges, None, iterations=1)
cnts = cv2.findContours(edges.copy(), cv2.RETR_EXTERNAL, cv2.CHAIN_APPROX_SIMPLE)
cnts = imutils.grab_contours(cnts)
# sorting the contours to find the largest and smallest one
c1 = max(cnts, key=cv2.contourArea)
c2 = min(cnts, key=cv2.contourArea)
# determine the most extreme points along the contours
extLeft1 = tuple(c1[c1[:, :, 0].argmin()][0])
extRight1 = tuple(c1[c1[:, :, 0].argmax()][0])
extLeft2 = tuple(c2[c2[:, :, 0].argmin()][0])
extRight2 = tuple(c2[c2[:, :, 0].argmax()][0])
# show contour
cimg = cv2.drawContours(img, cnts, -1, (0,200,0), 2)
# set y of left point to y of right point
lst1 = list(extLeft1)
lst1[1] = extRight1[1]
extLeft1 = tuple(lst1)
lst2 = list(extLeft2)
lst2[1] = extRight2[1]
extLeft2= tuple(lst2)
# compute the distance between the points (x1, y1) and (x2, y2)
dist1 = math.sqrt( ((extLeft1[0]-extRight1[0])**2)+((extLeft1[1]-extRight1[1])**2) )
dist2 = math.sqrt( ((extLeft2[0]-extRight2[0])**2)+((extLeft2[1]-extRight2[1])**2) )
# draw lines
cv2.line(cimg, extLeft1, extRight1, (255,0,0), 1)
cv2.line(cimg, extLeft2, extRight2, (255,0,0), 1)
# draw the distance text
font = cv2.FONT_HERSHEY_SIMPLEX
fontScale = 0.5
fontColor = (255,0,0)
lineType = 1
cv2.putText(cimg,str(dist1),(155,100),font, fontScale, fontColor, lineType)
cv2.putText(cimg,str(dist2),(155,280),font, fontScale, fontColor, lineType)
# show image
cv2.imshow("Image", img)
cv2.waitKey(0)
Now I would also need the angle of the slope lines on the bottom side of the upper contour.
Any ideas how I can get this? Is it possible using contours?
Or is it necessary to use HoughLinesP and sort the regarding lines somehow?
And continued question: Maybe its also possible to get function which describes parabola slope of that sides ?
Thanks alot for any help!
python opencv image-processing angle
add a comment |
I got two detected contours in an image and need the diameter between the two vertical-edges of the top contour and the diameter between the vertical-edges of the lower contour. I achieved this with this code.
import cv2
import numpy as np
import math, os
import imutils
img = cv2.imread("1.jpg")
gray = cv2.cvtColor(img, cv2.COLOR_RGB2GRAY)
gray = cv2.GaussianBlur(gray, (7, 7), 0)
edges = cv2.Canny(gray, 200, 100)
edges = cv2.dilate(edges, None, iterations=1)
edges = cv2.erode(edges, None, iterations=1)
cnts = cv2.findContours(edges.copy(), cv2.RETR_EXTERNAL, cv2.CHAIN_APPROX_SIMPLE)
cnts = imutils.grab_contours(cnts)
# sorting the contours to find the largest and smallest one
c1 = max(cnts, key=cv2.contourArea)
c2 = min(cnts, key=cv2.contourArea)
# determine the most extreme points along the contours
extLeft1 = tuple(c1[c1[:, :, 0].argmin()][0])
extRight1 = tuple(c1[c1[:, :, 0].argmax()][0])
extLeft2 = tuple(c2[c2[:, :, 0].argmin()][0])
extRight2 = tuple(c2[c2[:, :, 0].argmax()][0])
# show contour
cimg = cv2.drawContours(img, cnts, -1, (0,200,0), 2)
# set y of left point to y of right point
lst1 = list(extLeft1)
lst1[1] = extRight1[1]
extLeft1 = tuple(lst1)
lst2 = list(extLeft2)
lst2[1] = extRight2[1]
extLeft2= tuple(lst2)
# compute the distance between the points (x1, y1) and (x2, y2)
dist1 = math.sqrt( ((extLeft1[0]-extRight1[0])**2)+((extLeft1[1]-extRight1[1])**2) )
dist2 = math.sqrt( ((extLeft2[0]-extRight2[0])**2)+((extLeft2[1]-extRight2[1])**2) )
# draw lines
cv2.line(cimg, extLeft1, extRight1, (255,0,0), 1)
cv2.line(cimg, extLeft2, extRight2, (255,0,0), 1)
# draw the distance text
font = cv2.FONT_HERSHEY_SIMPLEX
fontScale = 0.5
fontColor = (255,0,0)
lineType = 1
cv2.putText(cimg,str(dist1),(155,100),font, fontScale, fontColor, lineType)
cv2.putText(cimg,str(dist2),(155,280),font, fontScale, fontColor, lineType)
# show image
cv2.imshow("Image", img)
cv2.waitKey(0)
Now I would also need the angle of the slope lines on the bottom side of the upper contour.
Any ideas how I can get this? Is it possible using contours?
Or is it necessary to use HoughLinesP and sort the regarding lines somehow?
And continued question: Maybe its also possible to get function which describes parabola slope of that sides ?
Thanks alot for any help!
python opencv image-processing angle
I got two detected contours in an image and need the diameter between the two vertical-edges of the top contour and the diameter between the vertical-edges of the lower contour. I achieved this with this code.
import cv2
import numpy as np
import math, os
import imutils
img = cv2.imread("1.jpg")
gray = cv2.cvtColor(img, cv2.COLOR_RGB2GRAY)
gray = cv2.GaussianBlur(gray, (7, 7), 0)
edges = cv2.Canny(gray, 200, 100)
edges = cv2.dilate(edges, None, iterations=1)
edges = cv2.erode(edges, None, iterations=1)
cnts = cv2.findContours(edges.copy(), cv2.RETR_EXTERNAL, cv2.CHAIN_APPROX_SIMPLE)
cnts = imutils.grab_contours(cnts)
# sorting the contours to find the largest and smallest one
c1 = max(cnts, key=cv2.contourArea)
c2 = min(cnts, key=cv2.contourArea)
# determine the most extreme points along the contours
extLeft1 = tuple(c1[c1[:, :, 0].argmin()][0])
extRight1 = tuple(c1[c1[:, :, 0].argmax()][0])
extLeft2 = tuple(c2[c2[:, :, 0].argmin()][0])
extRight2 = tuple(c2[c2[:, :, 0].argmax()][0])
# show contour
cimg = cv2.drawContours(img, cnts, -1, (0,200,0), 2)
# set y of left point to y of right point
lst1 = list(extLeft1)
lst1[1] = extRight1[1]
extLeft1 = tuple(lst1)
lst2 = list(extLeft2)
lst2[1] = extRight2[1]
extLeft2= tuple(lst2)
# compute the distance between the points (x1, y1) and (x2, y2)
dist1 = math.sqrt( ((extLeft1[0]-extRight1[0])**2)+((extLeft1[1]-extRight1[1])**2) )
dist2 = math.sqrt( ((extLeft2[0]-extRight2[0])**2)+((extLeft2[1]-extRight2[1])**2) )
# draw lines
cv2.line(cimg, extLeft1, extRight1, (255,0,0), 1)
cv2.line(cimg, extLeft2, extRight2, (255,0,0), 1)
# draw the distance text
font = cv2.FONT_HERSHEY_SIMPLEX
fontScale = 0.5
fontColor = (255,0,0)
lineType = 1
cv2.putText(cimg,str(dist1),(155,100),font, fontScale, fontColor, lineType)
cv2.putText(cimg,str(dist2),(155,280),font, fontScale, fontColor, lineType)
# show image
cv2.imshow("Image", img)
cv2.waitKey(0)
Now I would also need the angle of the slope lines on the bottom side of the upper contour.
Any ideas how I can get this? Is it possible using contours?
Or is it necessary to use HoughLinesP and sort the regarding lines somehow?
And continued question: Maybe its also possible to get function which describes parabola slope of that sides ?
Thanks alot for any help!
python opencv image-processing angle
python opencv image-processing angle
edited Jan 3 at 21:39


Patrick Artner
26.4k62544
26.4k62544
asked Jan 3 at 21:37
sonicdoosonicdoo
132
132
add a comment |
add a comment |
1 Answer
1
active
oldest
votes
There are several ways to obtain just the slopes. In order to know the slope, we can can use cv2.HoughLines
to detect the bottom horizontal line, detect to end points of that line and from those, obtain the slopes. As an illustration,
lines = cv2.HoughLines(edges, rho=1, theta=np.pi/180, threshold=int(dist2*0.66) )
on edges
in your code gives 4 lines, and if we force the angle to be horizontal
for line in lines:
rho, theta = line[0]
# here we filter out non-horizontal lines
if abs(theta - np.pi/2) > np.pi/180:
continue
a = np.cos(theta)
b = np.sin(theta)
x0 = a*rho
y0 = b*rho
x1 = int(x0 + 1000*(-b))
y1 = int(y0 + 1000*(a))
x2 = int(x0 - 1000*(-b))
y2 = int(y0 - 1000*(a))
cv2.line(img_lines,(x1,y1),(x2,y2),(0,0,255),1)
we get:
For the extended question concerns with the parabolas, we first compose a function that returns the left and right points:
def horizontal_scan(gray_img, thresh=50, start=50):
'''
scan horizontally for left and right points until we met an all-background line
@param thresh: threshold for background pixel
@param start: y coordinate to start scanning
'''
ret =
thickness = 0
for i in range(start,len(gray_img)):
row = gray_img[i]
# scan for left:
left = 0
while left < len(row) and row[left]<thresh:
left += 1
if left==len(row):
break;
# scan for right:
right = left
while right < len(row) and row[right] >= thresh:
right+=1
if thickness == 0:
thickness = right - left
# prevent sudden drop, error/noise
if (right-left) < thickness//5:
continue
else:
thickness = right - left
ret.append((i,left,right))
return ret
# we start scanning from extLeft1 down until we see a blank line
# with some tweaks, we can make horizontal_scan run on edges,
# which would be simpler and faster
horizontal_lines = horizontal_scan(gray, start = extLeft1[1])
# check if horizontal_line[0] are closed to extLeft1 and extRight1
print(horizontal_lines[0], extLeft1, extRight1[0])
Note that we can use this function to find the end points of the horizontal line returned by HoughLines.
# last line of horizontal_lines would be the points we need:
upper_lowest_y, upper_lowest_left, upper_lowest_right = horizontal_lines[-1]
img_lines = img.copy()
cv2.line(img_lines, (upper_lowest_left, upper_lowest_y), extLeft1, (0,0,255), 1)
cv2.line(img_lines, (upper_lowest_right, upper_lowest_y), extRight1, (0,0,255),1)
and that gives:
Let's return to the extended question, where we have those left and right points:
left_points = [(x,y) for y,x,_ in horizontal_lines]
right_points = [(x,y) for y,_,x in horizontal_lines]
Obviously, they would not fit perfectly in a parabola, so we need some sort of approximation/fitting here. For that, we can build a LinearRegression model:
from sklearn.linear_model import LinearRegression
class BestParabola:
def __init__(self, points):
x_x2 = np.array([(x**2,x) for x,_ in points])
ys = np.array([y for _,y in points])
self.lr = LinearRegression()
self.lr.fit(x_x2,ys)
self.a, self.b = self.lr.coef_
self.c = self.lr.intercept_
self.coef_ = (self.c,self.b,self.a)
def transform(self,points):
x_x2 = np.array([(x**2,x) for x,_ in points])
ys = self.lr.predict(x_x2)
return np.array([(x,y) for (_,x),y in zip(x_x2,ys)])
And then, we can fit the given left_points, right_points
to get the desired parabolas:
# construct the approximate parabola
# the parabollas' coefficients are accessible by BestParabola.coef_
left_parabola = BestParabola(left_points)
right_parabola = BestParabola(right_points)
# get points for rendering
left_parabola_points = left_parabola.transform(left_points)
right_parabola_points = right_parabola.transform(right_points)
# render with matplotlib, cv2.drawContours would work
plt.figure(figsize=(8,8))
plt.imshow(cv2.cvtColor(img,cv2.COLOR_BGR2RGB))
plt.plot(left_parabola_points[:,0], left_parabola_points[:,1], linewidth=3)
plt.plot(right_parabola_points[:,0], right_parabola_points[:,1], linewidth=3, color='r')
plt.show()
Which gives:
The left parabola is not perfect, but you should work out that if need be :-)
Thanks Quang Hoang for your perfect answer! Answered all my questions.<(^^)>
– sonicdoo
Jan 4 at 8:39
add a comment |
Your Answer
StackExchange.ifUsing("editor", function () {
StackExchange.using("externalEditor", function () {
StackExchange.using("snippets", function () {
StackExchange.snippets.init();
});
});
}, "code-snippets");
StackExchange.ready(function() {
var channelOptions = {
tags: "".split(" "),
id: "1"
};
initTagRenderer("".split(" "), "".split(" "), channelOptions);
StackExchange.using("externalEditor", function() {
// Have to fire editor after snippets, if snippets enabled
if (StackExchange.settings.snippets.snippetsEnabled) {
StackExchange.using("snippets", function() {
createEditor();
});
}
else {
createEditor();
}
});
function createEditor() {
StackExchange.prepareEditor({
heartbeatType: 'answer',
autoActivateHeartbeat: false,
convertImagesToLinks: true,
noModals: true,
showLowRepImageUploadWarning: true,
reputationToPostImages: 10,
bindNavPrevention: true,
postfix: "",
imageUploader: {
brandingHtml: "Powered by u003ca class="icon-imgur-white" href="https://imgur.com/"u003eu003c/au003e",
contentPolicyHtml: "User contributions licensed under u003ca href="https://creativecommons.org/licenses/by-sa/3.0/"u003ecc by-sa 3.0 with attribution requiredu003c/au003e u003ca href="https://stackoverflow.com/legal/content-policy"u003e(content policy)u003c/au003e",
allowUrls: true
},
onDemand: true,
discardSelector: ".discard-answer"
,immediatelyShowMarkdownHelp:true
});
}
});
Sign up or log in
StackExchange.ready(function () {
StackExchange.helpers.onClickDraftSave('#login-link');
});
Sign up using Google
Sign up using Facebook
Sign up using Email and Password
Post as a guest
Required, but never shown
StackExchange.ready(
function () {
StackExchange.openid.initPostLogin('.new-post-login', 'https%3a%2f%2fstackoverflow.com%2fquestions%2f54030149%2fcalculate-slope-length-and-angle-of-a-specific-part-side-line-on-a-contour%23new-answer', 'question_page');
}
);
Post as a guest
Required, but never shown
1 Answer
1
active
oldest
votes
1 Answer
1
active
oldest
votes
active
oldest
votes
active
oldest
votes
There are several ways to obtain just the slopes. In order to know the slope, we can can use cv2.HoughLines
to detect the bottom horizontal line, detect to end points of that line and from those, obtain the slopes. As an illustration,
lines = cv2.HoughLines(edges, rho=1, theta=np.pi/180, threshold=int(dist2*0.66) )
on edges
in your code gives 4 lines, and if we force the angle to be horizontal
for line in lines:
rho, theta = line[0]
# here we filter out non-horizontal lines
if abs(theta - np.pi/2) > np.pi/180:
continue
a = np.cos(theta)
b = np.sin(theta)
x0 = a*rho
y0 = b*rho
x1 = int(x0 + 1000*(-b))
y1 = int(y0 + 1000*(a))
x2 = int(x0 - 1000*(-b))
y2 = int(y0 - 1000*(a))
cv2.line(img_lines,(x1,y1),(x2,y2),(0,0,255),1)
we get:
For the extended question concerns with the parabolas, we first compose a function that returns the left and right points:
def horizontal_scan(gray_img, thresh=50, start=50):
'''
scan horizontally for left and right points until we met an all-background line
@param thresh: threshold for background pixel
@param start: y coordinate to start scanning
'''
ret =
thickness = 0
for i in range(start,len(gray_img)):
row = gray_img[i]
# scan for left:
left = 0
while left < len(row) and row[left]<thresh:
left += 1
if left==len(row):
break;
# scan for right:
right = left
while right < len(row) and row[right] >= thresh:
right+=1
if thickness == 0:
thickness = right - left
# prevent sudden drop, error/noise
if (right-left) < thickness//5:
continue
else:
thickness = right - left
ret.append((i,left,right))
return ret
# we start scanning from extLeft1 down until we see a blank line
# with some tweaks, we can make horizontal_scan run on edges,
# which would be simpler and faster
horizontal_lines = horizontal_scan(gray, start = extLeft1[1])
# check if horizontal_line[0] are closed to extLeft1 and extRight1
print(horizontal_lines[0], extLeft1, extRight1[0])
Note that we can use this function to find the end points of the horizontal line returned by HoughLines.
# last line of horizontal_lines would be the points we need:
upper_lowest_y, upper_lowest_left, upper_lowest_right = horizontal_lines[-1]
img_lines = img.copy()
cv2.line(img_lines, (upper_lowest_left, upper_lowest_y), extLeft1, (0,0,255), 1)
cv2.line(img_lines, (upper_lowest_right, upper_lowest_y), extRight1, (0,0,255),1)
and that gives:
Let's return to the extended question, where we have those left and right points:
left_points = [(x,y) for y,x,_ in horizontal_lines]
right_points = [(x,y) for y,_,x in horizontal_lines]
Obviously, they would not fit perfectly in a parabola, so we need some sort of approximation/fitting here. For that, we can build a LinearRegression model:
from sklearn.linear_model import LinearRegression
class BestParabola:
def __init__(self, points):
x_x2 = np.array([(x**2,x) for x,_ in points])
ys = np.array([y for _,y in points])
self.lr = LinearRegression()
self.lr.fit(x_x2,ys)
self.a, self.b = self.lr.coef_
self.c = self.lr.intercept_
self.coef_ = (self.c,self.b,self.a)
def transform(self,points):
x_x2 = np.array([(x**2,x) for x,_ in points])
ys = self.lr.predict(x_x2)
return np.array([(x,y) for (_,x),y in zip(x_x2,ys)])
And then, we can fit the given left_points, right_points
to get the desired parabolas:
# construct the approximate parabola
# the parabollas' coefficients are accessible by BestParabola.coef_
left_parabola = BestParabola(left_points)
right_parabola = BestParabola(right_points)
# get points for rendering
left_parabola_points = left_parabola.transform(left_points)
right_parabola_points = right_parabola.transform(right_points)
# render with matplotlib, cv2.drawContours would work
plt.figure(figsize=(8,8))
plt.imshow(cv2.cvtColor(img,cv2.COLOR_BGR2RGB))
plt.plot(left_parabola_points[:,0], left_parabola_points[:,1], linewidth=3)
plt.plot(right_parabola_points[:,0], right_parabola_points[:,1], linewidth=3, color='r')
plt.show()
Which gives:
The left parabola is not perfect, but you should work out that if need be :-)
Thanks Quang Hoang for your perfect answer! Answered all my questions.<(^^)>
– sonicdoo
Jan 4 at 8:39
add a comment |
There are several ways to obtain just the slopes. In order to know the slope, we can can use cv2.HoughLines
to detect the bottom horizontal line, detect to end points of that line and from those, obtain the slopes. As an illustration,
lines = cv2.HoughLines(edges, rho=1, theta=np.pi/180, threshold=int(dist2*0.66) )
on edges
in your code gives 4 lines, and if we force the angle to be horizontal
for line in lines:
rho, theta = line[0]
# here we filter out non-horizontal lines
if abs(theta - np.pi/2) > np.pi/180:
continue
a = np.cos(theta)
b = np.sin(theta)
x0 = a*rho
y0 = b*rho
x1 = int(x0 + 1000*(-b))
y1 = int(y0 + 1000*(a))
x2 = int(x0 - 1000*(-b))
y2 = int(y0 - 1000*(a))
cv2.line(img_lines,(x1,y1),(x2,y2),(0,0,255),1)
we get:
For the extended question concerns with the parabolas, we first compose a function that returns the left and right points:
def horizontal_scan(gray_img, thresh=50, start=50):
'''
scan horizontally for left and right points until we met an all-background line
@param thresh: threshold for background pixel
@param start: y coordinate to start scanning
'''
ret =
thickness = 0
for i in range(start,len(gray_img)):
row = gray_img[i]
# scan for left:
left = 0
while left < len(row) and row[left]<thresh:
left += 1
if left==len(row):
break;
# scan for right:
right = left
while right < len(row) and row[right] >= thresh:
right+=1
if thickness == 0:
thickness = right - left
# prevent sudden drop, error/noise
if (right-left) < thickness//5:
continue
else:
thickness = right - left
ret.append((i,left,right))
return ret
# we start scanning from extLeft1 down until we see a blank line
# with some tweaks, we can make horizontal_scan run on edges,
# which would be simpler and faster
horizontal_lines = horizontal_scan(gray, start = extLeft1[1])
# check if horizontal_line[0] are closed to extLeft1 and extRight1
print(horizontal_lines[0], extLeft1, extRight1[0])
Note that we can use this function to find the end points of the horizontal line returned by HoughLines.
# last line of horizontal_lines would be the points we need:
upper_lowest_y, upper_lowest_left, upper_lowest_right = horizontal_lines[-1]
img_lines = img.copy()
cv2.line(img_lines, (upper_lowest_left, upper_lowest_y), extLeft1, (0,0,255), 1)
cv2.line(img_lines, (upper_lowest_right, upper_lowest_y), extRight1, (0,0,255),1)
and that gives:
Let's return to the extended question, where we have those left and right points:
left_points = [(x,y) for y,x,_ in horizontal_lines]
right_points = [(x,y) for y,_,x in horizontal_lines]
Obviously, they would not fit perfectly in a parabola, so we need some sort of approximation/fitting here. For that, we can build a LinearRegression model:
from sklearn.linear_model import LinearRegression
class BestParabola:
def __init__(self, points):
x_x2 = np.array([(x**2,x) for x,_ in points])
ys = np.array([y for _,y in points])
self.lr = LinearRegression()
self.lr.fit(x_x2,ys)
self.a, self.b = self.lr.coef_
self.c = self.lr.intercept_
self.coef_ = (self.c,self.b,self.a)
def transform(self,points):
x_x2 = np.array([(x**2,x) for x,_ in points])
ys = self.lr.predict(x_x2)
return np.array([(x,y) for (_,x),y in zip(x_x2,ys)])
And then, we can fit the given left_points, right_points
to get the desired parabolas:
# construct the approximate parabola
# the parabollas' coefficients are accessible by BestParabola.coef_
left_parabola = BestParabola(left_points)
right_parabola = BestParabola(right_points)
# get points for rendering
left_parabola_points = left_parabola.transform(left_points)
right_parabola_points = right_parabola.transform(right_points)
# render with matplotlib, cv2.drawContours would work
plt.figure(figsize=(8,8))
plt.imshow(cv2.cvtColor(img,cv2.COLOR_BGR2RGB))
plt.plot(left_parabola_points[:,0], left_parabola_points[:,1], linewidth=3)
plt.plot(right_parabola_points[:,0], right_parabola_points[:,1], linewidth=3, color='r')
plt.show()
Which gives:
The left parabola is not perfect, but you should work out that if need be :-)
Thanks Quang Hoang for your perfect answer! Answered all my questions.<(^^)>
– sonicdoo
Jan 4 at 8:39
add a comment |
There are several ways to obtain just the slopes. In order to know the slope, we can can use cv2.HoughLines
to detect the bottom horizontal line, detect to end points of that line and from those, obtain the slopes. As an illustration,
lines = cv2.HoughLines(edges, rho=1, theta=np.pi/180, threshold=int(dist2*0.66) )
on edges
in your code gives 4 lines, and if we force the angle to be horizontal
for line in lines:
rho, theta = line[0]
# here we filter out non-horizontal lines
if abs(theta - np.pi/2) > np.pi/180:
continue
a = np.cos(theta)
b = np.sin(theta)
x0 = a*rho
y0 = b*rho
x1 = int(x0 + 1000*(-b))
y1 = int(y0 + 1000*(a))
x2 = int(x0 - 1000*(-b))
y2 = int(y0 - 1000*(a))
cv2.line(img_lines,(x1,y1),(x2,y2),(0,0,255),1)
we get:
For the extended question concerns with the parabolas, we first compose a function that returns the left and right points:
def horizontal_scan(gray_img, thresh=50, start=50):
'''
scan horizontally for left and right points until we met an all-background line
@param thresh: threshold for background pixel
@param start: y coordinate to start scanning
'''
ret =
thickness = 0
for i in range(start,len(gray_img)):
row = gray_img[i]
# scan for left:
left = 0
while left < len(row) and row[left]<thresh:
left += 1
if left==len(row):
break;
# scan for right:
right = left
while right < len(row) and row[right] >= thresh:
right+=1
if thickness == 0:
thickness = right - left
# prevent sudden drop, error/noise
if (right-left) < thickness//5:
continue
else:
thickness = right - left
ret.append((i,left,right))
return ret
# we start scanning from extLeft1 down until we see a blank line
# with some tweaks, we can make horizontal_scan run on edges,
# which would be simpler and faster
horizontal_lines = horizontal_scan(gray, start = extLeft1[1])
# check if horizontal_line[0] are closed to extLeft1 and extRight1
print(horizontal_lines[0], extLeft1, extRight1[0])
Note that we can use this function to find the end points of the horizontal line returned by HoughLines.
# last line of horizontal_lines would be the points we need:
upper_lowest_y, upper_lowest_left, upper_lowest_right = horizontal_lines[-1]
img_lines = img.copy()
cv2.line(img_lines, (upper_lowest_left, upper_lowest_y), extLeft1, (0,0,255), 1)
cv2.line(img_lines, (upper_lowest_right, upper_lowest_y), extRight1, (0,0,255),1)
and that gives:
Let's return to the extended question, where we have those left and right points:
left_points = [(x,y) for y,x,_ in horizontal_lines]
right_points = [(x,y) for y,_,x in horizontal_lines]
Obviously, they would not fit perfectly in a parabola, so we need some sort of approximation/fitting here. For that, we can build a LinearRegression model:
from sklearn.linear_model import LinearRegression
class BestParabola:
def __init__(self, points):
x_x2 = np.array([(x**2,x) for x,_ in points])
ys = np.array([y for _,y in points])
self.lr = LinearRegression()
self.lr.fit(x_x2,ys)
self.a, self.b = self.lr.coef_
self.c = self.lr.intercept_
self.coef_ = (self.c,self.b,self.a)
def transform(self,points):
x_x2 = np.array([(x**2,x) for x,_ in points])
ys = self.lr.predict(x_x2)
return np.array([(x,y) for (_,x),y in zip(x_x2,ys)])
And then, we can fit the given left_points, right_points
to get the desired parabolas:
# construct the approximate parabola
# the parabollas' coefficients are accessible by BestParabola.coef_
left_parabola = BestParabola(left_points)
right_parabola = BestParabola(right_points)
# get points for rendering
left_parabola_points = left_parabola.transform(left_points)
right_parabola_points = right_parabola.transform(right_points)
# render with matplotlib, cv2.drawContours would work
plt.figure(figsize=(8,8))
plt.imshow(cv2.cvtColor(img,cv2.COLOR_BGR2RGB))
plt.plot(left_parabola_points[:,0], left_parabola_points[:,1], linewidth=3)
plt.plot(right_parabola_points[:,0], right_parabola_points[:,1], linewidth=3, color='r')
plt.show()
Which gives:
The left parabola is not perfect, but you should work out that if need be :-)
There are several ways to obtain just the slopes. In order to know the slope, we can can use cv2.HoughLines
to detect the bottom horizontal line, detect to end points of that line and from those, obtain the slopes. As an illustration,
lines = cv2.HoughLines(edges, rho=1, theta=np.pi/180, threshold=int(dist2*0.66) )
on edges
in your code gives 4 lines, and if we force the angle to be horizontal
for line in lines:
rho, theta = line[0]
# here we filter out non-horizontal lines
if abs(theta - np.pi/2) > np.pi/180:
continue
a = np.cos(theta)
b = np.sin(theta)
x0 = a*rho
y0 = b*rho
x1 = int(x0 + 1000*(-b))
y1 = int(y0 + 1000*(a))
x2 = int(x0 - 1000*(-b))
y2 = int(y0 - 1000*(a))
cv2.line(img_lines,(x1,y1),(x2,y2),(0,0,255),1)
we get:
For the extended question concerns with the parabolas, we first compose a function that returns the left and right points:
def horizontal_scan(gray_img, thresh=50, start=50):
'''
scan horizontally for left and right points until we met an all-background line
@param thresh: threshold for background pixel
@param start: y coordinate to start scanning
'''
ret =
thickness = 0
for i in range(start,len(gray_img)):
row = gray_img[i]
# scan for left:
left = 0
while left < len(row) and row[left]<thresh:
left += 1
if left==len(row):
break;
# scan for right:
right = left
while right < len(row) and row[right] >= thresh:
right+=1
if thickness == 0:
thickness = right - left
# prevent sudden drop, error/noise
if (right-left) < thickness//5:
continue
else:
thickness = right - left
ret.append((i,left,right))
return ret
# we start scanning from extLeft1 down until we see a blank line
# with some tweaks, we can make horizontal_scan run on edges,
# which would be simpler and faster
horizontal_lines = horizontal_scan(gray, start = extLeft1[1])
# check if horizontal_line[0] are closed to extLeft1 and extRight1
print(horizontal_lines[0], extLeft1, extRight1[0])
Note that we can use this function to find the end points of the horizontal line returned by HoughLines.
# last line of horizontal_lines would be the points we need:
upper_lowest_y, upper_lowest_left, upper_lowest_right = horizontal_lines[-1]
img_lines = img.copy()
cv2.line(img_lines, (upper_lowest_left, upper_lowest_y), extLeft1, (0,0,255), 1)
cv2.line(img_lines, (upper_lowest_right, upper_lowest_y), extRight1, (0,0,255),1)
and that gives:
Let's return to the extended question, where we have those left and right points:
left_points = [(x,y) for y,x,_ in horizontal_lines]
right_points = [(x,y) for y,_,x in horizontal_lines]
Obviously, they would not fit perfectly in a parabola, so we need some sort of approximation/fitting here. For that, we can build a LinearRegression model:
from sklearn.linear_model import LinearRegression
class BestParabola:
def __init__(self, points):
x_x2 = np.array([(x**2,x) for x,_ in points])
ys = np.array([y for _,y in points])
self.lr = LinearRegression()
self.lr.fit(x_x2,ys)
self.a, self.b = self.lr.coef_
self.c = self.lr.intercept_
self.coef_ = (self.c,self.b,self.a)
def transform(self,points):
x_x2 = np.array([(x**2,x) for x,_ in points])
ys = self.lr.predict(x_x2)
return np.array([(x,y) for (_,x),y in zip(x_x2,ys)])
And then, we can fit the given left_points, right_points
to get the desired parabolas:
# construct the approximate parabola
# the parabollas' coefficients are accessible by BestParabola.coef_
left_parabola = BestParabola(left_points)
right_parabola = BestParabola(right_points)
# get points for rendering
left_parabola_points = left_parabola.transform(left_points)
right_parabola_points = right_parabola.transform(right_points)
# render with matplotlib, cv2.drawContours would work
plt.figure(figsize=(8,8))
plt.imshow(cv2.cvtColor(img,cv2.COLOR_BGR2RGB))
plt.plot(left_parabola_points[:,0], left_parabola_points[:,1], linewidth=3)
plt.plot(right_parabola_points[:,0], right_parabola_points[:,1], linewidth=3, color='r')
plt.show()
Which gives:
The left parabola is not perfect, but you should work out that if need be :-)
answered Jan 4 at 3:53


Quang HoangQuang Hoang
2,38711016
2,38711016
Thanks Quang Hoang for your perfect answer! Answered all my questions.<(^^)>
– sonicdoo
Jan 4 at 8:39
add a comment |
Thanks Quang Hoang for your perfect answer! Answered all my questions.<(^^)>
– sonicdoo
Jan 4 at 8:39
Thanks Quang Hoang for your perfect answer! Answered all my questions.<(^^)>
– sonicdoo
Jan 4 at 8:39
Thanks Quang Hoang for your perfect answer! Answered all my questions.<(^^)>
– sonicdoo
Jan 4 at 8:39
add a comment |
Thanks for contributing an answer to Stack Overflow!
- Please be sure to answer the question. Provide details and share your research!
But avoid …
- Asking for help, clarification, or responding to other answers.
- Making statements based on opinion; back them up with references or personal experience.
To learn more, see our tips on writing great answers.
Sign up or log in
StackExchange.ready(function () {
StackExchange.helpers.onClickDraftSave('#login-link');
});
Sign up using Google
Sign up using Facebook
Sign up using Email and Password
Post as a guest
Required, but never shown
StackExchange.ready(
function () {
StackExchange.openid.initPostLogin('.new-post-login', 'https%3a%2f%2fstackoverflow.com%2fquestions%2f54030149%2fcalculate-slope-length-and-angle-of-a-specific-part-side-line-on-a-contour%23new-answer', 'question_page');
}
);
Post as a guest
Required, but never shown
Sign up or log in
StackExchange.ready(function () {
StackExchange.helpers.onClickDraftSave('#login-link');
});
Sign up using Google
Sign up using Facebook
Sign up using Email and Password
Post as a guest
Required, but never shown
Sign up or log in
StackExchange.ready(function () {
StackExchange.helpers.onClickDraftSave('#login-link');
});
Sign up using Google
Sign up using Facebook
Sign up using Email and Password
Post as a guest
Required, but never shown
Sign up or log in
StackExchange.ready(function () {
StackExchange.helpers.onClickDraftSave('#login-link');
});
Sign up using Google
Sign up using Facebook
Sign up using Email and Password
Sign up using Google
Sign up using Facebook
Sign up using Email and Password
Post as a guest
Required, but never shown
Required, but never shown
Required, but never shown
Required, but never shown
Required, but never shown
Required, but never shown
Required, but never shown
Required, but never shown
Required, but never shown
VKr6Se9cUn1,p,JpWOepes,lqNxWXj,Kkd2F,E7j5w6 2,N,VsP6T4H17N,e,hfQI,lVh iGqoVKk30D0dbGTX